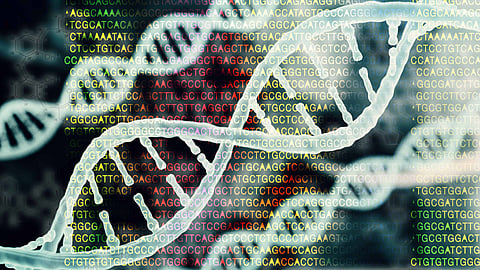
Artificial Intelligence (AI) is a powerful force that is already reshaping our lives, environment, and interactions. It may be defined as a program whose aim is to produce human-like cognitive processes and potentially even improving on them. AI has many facets: it may be algorithmic as in game-playing programs or take a control-theoretic approach as in autonomous vehicles. It may also manifest itself as linguistic ability, creativity, spatial reasoning, learning, and many others. We are now recognizing that AI has begun to make huge inroads to Life Sciences, be it making discoveries from huge biological data using machine learning, combining health records and genomic data of various types, discovering new drugs or drug targets, finding new groups of cell types, making diagnosis, or customizing health procedures as in precision medicine.
There are numerous areas where the life science industry uses AI effectively today. The following sections describe six of those areas.
Histopathology image analysis and automated diagnosis were ripe for AI, given the technological progress in the digitalization of complete histology slides, which permit all microscopic magnifications. AI and pattern recognition, combined with complex algorithms and automated immunohistochemical measurement systems, have advanced pathologists' ability to oversee the analysis and concentrate on more difficult cases.
Life sciences companies are exploring how AI can be leveraged to identify new indications for existing products or research new candidates. Examples include, but are not limited to:
• Using sophisticated learning algorithms to mine real-world structured and unstructured data to uncover insights can lead to the identification of new mechanisms of disease, potential new line extension, and design for pre-clinical experiments.
• Knowledge gaps in how candidates act on proteins to aid the design of new drugs can be filled.
• Knowledge can be extracted in real-time from commercial, scientific, and regulatory literature, allowing researchers to identify competitive white space, eliminate blind spots in research, and discover disease similarities.
Across the industry, product development timelines range from seven to 10 years from discovery to launch, with sights set on reducing them to five to seven years. Advancements in AI and machine learning to reduce the time it takes to develop, manufacture, and launch new patient therapies support the goal of reducing overall product development timelines. Scientists are integrating research data, lab data, and clinical data, in combination with new information sources (e.g., social media and wearables) across the drug development spectrum, creating a holistic picture of the drug development candidate. Improving ways to acquire and mine data in real-time allows scientists to use AI and machine learning to make improved decisions faster, which will accelerate the product development and scale-up process.
Compliance is often a burden on companies and requires an approach to mitigate costs while meeting regulation. The European Medicines Agency's policies 0070 and 0043 are examples of regulations that have recently been introduced requiring companies to anonymize or redact patient information in clinical submissions. While generalist automation tools are available, many do not satisfy the accuracy needed to meet the policy's requirements. New applications are emerging utilizing advanced algorithms based on customized NLP technologies incorporating scientific-specific taxonomies and text-mining models. Using these advanced models, it is possible to identify keywords, phrases, and data patterns (such as adverse event dates) that may require redaction or anonymization. These new applications provide a higher level of accuracy required to meet the policy requirements while also automating manual activities.
Nearly 80 percent of clinical trials fail to meet their patient enrollment deadlines. Combining unanalyzed historical structured and unstructured clinical trial data into advanced AI models can improve and accelerate clinical site and patient selection decisions by highlighting high-probability targets. Continuing to use advanced AI models during active clinical programs enables real-time adjustment and course corrections. Engaging high-probability success targets at clinical trial onset in combination with a willingness to make real-time course corrections increase the likelihood of meeting patient enrollment timelines.
Life sciences companies are responsible for up-to-date information on the safety of their products. Pharmaceutical labels (e.g., package insert, patient information leaflets) are an important way to communicate safety information. When an update to the reference label is necessary for safety reasons, there are multiple considerations (e.g., artwork printing, production run dates) for submitting the local product label to the corresponding health authority. Most companies' SOP timelines that govern submission due dates are more stringent than what health authorities require, thus increasing workload and cost. Combining and connecting the appropriate data points with machine learning and predictive analytics can determine an optimal submission date.