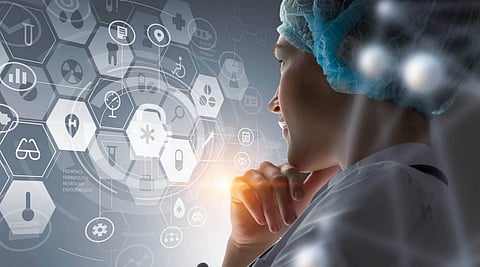
In a bid to offer the best of healthcare solutions, all the major segments of the healthcare industry from healthcare IT, payers, providers, and pharmaceutical companies are under increased pressure to improve the quality of patient care and offer the best of healthcare services at a lower cost.
Each of these organizations is accessing and finding value in an ever-growing pool of patient data. The diversity of this data which includes the EMR notes, medical correspondence, the output from health wearables, biomedical research, claims data, mobile data, and social media conversations imply that these are generated from multiple siloed data sources. In the healthcare industry, about 80 percent of the healthcare data is unstructured, which makes it tough for organizations to access and integrate with other data sources. The ability to securely integrate this wealth of data and apply predictive analytics would increase the efficiency of care, reduce fraudulent claims, discover more efficacious therapies, and improve physician enablement.
The MapR Distribution with Hadoop brings together the high volume of structured and unstructured healthcare data into a central repository which can deploy the existing hardware and network components. Multiple groups in healthcare organizations can access and store this data within a secure HIPAA-compliant Hadoop-enabled architecture. Doctors and caregivers have access to comprehensive patient data and medical research, which helps them to diagnose diseases at their early stages thus assigning therapies based on a patient's genetic makeup and adjusting the drug doses to minimize side effects to improve medical care.
Healthcare industry works on Electronic health records (EHR) a very unstructured document which poses a unique challenge to healthcare organizations as many EHRs allow free text input for clinical notes and other narrative data collection fields. This data is required to be extracted, processed, and normalized for analysis. Hadoop's distributed approach to data may be able to help. Hadoop works to store and analyse the data using mainly Hadoop Distributed Fie System (HDFS) and MapReduce.
Healthcare organizations continue to seek more effective ways to treat patients which can be achieved by collecting and analysing as much data as possible. Organizations collecting data on both patients and employees can more easily see where improvements need to be made and where ineffective efforts can be reduced. Hadoop has helped healthcare organisations in a multi-faced way in a number of applications. We have discussed a few examples and use cases on how Hadoop can help in healthcare.
Payers need to be able to detect fraud based on analysis of anomalies in billing data, procedural benchmark data or patient records. Payers can analyse data to detect anomalies like a hospital's overutilization of services in short time periods, patients receiving healthcare services from different hospitals at the same time, or identical prescriptions for the same patient filled in multiple locations. MapR uses anomaly detection to detect these incidents in real-time and alert providers to investigate them before payment is made.
Healthcare providers want to provide more proactive care for their patients by constantly monitoring patient vital signs. The data from these monitors can be used in real-time to alert care providers about changes in a patient's condition. MapR can help collect this data and stream it in real-time, which can help in detecting changes. Improved algorithms that run against larger sets of data can improve the likelihood of knowing when a particular patient might have an emergency, which helps providers plan for effective interventions.
Personalized treatment helps in offering customised health care solutions to users. The medication or dosage can be changed based on how the medication is working. This analysis can be tailored to each patient's specific needs. MapR provides real-time access, at both the summary and detailed level, so treatment decisions can be adjusted in a timely manner.
Clinical researchers can access broad knowledge pools across multiple data sources to aid in the accuracy of diagnosing patient conditions. Bringing together individual datasets into a big data repository and applying algorithms for predictive modelling provides more accurate insights by identifying nuances in subpopulations. These nuances may be so rare that they are not seen in small research samples, but with the ability to apply algorithms to these individual data sets, nuances can now be clearly detectable.
Healthcare of the past was plagued by data infrastructures incapable of handling the volume, velocity, and variety of data needed to derive deep clinical, financial, and operational insights of the industry.
Traditionally, data has been the result of independent business processes, which invariably led to data silos. This delayed critical patient data and forced it to be reactive if spotted and reported at all. Meaningful data would sit in an overnight batch queue waiting to be loaded into the enterprise data warehouse (EDW) where key analytical applications could offer intelligent insights.
Join our WhatsApp Channel to get the latest news, exclusives and videos on WhatsApp
_____________
Disclaimer: Analytics Insight does not provide financial advice or guidance. Also note that the cryptocurrencies mentioned/listed on the website could potentially be scams, i.e. designed to induce you to invest financial resources that may be lost forever and not be recoverable once investments are made. You are responsible for conducting your own research (DYOR) before making any investments. Read more here.