Data Analytics and Stock Trading: How to Use Data Science in Stock Market Analysis
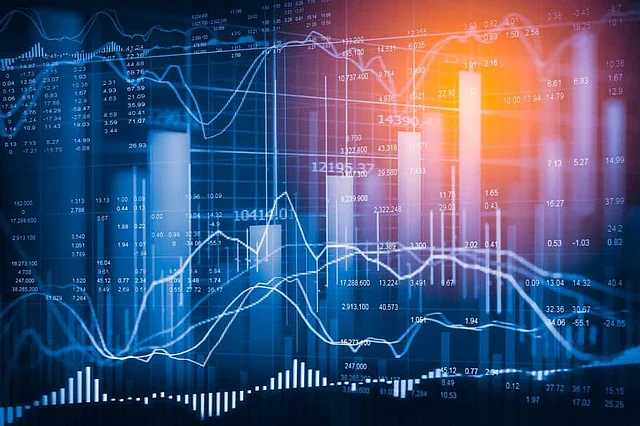
Everywhere you turn, you read about the power of data science. Everyone is concerned about data. Businesses are interested in understanding how data can help them trim expenses and improve their bottom line. The healthcare industry is interested in ways that data science can help them predict illnesses and provide better care to their patients.
Data science is typically represented in numbers. But these numbers could mean anything, ranging from the number of customers who purchase a product to the amount of inventory that is sold. Of course, these numbers could also represent cash.
It is in this way that data science is being used to provide a unique understanding of the stock market and financial data. Securities, commodities, and stocks follow some basic principles for trading. We can either sell, buy, or hold. The goal is to make the largest profit possible. The question that many are trying to answer is, what role does data science play in helping us make trades in the stock market?
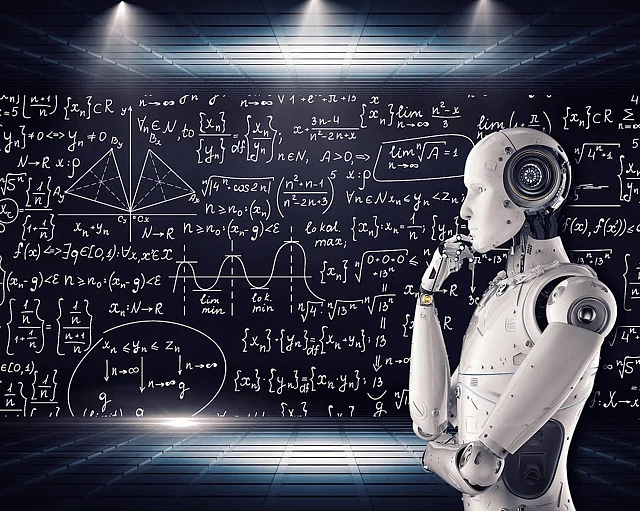
Trading platforms became very popular in the last two decades, but each platform offers different options, tools, fees, etc. Despite this growing trend, Canadians still haven't been able to access zero trading commission platforms. Gary Stevens from Hosting Canada conducted a 12-month research on how some of the most popular stock trading platforms work, and compared what each of them offers to its users. You need to understand how they work in order to pick what's best for you – and Gary's thorough guide is able to help you with that.
We also recommend this guide by The Balance if you're looking for more detailed information about Canadian ETFs.
Understanding Basic Data Science Concepts When Dealing with the Stock Market
There are a lot of phrases used in data science that a person would have to be a scientist to know. At its most basic level, data science is math that is sprinkled with an understanding of programming and statistics.
There are certain concepts in data science that are used when analyzing the market. In this context, we are using the term "analyze" to determine whether it is worth it to invest in a stock. There are some basic data science concepts that are good to be familiar with.
Algorithms
Algorithms are used extensively in data science. Basically, an algorithm is a group of rules needed to perform a task. You have likely heard about algorithms being used when buying and selling stocks. Algorithmic trading is where algorithms set rules for things like when to buy a stock or when to sell a stock.
