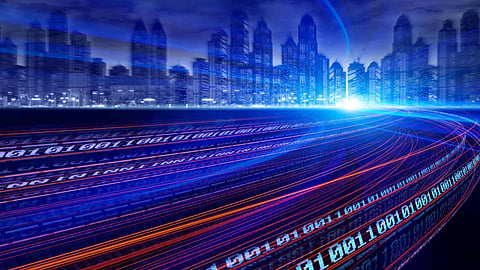
Today, the world is producing a mammoth amount of data every day. This voluminous data is persistently multiplying at a staggering rate. However, this is overwhelming businesses as they seek to collect and derive value from the real-time data, also known as streaming data. In general, streaming data is the incessant flow of data produced by various numbers of sources. Capitalizing on streaming data is a very complex process as most Big data architectures and the cloud is not able to handle this amount of data. By using effective stream processing technology, streaming data can be processed, stored, assessed, and acted upon as it is generated in real-time.
Previously, data processing was much easier as legacy infrastructures were much more structured and only had a handful of sources that generated data. So, the entire system could be architected in a way that could stipulate and unify the data and data structures. Conversely, data in modern days come from an endless number of sources. Every step of our browsing websites, exploring the Internet, using sensors, mobile devices, and various tools produce a massive volume of data. Thus, with legacy infrastructures, handling and regulating them is impossible.
Digital data streams provide companies with the ability to create new products and services, improve their value to existing customers, and optimize internal operations. A majority of businesses use these data streams to enhance their market positions and gain an advantage over competitors.
Using applications for data streams require two main functions, storage and processing. Storage must have the capacity to store an enormous amount of data streams sequentially and consistently, whereas processing should require to interact with storage, and assess and run a computation on the data.
Interpreting data to derive meaningful information often relies on organizations' ability to identify potential data streams based on feasibility and streamability. This enables firms to analyze the viability of harnessing a given class of events or creating a data stream that does not previously exist. Once businesses recognize the potential data streams, they then require to evaluate how much value they can excerpt from the initiative.
Streaming data plays an essential role in the world of big data, delivering real-time analyses, data integration, and data ingestion. Making use of advanced data stream systems that combine data from distinct sources and make it available to decision-makers enables companies to develop strong business intelligence and analytics capabilities. Without having the right architecture in place, organizational data lakes quickly become data swamps. In this way, they must analyze business challenges to get to their correct data streaming architecture, as well as they must close the blurring lines between modern and existing technologies.
Moreover, garnering real-time insights from large-scale data depends in part on developing a system around a streaming architecture. To build such systems, organizations often look for fast stream processing systems like Apache Spark Streaming, with its in-memory near real-time capability through micro-batching, or truly real-time streaming tools such as Apache Storm.
Join our WhatsApp Channel to get the latest news, exclusives and videos on WhatsApp
_____________
Disclaimer: Analytics Insight does not provide financial advice or guidance. Also note that the cryptocurrencies mentioned/listed on the website could potentially be scams, i.e. designed to induce you to invest financial resources that may be lost forever and not be recoverable once investments are made. You are responsible for conducting your own research (DYOR) before making any investments. Read more here.