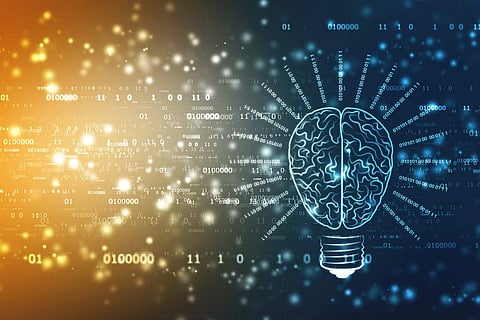
Most data advancement projects come up short since companies disregard fundamental steps that should drive the project. It's important to understand that fundamentals are as significant as the science behind the development.
To become completely data-powered, it is about learning the essential steps and phases of a data analytics project and tailing them from raw information preparation to building an AI model, and eventually, to operationalization.
Understanding the business or action that your data project is part of is vital to assure its prosperity and the first phase of any sound data analytics project. To spur the various on-screen characters important to get your project from design to production, your project must be the answer to an unmistakable authoritative need.
Before even considering the data, go out and converse with the individuals in your company whose processes or whose business you expect to improve with data. At that point, plunk down to characterize a course of events and concrete key performance indicators. You might find planning and procedures to be exhausting, however, ultimately, they are a fundamental initial step to kickstart your data initiative.
Having great data is a method of creating accountability in a company. Responsible employees guarantee due to constancy in capturing and storing data created from the everyday operations of the business. Frequently, companies wind up in a fix where existing data isn't usable. Helpless data quality leads to wrong insights and at last wrong choices.
With low quality data, data projects will undoubtedly be valueless. There is nothing so lethal than settling on wrong decisions because of wrong information. This thus can influence a broad scope of things ranging from the business focus, brand representation to product development.
Data audits should eventually guarantee all information required to settle on decisions is accessible in an analyzable format.
The best organizations develop a culture that urges utilizing data to solve issues, which goes way beyond just the analytics team. Questions should be welcomed from all areas of the company. Companies should set an unequivocal expectation that employees use data in better approaches to respond to new inquiries. One approach to do this is to characterize intellectual curiosity as a basic criterion for advancement.
When you're managing huge volumes of data, visualization is the most ideal approach to explore and impart your discoveries. The precarious part here is to have the option to delve into your graphs whenever and answer any question somebody would have about a given insight. That is the point at which the data preparation proves to be useful: you're the person who did all the filthy work, so you realize the data is like the palm of your hand!
If this is the last step of your project, it's critical to utilize APIs and plugins so you can push those insights to where your end-users need to have them. Graphs are additionally another approach to advance your dataset and grow all the more fascinating features. For instance, by putting your data points on a map you could maybe see that particular geographic zones are more telling than explicit nations or cities.
With an increasingly complex data set, remember that your users can rapidly get overwhelmed
regardless of whether you comprehend the visualization totally. Since the visual-processing centers in our minds are so solid, it is simple for us to feel totally great with a complex visualization since we have been submerged in the information. New users, however, battle without that foundation.
The most important stage in executing a data analytics project is the modelling stage. The stage is totally subject to having great indicators, quality data and well mapped success factors. Achievement factors thus become variables. Prescient models allot a probability score to a result utilizing a set of predictor variables.
The fundamental objective in any business project is to demonstrate its adequacy as quick as conceivable to legitimize, well, your job. The same goes for data projects. By picking up time on data cleaning and enriching, you can go to the furthest limit of the project quickly and get your underlying outcomes. This is the final phase of finishing your data analytics project and one that is critical to the whole data life cycle.
Probably the greatest misstep that individuals make as to machine learning is believing that once a model is built and goes live, it will keep filling in as ordinary indefinitely. Despite what might be expected, models will really degrade in quality after some time if they're not ceaselessly improved and taken care of new data.
Unexpectedly, so as to effectively finish your first data project, you have to understand that your model will never be completely "finished." In order for it to stay helpful and accurate, you have to continually reevaluate, retrain it, and develop new features. If there's anything you detract from these fundamental steps in analytics and data science, it is that a data analyst's job is never really done, however that is the thing that makes working with data all the more intriguing!
Join our WhatsApp Channel to get the latest news, exclusives and videos on WhatsApp
_____________
Disclaimer: Analytics Insight does not provide financial advice or guidance. Also note that the cryptocurrencies mentioned/listed on the website could potentially be scams, i.e. designed to induce you to invest financial resources that may be lost forever and not be recoverable once investments are made. You are responsible for conducting your own research (DYOR) before making any investments. Read more here.