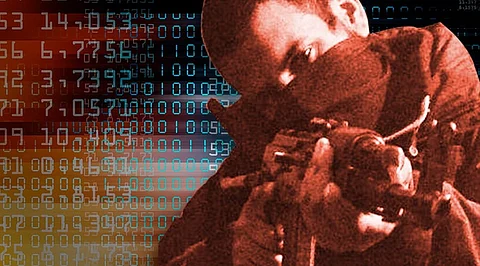
India has a history of terrorist attacks. Since the 70s, more than 12k terrorist incidents have taken place (according to a report) that includes the 1993 Bombay Bombing, the 2008 Mumbai attacks, and the recent 2021 Sukma-Bijapur attack. Researchers have been researching to better understand the mechanisms behind terrorism in the hope of preventing future potentially devastating acts of terror. Regardless of the huge endeavors contributed to study terrorism, the quantitative examination has principally evolved and applied methodologies targeting portraying provincial instances of terrorist acts without giving dependable and exact momentary expectations at the local level needed by policymakers to carry out designated intercessions. So the question is can machine learning predict future terrorism?
Distributing in science advances, a global exploration group drove by Dr. Andre Python from the Center of Data Science at Zhejiang University explore machine learning algorithms capable of predicting and explaining at fine spatiotemporal scale the occurrence of terrorism executed by non-state entertainers outside authentic fighting (non-state illegal intimidation) across the world. To cover all locales overall possibly influenced by terrorism throughout a huge period. An interpretable tree-based AI calculation is contrasted with elective benchmark prescient models to anticipate and clarify the likelihood of the occurrence of terrorism (reaction) in every week cell across the world. To anticipate complex social wonders like terrorism at fine spatiotemporal scales, hypothetically educated AI calculations are probably going to beat stingy models utilizing procedural provisions only. The decision of the provisions remembered for the prescient model is critical; the importance of the model yields and the prescient exhibition advantage from a strong calculated comprehension of the instruments driving illegal intimidation at the scale on which predictions are made.
While the prescient performance of AI calculations is moderately high in regions that are profoundly influenced by terrorism, it stays challenging to anticipate occasions that happen in locales that have not experienced illegal intimidation over an extensive stretch. Algorithms might show a generally decent by and large precision even at fine spatial and worldly goals. Terrorist events happened in under 2% of the week cells considered in our worldwide examination. Information irregularity decreases the exactness of the models, which is the number of week cells that experienced psychological warfare and have been effectively anticipated separated by the absolute number of week cells anticipated to experience illegal intimidation. This implies that to forestall an enormous extent of terrorist occasions in a locale that is not quite influenced by illegal intimidation, significant assets are needed to study huge regions where terrorism might happen.
Alongside conflict among researchers about the meaning of terrorism, the accessibility, spatiotemporal inclusion, and the nature of openly accessible information on illegal intimidation and its potential drivers stay a significant obstruction to precisely foresee terrorism worldwide and at strategy pertinent scales. But illegal intimidation information and socio-economical drivers are turning out to be more detailed, complete, and all the more effectively available. Likewise, the continuous advancement in interpretable AI calculations is exceptionally encouraging and will make these integral assets more available to the examination local area and experts in the coming years.
As of not long ago, the understanding of models was basically held to traditional measurable models which force a parametric connection among features and the reaction like in linear regression models where components are assumed to be directly connected with the reaction, and the coefficient related with each element can be assessed and further deciphered in accordance with existing illegal intimidation speculations. In this study, the analysts utilized an interpretable AI calculation to acquire high prescient execution without compromising the interpretability of the outcomes.
The machine-learning algorithm has possibly caught complex connections of local and worldwide drivers of terrorism at a scale that is important for decision-makers. The interpretability of their model has significant advantages past its prescient capacities. Results can be studied in accordance with terrorism theories and can hence add to build trust among modelers and specialists, which is a vital stage to make these calculations to predict the future of terrorism.