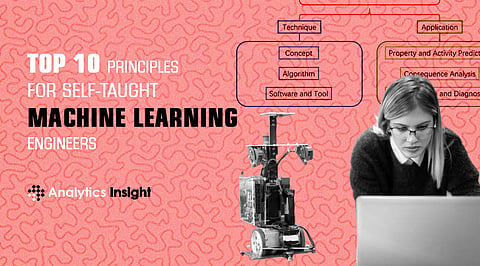
Being a self-taught machine learning engineer takes more than what is generally visible. Usually, people think about issues such as going to the right sources, scouting for good projects, and looking for the right opportunity to strike. But self-taught learners have challenges thrown at them which lie beyond the spectrum of the normal learning cycle. Here are the top 10 principles a self-taught machine learning engineer should follow.
A machine learning engineer works on building data pipelines, a task that is the culmination of quality data, ingenious math, and effective code. While data provides you with the building blocks math has all the tools hidden in itself, code will allow you to create a tool spinning machine.
Checking only for the above three factors and forgetting about the outcome is like laying a road towards an unknown destination. Therefore, instead of overthinking about developing a perfect model which has a 10% probability of working, it is advised to design a model that has an 80% of probability of working.
Data is Devine and math is pure. But you know at times, it takes just one data point to prove the theory otherwise. Therefore, be a skeptic at heart with math in mind.
Throughout the play, make sure your role is enacted to the point.
When machines do the learning part, you stick to the training part. Ensure your models do not fall short on the ethical dimension of their role.
Software and machine learning projects evolve constantly. As you progress in the learning process, you may have to recreate the project from scratch. There will be moments when u discover new data or more efficient code or optimizer which
Machine learning tools are available in plenty and the debate around them is endless. What you need is — focus. Understand which tool allows you to get the fastest result most reliably. And let the problem at hand decide the tool, not the other way round.
Build ideas and share them with others who would benefit from them. When no one is sure which idea may work, the only way is to let the ideas flow and seek feedback.
You are not the only star in the galaxy. How you take others' success and achievements only reveals your attitude towards your capability.
Choose machine learning projects which suit your interests and never do it in a rush. Go for the ones which enhance your learning curve and satiate creative urges.
Gratitude pays in ways you do not understand. If only you understand what fight they have put up with, for machine learning to develop to this level, you would learn more than what you aim for. Just remember, your work too will contribute to defining the future of machine learning.
Join our WhatsApp Channel to get the latest news, exclusives and videos on WhatsApp
_____________
Disclaimer: Analytics Insight does not provide financial advice or guidance. Also note that the cryptocurrencies mentioned/listed on the website could potentially be scams, i.e. designed to induce you to invest financial resources that may be lost forever and not be recoverable once investments are made. You are responsible for conducting your own research (DYOR) before making any investments. Read more here.