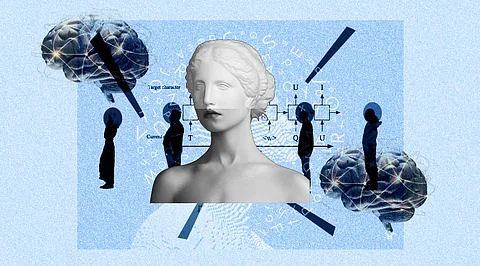
Generative Pre-Trained Transformer (GPT) is about to be improvised. Open AI, the non-profit research institute which is constantly striving to make AI human-like, or rather human brain into it has been working on its next version GPT-4, and will be soon released into the market. The earlier version of GPT-3 launched two years ago was designed in a neural network framework that applies machine learning to generate text. It only needs one simple cue to generate long and meaningful ML-generated text. There is lots of excitement around GPT-4 which has raised the stakes for superior performance. According to reports, the model is trained on 100 trillion machine learning parameters that may go beyond mere textual output. Apart from this fact, there is very little public info like what would be its specific features be like.
However, there have been many speculations doing rounds. Since GPT-4 is expected to have around 100 trillion parameters and will be five hundred times larger than GPT-3, it is giving room for some hyper-inflated expectations. Since the human brain has around 100 trillion synapses, GPT-4 too will have as many synapses generating hopes of having a human-like language generator in the market. This brings the old debate around the efficiency of large models again into the limelight. Big companies like Google and Facebook too, are no exception to falling into this trap. When OpenAI's Jared Kaplan and colleagues reported that scaling the number of parameters always results in improved performance, they took to its face value only to see smaller but efficient models beating out PaLM, and GSLM. In other cases, like MT-NLG, doesn't stand up to any benchmark when compared to smaller models like Gopher or Chinchilla.
The notion, that "larger is better" is gradually being abandoned by big companies, and making them look for alternative routes for better-performing machine learning models. Given the collateral damage they can inflict – environmental, financial, and discrimination – the number of parameters used has become the least effective factor to consider to estimate the efficiency of a model. OpnAI's CEO Sam Altman himself made it clear that they are not focussing on making models extremely larger anymore. GPT-4 though is touted to have trained on a meteoric number of parameters; the number is only slightly higher than that for GPT-3. GPT-4, perhaps is OpenAI's attempt to reach the optimum number of parameters for a language model and shift to other aspects such as data, algorithms, parameterization, or alignment to achieve significant improvements more cleanly. In a few shot settings, GPT-3 has proven to be the best at machine learning tasks, question answering, or doing fill-in-the-blanks, a strength GPT-3 inherits by virtue of its design. As Open AI researchers put it because few-shot results are better than zero-shot results, by increasing the number of parameters, the rate of increase in performance can be increased compared to the zero-shot model, making GPT-3 a meta learner and increasing the number of parameters will increase its meta-learning capabilities. The huge number of parameters signifies the possibility of having a large context window taking it only a bit closer to the human brain. Even this conclusion be derived only after trying the model thoroughly.