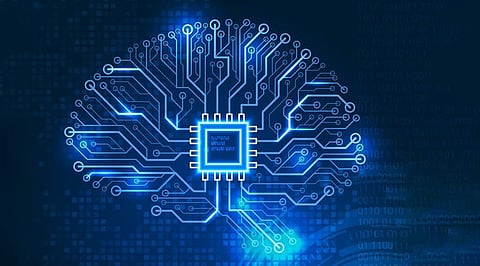
A decade ago, machine learning, particularly deep neural networks, played a significant role in the development of commercial AI applications. Deep neural networks were effectively executed in the mid 2010s on account of the computational capacity of modern computing hardware. Artificial intelligence hardware is another generation of hardware custom-built for AI applications.
As artificial intelligence and its applications become more far and wide, the competition to create less expensive and faster chips are probably going to grow among tech goliaths. Organizations can either rent these hardware on the cloud from cloud providers like Amazon AWS' Sagemaker service or purchase their hardware. Own hardware can bring about lower costs if usage can be kept high. If not, organizations are in an ideal situation depending on the cloud sellers.
Deep neural network- fueled solutions make up most commercial AI applications. The number and significance of these applications have been growing emphatically since the 2010s and are relied upon to continue developing at a similar speed. For instance, McKinsey predicts AI applications to create $4-6 trillion of significant value yearly.
Another recent Mckinsey research expresses that AI-related semiconductors will see around 18% development every year throughout the next few years. This is multiple times more than the development of semiconductors utilized in non-AI applications. A similar study expresses that AI hardware will be assessed to turn into a $67 billion market in income.
Working along with a global team, researchers at the University of Münster are growing new methodologies and process architectures that can adapt to these tasks very proficiently. They have now indicated that so-called photonic processors, with which data is processed by methods for light, can process data substantially more quickly and in equal — something electronic chips are unequipped for doing. The outcomes have been published in the journal Nature.
The group of analysts driven by Prof. Wolfram Pernice from the Institute of Physics and the Center for Soft Nanoscience at the University of Münster executed hardware accelerator for so-called matrix multiplications, which alludes to the fundamental processing load in the computation of neural networks. Neural networks are a progression of algorithms which reproduce the human mind. This is useful, for instance, for classifying objects in pictures and for speech recognition.
In the study, the physicists utilized a so-called convolutional neural network for the acknowledgment of handwritten numbers. These organizations are an idea in the field of machine learning roused by biological cycles. They are utilized fundamentally in the processing of image or audio data, as they at present accomplish the most noteworthy precision of classification.
The analysts consolidated the photonic structures with phase-change materials (PCMs) as energy-effective storage components. PCMs are typically utilized with DVDs or BluRay discs in optical data storage. In the new processor, this makes it conceivable to store and protect the matrix components without the requirement for an energy supply. To complete matrix multiplications on multiple data sets in parallel, the Münster physicists utilized a chip-based frequency comb as a light source.
Rather than conventional gadgets, which normally work in the low GHz range, optical modulation speeds can be accomplished with speeds up to the 50 to 100 GHz range." This implies that the process grants information rates and computing densities, for example operations per area of processor, never previously achieved.
The machine learning accelerators bring advantages over utilizing universally purpose hardware
Faster computation: Artificial intelligence applications ordinarily require equal computational abilities to run modern training models and algorithms. Artificial intelligence hardware gives more parallel processing capability that is assessed to have up to multiple times all the more competing power in ANN applications compared to customary semiconductor devices at comparative price points.
High bandwidth memory: Specialized AI hardware is assessed to dispense 4-5 times more bandwidth than customary chips. This is fundamental in light of the fact that because of the requirement for parallel processing, AI applications require altogether more bandwidth between processors for efficient performance.
Join our WhatsApp Channel to get the latest news, exclusives and videos on WhatsApp
_____________
Disclaimer: Analytics Insight does not provide financial advice or guidance. Also note that the cryptocurrencies mentioned/listed on the website could potentially be scams, i.e. designed to induce you to invest financial resources that may be lost forever and not be recoverable once investments are made. You are responsible for conducting your own research (DYOR) before making any investments. Read more here.