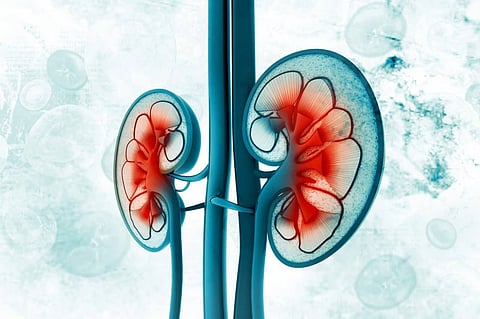
Contemporary medicinal science vigorously depends on the utilization of technology. Some portion of this innovation endeavors to improve examination and measurement of the human body, with the absolute most amazing technical breakthroughs to be found in the advancement of non-intrusive methodology. Another part focuses around the development of gadgets that help treatments for explicit pathologies. An excellent example of this is the artificial kidney, which has turned into the target of intensive research from numerous directions and produces incredible desires for dialysis patients. Research on the artificial kidney is as yet nascent however, and there are numerous challenges that must be solved before it will end up being a reality and part of clinical practice in nephrology. One of these non-unimportant difficulties concerns the wellbeing of clients of these new dialysis gadgets. Safety risks make effective monitoring frameworks obligatory.
Kidney disease influences roughly 30 million Americans. Around 650,000 of these people – including almost 15,000 Virginians, experience the ill effects of end-stage renal malady, requiring prolonged dialysis, the mechanical purging of blood. The University of Virginia thinks about more than 900 of these patients at its 11 dialysis clinics around the state.
According to Dr. Brendan Bowman, a nephrologist and director of UVA's dialysis framework, dialysis patients suffer high hospitalization rates, high healthcare services costs and higher mortality than numerous patients with unending sickness. "We might want to improve treatment outcomes while additionally cutting expenses for this treatment," said Dr. Brendan Bowman.
To do this, Bowman and different doctors are working with UVA frameworks data scientists and engineers on creating predictive systems to improve drug dosing conventions. The research is subsidized by UVA's Engineering in Medicine program, which unites medical clinicians and engineers to make better outcome results also by Virginia's Center for Innovative Technology.
A result of the abundance of technological gadgets encompassing the patient at the point of care is the persistent generation of information in an electronic arrangement, which, therefore, creates the requirement for analytical techniques equipped for changing such data into knowledge that is at last valuable for patients' treatment. The field of artificial intelligence (AI) is as of now giving probably the most encouraging outcomes as analytical tools.
Kidney disease can happen for numerous reasons, yet generally it is the consequence of strain put on the organ by other chronic ailments, for example, diabetes and high blood pressure. After some time, the kidney's proficiency at expelling impurities from the blood can decrease to the point where a patient requires dialysis three times each week. This normally includes visiting a clinic, being associated with a dialysis machine, and having the blood, every last bit of it, cycled through a channel, washed down of toxins and pollutants, and returned back to the body. Patients with end-stage renal illness frequently should do this forever, or, if lucky, until experiencing a kidney transplant.
Patients with end-stage renal illness generally experience the ill effects of anemia, which can cause shortness of breath, exhaustion, disquietude and dizziness, among different sufferings. Clinicians treat anemia with costly injectable meds, erythropoietin boss among them, to animate red platelet generation, a task regularly handled by a sound kidney.
The study's initial discoveries, a nine-month pilot project, recommend that the UVA dialysis framework could improve the treatment of low red blood cells, while at the same time lessening pointless utilization of a costly anemia treatment medicine by 25%. This could result in a better quality of life for dialysis patients and potential savings to the UVA Health System of $750,000 to $1 million every year.
How much prescription to utilize, however, isn't one-size-fits-all; it is as much workmanship as science. Every patient is unique, some requiring more drug than others, and clinicians should tweak their dosing throughout months, continually changing in accordance to locate that "sweet spot" in the cycle where the patient gets simply enough prescription to return red platelet checks to near normal, however less than the patient encounters destructive symptoms, or to be inefficient of costly medications.
As per Brown, this is fundamentally a control issue, which is an old-style engineering. We can utilize historical data to educate how we settle on decisions presently to influence future conditions. The objective is to build up a framework that can help clinicians more precisely control and foresee their patient's red blood cell count dependent on what we gather from huge data samples from an earlier time.
Bowman, it turns out, has reams of information, dosing records for somewhere in the range of 3,000 patients over a decade. Filtering through those treatment records to discover perfect dosing levels under a wide scope of conditions is an imposing task, requiring refined information analytics systems.