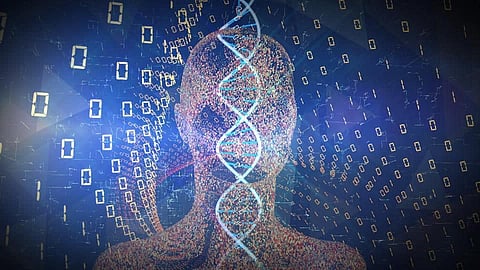
According to a study published in the journal Radiology, when machine learning is combined with common heart scan, it can predict heart attacks and other cardiac events better than traditional risk models. Observing the worldwide data, heart disease is the most common and leading cause of death in both men and women, especially in the United States. Consequently, precision in risk assessment is mandatory for early intervention to say diet, exercise, drugs including cholesterol-lowering statins.
In this context, CCTA (Coronary Computed Tomography Arteriography) provides with a highly detailed set of images of the heart vessels and happens to be a refining risk assessment tool.
The study lead author Kevin M. Johnson, M.D., CCTA recently investigated a machine learning system which can mine the myriad details in CCTA-obtained images for a better and comprehensive prognostic picture. Johnson is an associate professor of radiology and biomedical imaging at the Yale School of Medicine in New Haven, Conn.
Johnson quoted that, "Starting from the ground up, I took imaging features from the coronary CT… Each patient had 64 of these features and I fed them into a machine learning algorithm. The algorithm can pull out the patterns in the data and predict that patients with certain patterns are more likely to have an adverse event like a heart attack than patients with other patterns."
To draft this study, Johnson and his skilled colleagues compared the ML approach with technologies including CAD-RADS and other vessel scoring system in around 6892 patients. The team followed the patients for nearly 9 years after CCTA and found that there were 380 deaths from all causes out of which 70 were from artery disease. Also, 43 patients reported heart attack.
Out of all the outcomes, ML approach better detected which patient would have a cardiac event as compared to others. Regarding the cholesterol problem and when to start statin, ML score ensured 93 percent of patients who have the probability to receive drug whereas CAD-RADS predicted only 69 percent of the event.
Talking about the ML approach, Kevin said, "The risk estimate that you get from doing the machine learning version of the model is more accurate than the risk estimates you're going to get if you rely on CAD-RADS. Both methods perform better than just using the Framingham risk estimate. This shows the value of looking at the coronary arteries to better estimate people's risk."
Following ML approaches contribution to vessel scoring, it can be predicted that it would increase the participation of non-invasive imaging to cardiovascular risk assessment. Also, the vessel score derived from machine learning could be put together with non-imaging risk factors including age, gender, hypertension, and smoking to develop more comprehensive risk models.
Johnson further described the ML approach and said, "Once you use a tool like this to help see that someone is at risk, then you can get the person on statins or get their glucose under control, get them off smoking, get their hypertension controlled, because those are the big, modifiable risk factors."
Currently, Kevin Johnson is working on a paper that takes outcomes of the ML-derived vessel score and combines them with the non-imaging risk factor to gain a bigger picture and perspective. According to him, if people's ages and particulars like smoking, diabetes, and hypertension added, it would enhance the overall power of the method and improve the overall outcomes.
Join our WhatsApp Channel to get the latest news, exclusives and videos on WhatsApp
_____________
Disclaimer: Analytics Insight does not provide financial advice or guidance. Also note that the cryptocurrencies mentioned/listed on the website could potentially be scams, i.e. designed to induce you to invest financial resources that may be lost forever and not be recoverable once investments are made. You are responsible for conducting your own research (DYOR) before making any investments. Read more here.