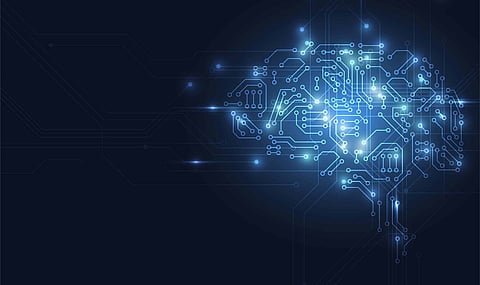
As artificial intelligence (AI) starts to develop—as an innovation as well as a scalable business practice, understanding why a few organizations are more effective than others at it frequently comes to realizing how to execute on goal-oriented plans.
Take data strategy, for instance—a key building block of AI adoption. By far most (85%) of the organizations creating AI today state they have an AI data strategy set up, yet the greater part likewise concede they don't understand enough about data infrastructure to deliver on AI initiatives.
Another study recommends why it's important to close the gap. Nearly 66% of companies viewed as top performers with AI state they have an unmistakable and steady data strategy. Only 20% of organizations are less effective with AI state the same.
What is clear is that AI has enormous potential. Regardless of tremendous levels of interest for AI advances, current implementations stay at very low levels. However, there is potential for solid development as CIOs start steering AI programs through a blend of buy, build and outsource efforts.
While it would be incredible if artificial intelligence was a straightforward module that could without much of a stretch be added to your business operations, similar to any activity that can change the manner in which you get things done, your business needs a strong foundation first.
Let's look at some important things to take into consideration to build an AI strategy.
Intelligent data strategy for AI begins with assessing "decision portfolio." That implies sitting with entrepreneurs and different chiefs and asking what choices they're currently making to use AI, the data they use to settle on those choices and the business value they hope to produce from those decisions.
"Pioneers of AI activities need to outline the key choices being made and adjust them to substantial worth, explicitly cost evasion, cost savings or net new revenue.
When those boundaries are defined, project leaders should then lead more of a technical audit to evaluate AI data infrastructure. At this stage, you take a look at the development of your whole data and AI scene. That implies discovering answers to a small bunch of key questions, for example, Have you gathered the correct data? Is it sorted out properly? Are there any models or dashboards worked around that data?
Joining these components helps lay the basic foundation for data strategy. You outline it up as decisions attached to real substantial worth. "What's more, you utilize both of those things, the decision portfolio and the technical evaluation to prioritize your decisions.
Artificial intelligence needs a robust and reliable technology infrastructure. Given AI's prominence, it is anything but difficult to overlook that it's not a self-contained technology. Without the help of well-working data and foundation, it is pointless. Deprived of the marketing hype, artificial intelligence is little more than an amalgamation of mathematical, statistical, and computer science techniques that depend intensely on a stable infrastructure and usable data.
A decent infrastructure considers the foundation of feedback loops, whereby victories and disappointments can be immediately hailed, analyzed, and acted upon.
Artificial intelligence in manufacturing is definitely not a brisk expansion to your business, and change management is an enormous aspect of the cycle. It starts with digitizing, a process that will associate workers with the digital value chain and empower them to turn out to be more engaged than ever before. To effectively execute a digital transformation and AI strategy, it is basic to draw on the knowledge of your inner group.
Advances in artificial intelligence are going on all the time and there is a requirement to consistently push ahead, which is the reason a working environment culture that is open to change is so significant. The energy you put into this change is similarly as significant as the advancements you implement to realise the vision.
Artificial intelligence can possibly offer new sources of revenue and profit, either through massive enhancements over the current method of getting things done or by empowering new processes that were not beforehand conceivable. In any case, incremental thinking about how AI can be utilized will undoubtedly lead to unobtrusive outcomes. Huge advantages are probably not going to be accomplished without another new business model mindset, or a so-called intelligence transformation.
Affectiva, which considers itself an emotion measurement organization, houses the world's biggest image database of sentiment-analyzed human faces. The organization investigates and groups a range of human feelings utilizing deep learning models that would then be able to be made available to customers. A few applications study emotional responses to ad campaigns, while others assist individuals with relearning emotional responses after a stroke. Affectiva has built a business model dependent on giving intelligence as a service in a field where nonhuman mediation was previously unrealistic.
These examples merely start to expose conceivable AI-empowered business models. We will soon have smart cameras that encourage franchising contracts and employee compensation schemes. Machine learning on granular information will take into consideration customization of products and services across time. As these and similar advancements open up new sources of income and benefits, new business models should in this manner be considered as a foundation of any AI strategy.
Join our WhatsApp Channel to get the latest news, exclusives and videos on WhatsApp
_____________
Disclaimer: Analytics Insight does not provide financial advice or guidance. Also note that the cryptocurrencies mentioned/listed on the website could potentially be scams, i.e. designed to induce you to invest financial resources that may be lost forever and not be recoverable once investments are made. You are responsible for conducting your own research (DYOR) before making any investments. Read more here.