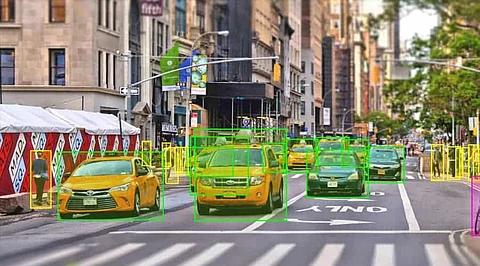
There is so much buzz about artificial intelligence (AI) and machine learning today. It is no longer surprising to realize that most of the tools you use online, from your smartphones, most websites, and various devices, use AI-powered machine learning to enhance your interaction with multiple applications. Some machine learning applications include facial recognition, speech recognition, financial security, bus schedules, traffic prediction, medical services, social media, customer support, and retail. Moreover, writing tools such as Spell Check are developed using machine learning.
Another excellent use of machine learning applications is predictive analytics. Suppose you log into your social media account,the system analyzes your interaction with the social media app. It can make intelligent choices about your friends and what type of sponsored content it can send through your news feed.
When a company is training a machine model for a specific application, it will use massive volumes of data. For example, an automotive company is developing a self-driving car. The machine learning model will need all the information to enable the autonomous vehicle to recognize all the objects and situations it will encounter in the real world.
A data annotation service provider will use all available data that they can feed to the machine. The information they provide to the device is not the usual data that programmers encode in machine learning. They will use various types of data, including photographs, videos, and text. Therefore, the annotators add labels to the elements in an image, photo, or video to enable the machine to recognize the information. They must ensure that all the labels or annotations are accurate. The work is tedious, and attention to detail is critical. The annotators must likewise ensure that the spelling of the labels is correct so that the machine model will learn adequately.
Humans perform the job of annotating the data. They have the skills and training to classify and decipher data that the machines can understand. For example, an image or photograph may have several objects such as humans, animals, and others. The annotator will need to label every recognizable and partially hidden item in the image. The labels are generic—man for a male person, woman for a female person, and it goes on, adding tags to each tree, every lamppost, cat, dog, and so on.
Given the example above, you can understand the volume of data they must handle and annotate to train a machine learning model. But because of the sheer volume of data they must annotate, there will be instances that they can make label and spelling errors. Thus, concentration and using tools like a spell checker can help.
Humans can perform various things and can exercise subjectivity. They can manage, understand intent, and face challenges and uncertainty. However, even if you input everything into an automated system, it does not have a human's insight. Therefore, there should be precision in the data tags so that the machines can learn the various objects precisely and develop the ability to provide accurate, on-target predictions and responses.
Humans better accomplish data annotation because they can annotate each data type carefully and precisely. They think critically while they work, manually check the spelling and placement of tags, and validate everything they do.