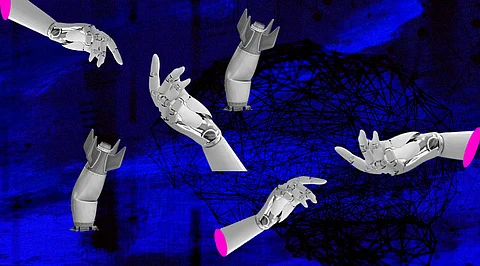
While the frequency of machine learning application development projects is clearly increasing, the number of project failures is nearly equalling it. In many respects, the growing failure rate of machine learning initiatives is discouraging new enterprises with excellent AI concepts from applying them. The goal of today's essay is to familiarize you with the many causes of machine learning project failure. Here are the reasons why ML projects fail:
If the graph teaches you anything, it's that a tremendous quantity of data is required for the success of an ML project. Businesses must have access to clean data in order to run a successful machine learning project. Clean data is data that is relevant, usable, devoid of errors, and easily available.
Aside from having clean, organized data, it is also required that the data be easily accessible for both small jobs and large-scale training in a single location: a data lake, a data warehouse, or some data platform.
Organizations prefer to incorporate models meant to stimulate innovation on the guidance of data scientists without considering their alignment with their current 'non-digital' culture and system components. So, while such solutions perform well in the industry, there is little to no rate of success in terms of ease of acceptance when they are integrated with the existing system.
The answers lie in bringing together all the teams that will concentrate on the ML project and those who will administer the legacy system. Following that, a milestone-based project deployment should be designed to help in smooth acceptance with a friction-free migration.
The sector is suffering from a severe scarcity of data scientists. Although there are many engineers who complete courses and label themselves as data scientists, the number of those who are actually trained to see through a complicated ML project is quite restricted. According to the 2020 State of Enterprise Machine Learning study, while the need for Machine Learning specialists is always increasing, there is a severe scarcity of qualified candidates to fill the position.
ML projects tend to become outdated over time and struggle to remain the best solution to the business issue. Typically, this occurs as a result of:
Updating an existing ML model is typically challenging and time-consuming: in many respects, it is akin to starting a new data science project. Despite the limitations, organizations often continue to use the approach long after the results have ceased.
Leaders may lack the commitment and technical confidence required to complete a project. While they support the initiative because of its fame, they pay less attention to assuring data accessibility, accuracy, funding and labor needs, and so on.
To ensure the success of an ML project, it is critical to keep everybody on board, particularly the board members, since even the slightest doubt in them may lead to a lot of nervousness among the teams, which assures project failure even before it begins.
Top 10 Ethereum NFT Projects You Should Know About in 2022
Netflix and Meta Stocks May Fall Further in Q2: Is it Sell Time?
Are Aliens Utilizing AI to Hide Themselves from Humans?
Why Hybrid Intelligence is The Future of Artificial Intelligence?
Know About Transformer Machine Learning Model at a Glance
Why Python Context Manager is Critical in Every Coding Project