For example, an algorithm could be set to purchase a stock once it drops by eight percent over the course of the day or to sell the stock if it loses 10 percent of its value compared to when it was first purchased. Algorithms are designed to function without human intervention. You may have heard of them referred to as bots. Like robots, they make calculated decisions devoid of emotions.
Training
We are not talking about preparing to run a 50 meter race. In machine learning and data science, training is where data is used to train a machine on how to respond. We can create a learning model. This machine learning model makes it possible for a computer to make accurate predictions based on the information it learned from the past. If you want to teach a machine to predict the future of stock prices, it would need a model of the stock prices of the previous year to use as a base to predict what will happen.
Testing
We have the data for stock prices for the last year. The training set would be the data from January to October. Then, we will use November and December as our testing set. Our machine should have learned by evaluating how the stocks worked from January through October. Now, we will ask it to predict what should have happened in November and December of that year. The predictions the machine makes will get compared to the real prices. The amount of variation that we see what the model predicts and the real data are what we are trying to eliminate as we adjust our training model.
The Role of Modeling to Predict Stock Prices
Data science relies heavily on modeling. This is an approach that uses math to examine past behaviors with the goal of forecasting future outcomes. In the stock market, a time series model is used.
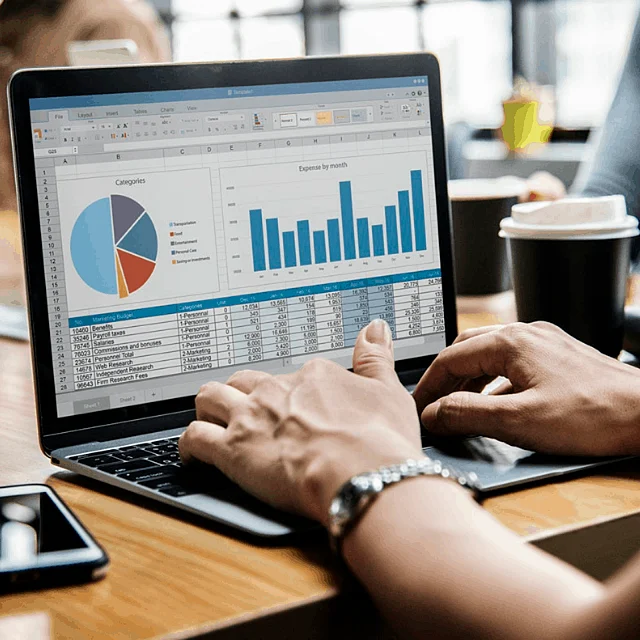
A time series is data, which in this case refers to the value of a stock, that is indexed over a period of time. This period of time could be divided hourly, daily, monthly, or even by the minute. A time series model is created by using machine learning and/or deep learning models to accumulate the price data. The data needs to be analyzed and then fitted to match the model. This is what makes it possible to predict future stock prices over a set timetable.
A second type of modeling that is used in machine learning and in data science is referred to as a classification model. These models are given data points and then they strive to classify or predict what is represented by those data points.
When discussing the stock market or stocks in general, a machine learning model can be given financial data like the P/E ratio, total debt, volume, etc. and then determine if a stock is a sound investment. Depending on the financials we give, a model can determine if now is the time to sell, hold, or buy a stock.
A model could predict something with so much complexity that it overlooks the relationship between the feature and the target variable. This is referred to as overfitting. Underfitting is where a model doesn't sufficiently match the data, so the results are predictions that are too simple.
Overfitting is a problem if the model finds it difficult to identify stock market trends, so it can't adapt to future events. Underfitting is where a model predicts the simple average price based on the stock's entire history. Both overfitting and underfitting lead to poor forecasts and predictions.
We have barely scratched the surface when discussing the link between machine learning concepts and stock market investments. However, it is important to understand the basic concepts we have discussed today as they serve as a basis for comprehending how machine learning is used to predict what the stock market can do. There are more concepts that can be learned by those who want to get to the nitty-gritty of data science and how it relates to the stock market.