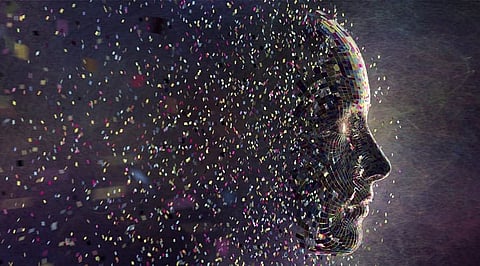
Recently researchers from Okinawa Institute of Science and Technology, used machine intelligence for mapping human brain
Since past couple of decade researchers are trying to make machines proficient in analyzing the data fed to them, understand it and make their own decision by mimicking human intelligence. Popularly known as artificial intelligence (AI), today enterprises are embedding AI technologies like machine learning and deep learning in solutions to make their organizational activities more efficient. However here's the problem: When we speak of AI does it differ from 'True AI', or does machine learning encompass all iterations of AI like deep learning, cognitive computing AI and others? This is why advocates of artificial intelligence are now contemplating if 'machine intelligence' will be an appropriate term to define intelligent capabilities shown by machinery systems.
Earlier 'intelligence', was extensively applied to humans and other living beings, only recently has this term been used for machines too. Machine intelligence aims to recreate human brain intelligence that is flexible and generalizes between different tasks, rather than focusing on a narrow set of specialized functions of many artificial intelligence subtypes. An article in Forbes explains machine intelligence is what's created when machines are programmed with some (but not all) aspects of human brain intelligence, including learning, problem solving and prioritization.
Machine intelligence algorithms can also help in better decision making. This is possible via machine learning – a latest tool that purports to improve this pre-emptive ability and make better decisions, through loops of self-learning. Technologies like cognitive computing, and some models of machine learning also work best when used in conjunction with humans. Meanwhile surveys have observed that people expect, high-level machine intelligence in future where machines will be capable of performing almost all tasks that are economically relevant today better than the median human (today) at each task – even asking subtle common-sense questions one can expect from a travel agent. Further, machine intelligence is also helping the scientific community develop new innovative techs that can augment abilities of existing technologies.
For instance, recently researchers from Okinawa Institute of Science and Technology (OIST) Graduate University, leveraged machine intelligence to improve the accuracy and reliability of a powerful brain-mapping technique. Their work published in Scientific Reports on December 18th, explains that researchers are more confidence in using the technique to untangle the human brain's wiring and to better understand the changes in this wiring that accompany neurological or mental disorders such as Parkinson's or Alzheimer's disease.
According to Professor Kenji Doya, who leads the Neural Computation Unit at the Okinawa Institute of Science and Technology Graduate University, 'connectome' of the brain is vital to fully understand the brain and all the complex processes it carries out before coming to a conclusion. To identify connectomes, researchers track nerve cell fibers that extend throughout the brain. In animal experiments, scientists can inject a fluorescent tracer into multiple points in the brain and image where the nerve fibers originating from these points extend to. Professor Kenji reveals that this process requires analyzing hundreds of brain slices from many animals. Since it is so invasive, it cannot be used in humans.
But latest advances in magnetic resonance imaging (MRI) have made it possible to estimate connectomes non-invasively. This technique, called diffusion MRI-based fiber tracking, uses powerful magnetic fields to track signals from water molecules as they move or diffuse along nerve fibers. Then, a computer algorithm uses these water signals to estimate the path of the nerve fibers throughout the whole brain, but at present, the algorithms do not produce convincing results.
Dr Carlos Gutierrez, first author and a postdoctoral researcher in the OIST Neural Computation Unit clarifies, "The connectomes can be dominated by false positives, meaning they show neural connections that aren't really there." Moreover, the algorithms struggle to detect nerve fibres that stretch between remote regions of the brain. Yet these long-distance connections are some of the most important for understanding how the brain functions.
So to offset these concerns, researchers devised an evolutionary algorithm based on machine intelligence. This new fiber tracking algorithm estimated the connectome from the diffusion MRI data using parameters that changed or mutated in each successive generation. Those parameters competed against each other and the best parameters, the ones that generated connectomes that most closely matched the neural network detected by the fluorescent tracer – advanced to the next generation.
The team tested the algorithms using fluorescent tracer and MRI data from ten different marmoset brains. They ran multiple iterations where algorithms running for each brain exchanged their best parameters with each other, allowing them to settle on a more similar set of parameters. At the end of the process, the researchers took the best parameters and averaged them to create one shared set.
In their experiment, they discovered that the algorithm with the generic set of optimized parameters also generated a more accurate connectome in new marmoset brains that weren't part of the original training set, compared to the default parameters which were used earlier. Additionally, the remarkable difference between the images constructed by algorithms using the default and optimized parameters sends out a stark warning about MRI-based connectome research. The team hopes to continue using machine intelligence to identify the best parameters faster and to use the improved algorithm to accurately determine the connectome of brains with neurological or mental disorders and treat them better.
Currently, machine intelligence offers a suite of technologies that can improve existing tasks across all industry verticals. Together artificial intelligence, machine learning, deep learning and others, can help to address environmental issues, improve health, mitigate cybercrimes and deliver better democracy and other pressing ones we face today. And in coming years, the abilities of these machine intelligence archetypes will expand to new spectrums.