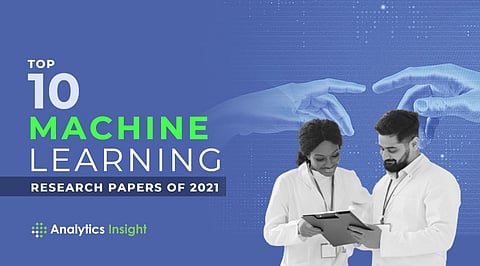
In 2021, machine learning and deep learning had many amazing advances and important research papers may lead to breakthroughs in technology that get used by billions of people. The research in this field is developing very quickly and to help you monitor the progress here is the list of most important recent scientific research papers.
By Paul Vicol, Luke Metz and Jascha Sohl-Dickstein
Presented a technique for fair-minded gradient assessment in untolled calculation charts, called Persistent Evolution Strategies (PES).
PES acquires inclinations from truncated unrolls, which speeds up streamlining by taking into consideration that frequent parameter updates while not experiencing truncation predisposition that influences many contending approaches. The researchers showed PES is extensively relevant, with tests exhibiting its application to an RNN-like task, support learning, etc.
By Lorenz Richter, Leon Sallandt, and Nikolas Nüsken
Showed tensor trains give an engaging estimate system to illustrative partial differential equations (PDEs): the mix of reformulations as far as in reverse stochastic differential equations and relapse type techniques in the tensor format hold the guarantee of utilizing latent low-rank designs empowering both pressure and effective calculation.
Following this, the scientists have created novel iterative plans including either unequivocal and quick or verifiable and precise updates. Their techniques accomplish an ideal compromise among precision and computational proficiency contrasted and SOTA neural organization-based methodologies.
By Will Grathwohl, Kevin Swersky, Milad Hashemi, David Duvenaud, and Chris J. Maddison
Proposed a general and versatile inexact examining methodology for probabilistic models with discrete factors. Their methodology utilizes angles of the probability work regarding its discrete contributions to propose refreshes in a MetropolisHastings sampler.
The specialists showed observationally that this methodology outflanks conventional samplers in numerous perplexing settings, including Ising models, Potts models, limited Boltzmann machines, and factorial secret Markov models. They additionally exhibited the utilization of their further developed sampler for preparing profound energy-based models (EBM) on high-dimensional discrete information. Further, this methodology outflanks variational auto-encoders and existing EBM.
By researchers at Cornell University, Yucheng Lu and Christopher De Sa
Showed how decentralization is a promising strategy for increasing equal machine learning systems. The scientists gave a tight lower bound on the emphasis intricacy for such techniques in a stochastic non-raised setting. The research papers expressed the pinnacle bound uncovered a theoretical gap in the realized assembly pace of many existing decentralized preparing calculations, like D-PSGD. The scientists demonstrated the lower bound is tight and feasible.
The scientists further proposed DeTAG, a useful tattle-style decentralized calculation that accomplishes the lower bound with just a logarithm hole. Exactly, they contrasted DeTaG and other decentralized calculations on picture order assignments and noticed that DeTAG appreciates quicker combination than baselines, particularly on unshuffled information and inadequate networks.
By Facebook AI researchers Yuandong Tian, Xinlei Chen, and Surya Ganguli
Examined different strategies around self-supervised learning (SSL) and proposed an original theoretical methodology, DirectPred that directly sets the straight indicator dependent on the measurements of its inputs, without angle training.
On the ImageNet dataset, it performed equivalently with more unpredictable two-layer non-straight indicators that utilize BatchNorm and beat a direct indicator by 2.5 percent in 300-age preparing (and 5 percent in 60-age). The specialists said DirectPred is persuaded by their theoretical investigation of the non-straight learning elements of non-contrastive SSL in simple linear networks.
By Bengio, Y., Clune, J., Lipson, H., & Yosinski, J.
Evaluated the generality versus particularity of neurons in each layer of a profound convolutional neural network and report a couple of astonishing outcomes. Adaptability is adversely influenced by two unmistakable issues: (1) the specialization of higher layer neurons to their unique errand to the detriment of execution on the objective undertaking, which was normal, and (2) enhancement hardships identified with dividing networks between co-adjusted neurons, which was not normal.
By Amorim, D.G., Barro, S., Cernadas, E., & Delgado, M.F.
Assessed 179 classifiers emerging from 17 families (discriminant investigation, Bayesian, neural organizations, support vector machines, choice trees, rule-based classifiers, boosting, stacking, arbitrary timberlands and different outfits, summed up direct models, machine learning, closest neighbors, partial least squares and principal segment relapse, calculated and multinomial relapse, various versatile relapse splines, and different strategies).
By Dong, X., Gabrilovich, E., Heitz, G., Horn, W., Lao, N., Murphy, K., & Zhang, W.
Introduction of Knowledge Vault, a web-scale probabilistic knowledge base that combines extractions from Web content (obtained via analysis of text, tabular data, page structure, and human annotations) with prior knowledge derived from existing knowledge repositories for constructing knowledge bases. They employ supervised machine learning methods for fusing distinct information sources.
By Lowe, D.G., & Muja, M.
New algorithms for rough closest neighbor coordinating and assess and contrast them and past algorithms. To scale to exceptionally enormous informational indexes that would some way or another not fit in the memory of a solitary machine, we propose a disseminated closest neighbor coordinating with a system that can be utilized with any of the algorithms depicted in the research papers.
By Huang, G., Huang, G., Song, S., & You, K.
Presented the research papers showing the status of the theoretical exploration and practical signs of progress on the Extreme learning machine (ELM). Aside from arrangement and relapse, ELM has as of late been reached out for bunching, include determination, illustrative learning, and numerous other learning errands. Because of its momentous productivity, effortlessness, and amazing speculation execution, ELM has been applied in an assortment of areas, like biomedical designing, PC vision, framework ID, and control and advanced mechanics.