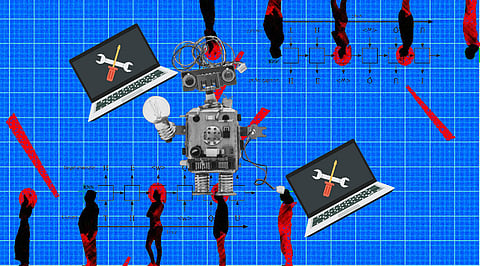
The term 'Large Language Models' or LLM is flourishing in the global tech market in recent times. Companies like OpenAI, Google, Meta, and many more are fully focused on introducing AI models in the form of a large language model to drive customer engagement towards their brands. Users using LLM and the global tech market are quite surprised with the smart features of these AI models from reputed tech companies. Meanwhile, scientists and other researchers have discovered some of the key flaws such as hardware problems of a large language model like GPT-3 that are not known to the general public. GPT-3, OPT, BERT, and many more AI models are gaining popularity for impressive discoveries in recent history in the field of artificial intelligence. Hardware problems pose some grave concerns in one of these large language models. Tech companies are not addressing the hardware problems while leveraging artificial intelligence, deep learning systems, and more. Let's explore how a large language model such as GPT-3 can have serious hardware problems in 2022 and beyond in the global tech market.
Some tech companies have started leveraging a popular large language model but are experiencing multiple hardware problems with LLM. It has been claimed that AI models such as GPT-3 are hard to run with these constant rare hardware problems. The global tech market is enjoying all the smart features of LLM while ignoring the back-end problems. It is getting difficult to train and run very large deep learning and AI models despite investing millions of dollars for training an LLM. Tech companies are facing difficulties in gaining expertise and distributed computing while dealing with hardware problems. It is quite rare in Industry 4.0 to have a specialization in distributed parallel computation and mend all necessary hardware problems.
One of the key hardware problems is seeking the right mode of distribution and hardware configuration because an LLM tends to grow bigger. There is no availability of a one-size-fits-all approach for all kinds of AI models and other hardware stacks. Some layers of AI models like GPT-3 and others can grow bigger to not fit on a single GPU. It is a constant barrier for tech companies because the tensor model parallel needs manual coding and configuration with expert knowledge. AI models like GPT-3, OPT, etc. are showing trial and error, failures, and continuous tweaking that are signals common while training a large language model on huge clusters of GPUs. Some studies showed the poor performance of large language models like GPT-3 and suffering from the same failures with hardware problems as present in deep learning systems. Poor performance includes plan generalization, replanning, optimal planning, and many more.
In order to solve these major hardware problems in an LLM, AI researchers and experts from different fields need to collaborate and work on an effective solution for tech companies across the world. One needs to contribute own specialization to create solutions efficiently and effectively for AI models.
Join our WhatsApp Channel to get the latest news, exclusives and videos on WhatsApp
_____________
Disclaimer: Analytics Insight does not provide financial advice or guidance. Also note that the cryptocurrencies mentioned/listed on the website could potentially be scams, i.e. designed to induce you to invest financial resources that may be lost forever and not be recoverable once investments are made. You are responsible for conducting your own research (DYOR) before making any investments. Read more here.