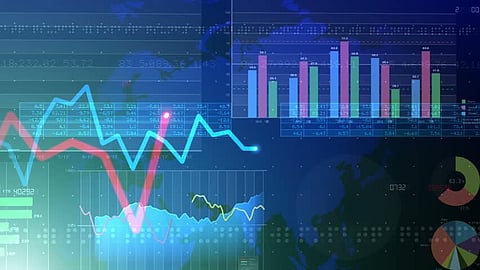
Data science typically deals with inferring data and creating related algorithms using various tools and techniques. This has the ability to add value to any business that can use their data effectively. Whether it is delivering assistance to businesses or executives, managing talent acquisition, workflows or making better decisions, data science holds vast promises to any company across any industry. Its applications range from healthcare, retail and personalized advertising to gaming and augmented reality, among others. In the banking sector, data science helps financial institutions to make smarter decisions to manage the challenges they face today.
As data analysis is the most crucial process in banking workflows, banks have realized the significance of big data technologies for their resource or asset management, and improve overall service delivery performance. In India, HDFC Bank, the country's second largest private sector lender, is the leading bank that introduced data analytics in the banking segment in early 2004 in the country. As data in the banking section is generated through multiple channels like call logs, emails, websites, social media and real-time market feeds, the use of data science is a valuable approach.
The face of the banking industry is continuously evolving, particularly post the financial crisis of 2008. Financial institutions are increasingly using machine learning to power their operations. As banks deal with a lot of customer data, analyzing and processing it draw a new set of risks and challenges. Thus, the use of data science in banking helps address fraud detection, customer predictions, risk modeling, personalized marketing, and customer support, among others.
Financial service providers have always been at the target of malicious actors or cybercriminals for data theft, ransomware attack, fraudulent and other malicious activities. In order to mitigate such vulnerabilities, banks are now turning to data science to detect deception and deliver customers with high level of security. It monitors and assesses users' banking activities to find out any suspicious or malicious patterns. By using advanced analytics software that provides necessary tools, banks can be able to identify and act on suspicious patterns, quickly notify customers of fraud incidents and place themselves for faster settlements. It also helps unveil patterns in bogus insurance claims.
As loan appraisals and lending is tied to the creditworthiness of the individuals or businesses that need loan, it requires to ease the big data problem. The more data banks have about an individual borrower, the better they can analyze their creditworthiness. In this way, financial institutions are using data science that is helping them in identifying individual's information at large and giving alerts to banks in the case of fraudulent.
With risk modeling, banks can devise new strategies to assess their performance. As Credit Risk Modeling is one of its most significant aspects, it enables financial institutions to scrutinize how their loan will be repaid. Significantly, this is vital because there is a chance that borrowers not being able to repay the loan. So, implementing Data Science, banking service providers can analyze and classify defaulters before sanctioning loan in a high-risk scenario. Moreover, risk modeling applies to the overall banking functions where analytical tools used to enumerate the performance of the banks as well as keep a track of their performance.
Today, almost every banking process has shifted to digital, making digital banking a prevalent and widely used. However, this creates voluminous amount of customer data, which needs to managed and processed in a secure manner. In this regard, the first and foremost step of a data scientist team in a bank is to segregate most germane data as not all the data they gather are useful and relevant. By doing so, they can foresee customer behaviors iteratively, interactions, and preferences. Implementing data science, financial institutions can also harness the potential of machine learning and predictive analytics to develop clustering tools that will help them identify various trends and patterns in managing customer data and fraud detection to secure that data.