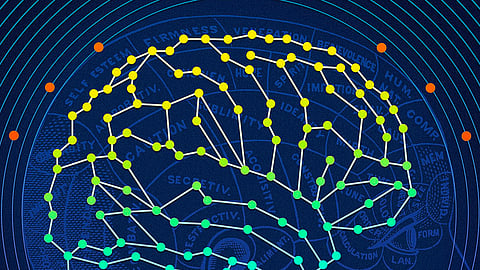
Imagine you enter your neighbor's kitchen room for the first time. As soon as you step your foot inside, you can figure out where the essential food items are present if the refrigerator is having two doors or a single door, what do their plates look like, etc. We humans barely have any trouble identifying things. This is because we have evolved ourselves through reasoning, learning, and cognitive thinking. Even toddlers can do the same when left in a playroom full of colorful balls. However, this is impossible for today's state-of-the-art neural networks.
Since the rollout of AI, there have been some impressive advances in data processing. Yet it still far behind replicating human behavior and intelligence in its totality. This has given scientists many restless nights until now. A collaborating team of researchers from the MIT-IBM Watson AI Lab, MIT's Computer Science and Artificial Intelligence Laboratory, Alphabet's DeepMind, and Harvard University have found a solution to this. Introducing CoLlision Events for Video REpresentation and Reasoning (CLEVRER), which is a new, large-scale video reasoning data set, is developed using principles of neural networks and symbolic AI, commonly termed as neuro-symbolic modeling.
The formation of such a system was primarily based on the need for an AI that can multi-task in a variety of domains, and can read data from a variety of sources (text, video, audio), whether the data is structured or unstructured. The neural network or deep learning allows large-scale pattern recognition and capturing complex correlations in massive data sets as inputs and hence interprets it using the natural language of various questions and answers. While, symbolic AI is good at capturing compositional and causal structure. Also, later can filter out irrelevant data too. Combining these two can help overcome each other's limitations. A neural network is a data-driven approach that is contrary to the rule-based approach of symbolic AI. So without a doubt combining these will help us reap the better of the two.
CLEVRER is built on a data set of Clevr, another data set released in 2016. This data set comprises of 20,000 5-second synthetic videos of colliding objects (three shapes of two materials and eight colors) on a tabletop created with the Bullet physics simulator, together with a natural language data set of questions and answers about objects in videos. More than 300,000 questions and answers are categorized as descriptive (e.g., "what color"), explanatory ("what's responsible for"), predictive ("what will happen next"), and counterfactual ("what if"). After analysis of CLEVRER, researchers found three elements — recognition of the objects and events in the videos, modeling the dynamics and causal relations between the objects and events, and understanding of the symbolic logic behind the questions — to be the most important. Using this, they built a model — Neuro-Symbolic Dynamic Reasoning (NS-DR) — that explicitly joined them together via a representation with 88.1% accuracy that other baseline models.
The creative of hybrid AI on the grounds of neuro-symbolic modeling is set to be one of the exciting, innovative trends of 2020. According to David Cox, head of the MIT-IBM Watson AI Lab, "The two forms of AI complement each other well and together can build more robust and reliable models with fewer data and more energy efficiency."
He continues, "Neural networks can get you to the symbolic domain, and then you can use a wealth of ideas from symbolic AI to understand the world." Neural networks help prioritize how symbolic programs organize and search through many facts related to a question as neural networks use analyzing correlations in the data, unlike logic powered symbolic AI. This eases reasoning about entities and their properties and relationships rather than depending on a human programmer. Therefore to conclude, a neuro-symbolic system employs both logic and language processing to a problem, similar to human brains. So maybe in future, we shall have a hybrid AI that is instilled with common sense reasoning and domain knowledge into deep learning, thereby much closer to be our digital twin!