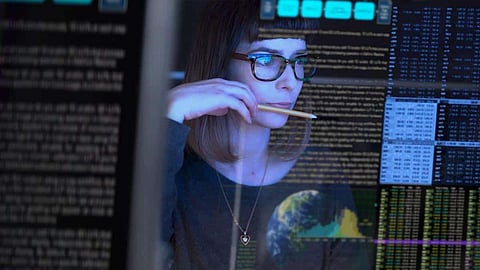
A machine learning algorithm could automate the way toward annotating training datasets for predictive analytics tools, a promising progression as certain datasets become progressively huge. Created by analysts at Massachusetts Institute of Technology (MIT), the model automatically learns highlights predictive of vocal cord issues and could help create strategies to anticipate, analyze, and treat the disorder.
Training datasets regularly comprise of many wiped out and healthy subjects, yet with moderately little data for each subject. Experts must discover those viewpoints or features in the datasets that will be significant for making forecasts.
MIT computer researchers are hoping to quicken the utilization of artificial intelligence to improve medical decision-making, via mechanizing a key step that is generally done by hand and that is winding up increasingly difficult as certain datasets develop ever-bigger.
The field of predictive analytics holds increasing guarantee for helping clinicians analyze and treat patients. Machine learning models can be trained to discover patterns in patient information to help in sepsis care, structure more secure chemotherapy regimens, and anticipate a patient's danger of having breast cancer or passing on in the ICU, to give some examples.
This procedure can be monotonous and costly, the MIT team stated, and it has turned into all the more challenging with the ascent of wearable gadgets. Experts would now be able to screen patients' biometrics over significant lots, including their dozing patterns or voice action. After just one week of monitoring, experts could have billions of data tests for each subject.
"It's winding up progressively simple to gather long time-series datasets. However, you have doctors that need to apply their insight to naming the dataset," said lead author Jose Javier Gonzalez Ortiz, a PhD student in the MIT Computer Science and Artificial Intelligence Laboratory (CSAIL)."We need to expel that manual part for the experts and offload all feature engineering to an ML model."
The team manufactured a machine learning model to automate this procedure, concentrating on prescient highlights of vocal cord issue. The highlights originated from a dataset of around 100 subjects, each with about seven days of voice-observing data and a few billion examples.
In other words, few subjects and a lot of data for every subject. The dataset contains sign caught from a little accelerometer sensor mounted on subjects' necks.
In trials, the model utilized features consequently removed from these data to classify, with high exactness, patients with and without vocal cord nodules. These are injuries that are developed in the larynx, regularly as a result of patterns of voice abuse, for example, belting out melodies or hollering. Critically, the model achieved this task without a huge set of hand-named data.
This research adds to MIT's endeavors to improve machine learning algorithms and quicken their adoption in healthcare. In June 2019, a group from the establishment built up a robotized framework that can assemble more data from pictures used to train ML models. The system can be utilized to improve the training of machine learning models, helping them to all the more likely to identify anatomical structures in new scans.
"We're trusting this will make image segmentation progressively open in reasonable circumstances where you don't have a great deal of training information," Amy Zhao, an alumni student in the Department of Electrical Engineering and Computer Science (EECS) and Computer Science and Artificial Intelligence Laboratory (CSAIL) said at the time. "In our methodology, you can figure out how to imitate the varieties in unlabeled scans to cleverly orchestrate a huge dataset to train your system."
While the feature extraction tool can be adjusted to learn patterns in any infection or condition, it has critical ramifications for distinguishing vocal cord issue and cautioning individuals to possibly harming vocal behaviors, the team said.
Going ahead, the researchers need to screen how various medicines, including surgery procedure and vocal treatment, impact vocal conduct. The team likewise hopes to utilize a comparable technique on electrocardiogram information, which is utilized to track strong elements of the heart.
Join our WhatsApp Channel to get the latest news, exclusives and videos on WhatsApp
_____________
Disclaimer: Analytics Insight does not provide financial advice or guidance. Also note that the cryptocurrencies mentioned/listed on the website could potentially be scams, i.e. designed to induce you to invest financial resources that may be lost forever and not be recoverable once investments are made. You are responsible for conducting your own research (DYOR) before making any investments. Read more here.