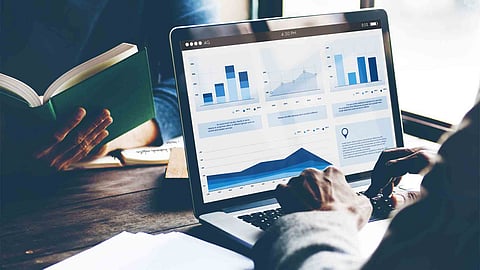
The increasing amounts of big data that modern enterprises possess make the case of data management even more vital than ever before. Efficient data management collaborating with Data Pipelines and Data Catalogues lets enterprises gain the maximum from the disruptive technologies, artificial intelligence in particular. Data catalogues are increasingly gaining prominence for all the data stakeholders, especially analyst, developer, and data scientists who are looking for available data assets to garner the maximum business intelligence.
As several companies haven't attained the ultimate nirvana, the high level of sophistication with crucial data-related aspects, posing a threat to the current data practices.
Is cleaning data really that difficult?
Research suggests, yes!! Cleaning crude and inaccurate data before feeding it into an AI model is a cumbersome process.
The Holistic Data Preparation Process-
To add to data management woes, Data Governance is fast gaining prominence as a tough problem spot.
As a result, it is easy to fall prey to pitfalls such as inadvertently using or revealing sensitive information hidden among anonymized data. For example, while a patient's name might be encrypted from one section of a medical record that is used by an AI system, it could be present in the doctor's notes section of the record. Such data governance and data responsibility conditions must be addressed since it is critical for the C-suite to be aware of as they work to stay in line with privacy rules, such as the California Consumer Privacy Act (CCPA), or the European Union's General Data Protection Regulation (GDPR) and otherwise manage reputation risk.
If these various data management and governance issues are not addressed early on, deeper issues could emerge later to fracture AI initiatives. AI technology providers can play a role in supporting businesses to navigate shortcomings related to data practices by:
These steps can enable the adopter organizations to develop a holistic data-based AI strategy that scales with and adapts to their changing needs and demands.
Join our WhatsApp Channel to get the latest news, exclusives and videos on WhatsApp
_____________
Disclaimer: Analytics Insight does not provide financial advice or guidance. Also note that the cryptocurrencies mentioned/listed on the website could potentially be scams, i.e. designed to induce you to invest financial resources that may be lost forever and not be recoverable once investments are made. You are responsible for conducting your own research (DYOR) before making any investments. Read more here.