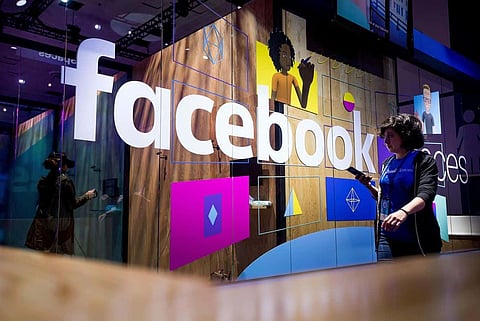
Notably, in the past few years, computer vision has expanded its reach and potential to a whole new level. Its applications and industry implementations encourage scientists and researchers to carry forward the legacy of advancement and experiment through new developments. In this regard, researchers from the University of Texas and Facebook AI Research investigated in a paper Ego-Topo, a technique that decomposes a space captured in a video into a topological map of activities before organizing the video into a series of visits to different zones.
As noted by Venture Beat, computer vision systems generally excel at detecting objects but struggle to make sense of the environments in which those objects are used. That's because they separate observed actions from physical context — even those that do model environments fail to discriminate between elements relevant to actions versus those that aren't (e.g., a cutting board on the counter versus a random patch of the floor).
Therefore, by reorganizing scenes into such "visits" as opposed to a series of footage, researchers assert, Ego-Topo is able to reason about first-person behavior (e.g., what are the most likely actions a person will do in the future?) and the environment itself (e.g., what are the possible object interactions that are likely in a particular zone, even if not observed there yet?).
According to a report, as noted by researchers, the first-person video naturally brings the use of a physical environment to the forefront, since it shows the camera wearer interacting fluidly in a space based on his intentions. However, current methods largely separate the observed actions from the persistent space itself. They have introduced a model for environmental affordances that are learned directly from the egocentric video. The main idea is to gain a human-centric model of physical space (such as a kitchen) that captures (1) the primary spatial zones of interaction and (2) the likely activities they support. Researchers' approach decomposes a space into a topological map derived from the first-person activity, organizing an ego-video into a series of visits to the different zones. Further, they show how to link zones across multiple related environments (e.g., from videos of multiple kitchens) to obtain a consolidated representation of environment functionality. On EPIC-Kitchens and EGTEA+, researchers demonstrated their approach for learning scene affordances and anticipating future actions in the long-form video.
They wrote, "our approach is best suited to long term activities in the [first-person] video where zones are repeatedly visited and used in multiple ways over time. This definition applies broadly to common household and workplace environments (e.g., office, kitchen, retail store, grocery). These tasks illustrate how a vision system that can successfully reason about scenes' functionality would contribute to applications in augmented reality (AR) and robotics. For example, an AR system that knows where actions are possible in the environment could interactively guide a person through a tutorial; a mobile robot able to learn from the video how people use a zone would be primed to act without extensive exploration."
As per their views, their experiments on scene affordance learning and long-range anticipation demonstrate its viability as enhanced representation of the environment gained from egocentric video. Future work can leverage the environment affordances to guide users in unfamiliar spaces with AR or allow robots to explore a new space through the lens of how it is likely used.
Join our WhatsApp Channel to get the latest news, exclusives and videos on WhatsApp
_____________
Disclaimer: Analytics Insight does not provide financial advice or guidance. Also note that the cryptocurrencies mentioned/listed on the website could potentially be scams, i.e. designed to induce you to invest financial resources that may be lost forever and not be recoverable once investments are made. You are responsible for conducting your own research (DYOR) before making any investments. Read more here.