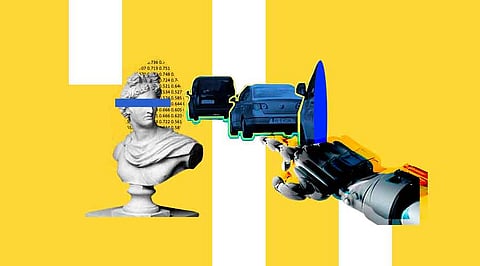
In Machine Learning (ML) and Artificial Intelligence (AI), data is the bedrock upon which success is built. However, not all data is created equal. Raw data, often chaotic, requires a transfer data formational process to make it usable for training AI and ML models. Data annotation, a meticulous and intricate task, is pivotal in converting raw data into valuable information that drives accurate predictions and insights.
Data annotation is fundamental for supervised learning, where models are trained on labeled data to make accurate predictions. By annotating data with labels, such as class categories or sentiment scores, data annotation equips ML algorithms to recognize patterns and make informed decisions.
Data annotation establishes ground truths in fields like image recognition and natural language processing. For instance, annotating images with bounding boxes or text entities creates a reference dataset, allowing models to learn and generalize from the provided examples.
Accurate data annotation leads to higher model performance. Models trained on precisely labeled data exhibit improved accuracy and reduced error rates, ultimately enhancing the quality of AI-driven predictions and outcomes.
Data annotation extends beyond simple labeling. It supports intricate tasks like semantic segmentation, where objects within images are precisely outlined or named entity recognition, identifying specific entities within the text. These annotations enable models to grasp nuances and context, yielding richer insights.
Data annotation is domain-specific and adaptable to various industries. From healthcare to autonomous driving, annotating data helps models understand domain-specific attributes, making them more adept at addressing unique challenges.
In cases of limited data availability, data annotation bridges the gap. Techniques like data augmentation create synthetic data points by perturbing existing annotations, enriching the training dataset, and improving model generalization.
Human annotation is invaluable for certain tasks. Through Human-in-the-Loop AI, human annotators continuously review and refine machine-generated annotations, ensuring accuracy and maintaining the model's relevance.
Data annotation extends to multimodal learning, where models process information from various sources like text, images, and audio. Annotation facilitates the convergence of these modalities, enabling holistic insights.
Data annotation allows for the identification and mitigation of bias in AI models. By ensuring diverse and balanced annotations, models become fairer, more inclusive, and aligned with ethical considerations.
Data annotation is a cycle, not a one-time endeavor. As models evolve, new data annotations refine their understanding. This continuous learning process ensures AI systems adapt to changing circumstances and remain relevant.
In the intricate landscape of ML and AI, data annotation is the transformative force that bridges the gap between raw data and actionable insights. By converting unstructured information into labeled, structured data, annotation empowers models to learn, predict, and evolve. As data annotation techniques evolve and intertwine with AI advances, the journey to smarter, more capable AI and ML systems accelerates, propelling industries into a future brimming with innovation and discovery.