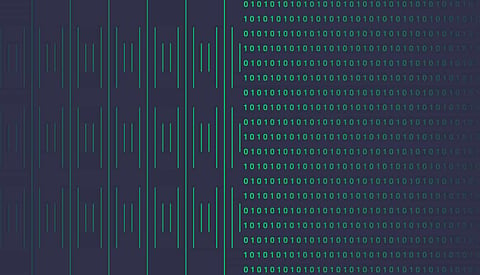
Most of the work of computer science is committed to making an interpretation of human thoughts into a structure that machines can comprehend. Code can be high-level like Python or Java or Ruby, which makes it simpler for people to read and write. However, underneath those languages, the manner in which contemplations are communicated must draw nearer and closer to the bits themselves through assembly language and object code, the 1s and 0s.
You could state that the historical backdrop of programming has been a consistent walk away from the machine and toward the human, moving increasingly more of work of translation into compute (which has become less expensive) and assuaging the human specialists (who are in every case excessively uncommon). Natural language processing, similar to the graphical user interfaces (GUIs) we came to know through personal computers, is another enormous advance toward that path. The main issue is, there are genuine cutoff points to what NLP can do.
Normal language preparing tries to complete two things: comprehend and produce human language. You may call these the passive and active sides of NLP. Natural language understanding can come in numerous structures. On the simplest level, you could classify a text: for instance, you may have a lot of messages and you need to know whether they are angry or happy since you work in client support.
NLP can do that, and it's called sentiment analysis. Or on the other hand, possibly you're an HR department and you need to sort resumes coming in for job descriptions; for example is the individual applying for the job of UX designer somebody who has UX experience, or somebody who is parachuting into the profession from a past career as a trapeze artist? NLP can do that, as well.
In another study published recently on the preprint server Arxiv.org, researchers at the University of Toronto and the Vector Institute, an independent nonprofit devoted to advancing AI, propose BabyAI++, a platform to contemplate whether descriptive texts help AI to sum up across dynamic situations. Both it and a few baseline models will soon be accessible on GitHub.
One of the most remarkable strategies in machine learning, reinforcement learning, which involves prodding software agents toward objectives by means of rewards is likewise one of the most defective. It's sample inefficient, which means it requires countless process cycles to finish and without extra data to cover variations, it adjusts inadequately to conditions that differ from the training environment.
It's speculated that earlier information on tasks through structured language could be joined with reinforcement learning to moderate its deficiencies, and BabyAI++ was intended to scrutinize this hypothesis. To this end, the platform expands upon a current reinforcement learning framework, BabyAI, to create different dynamic, color tile-based environments alongside texts that portray their layouts in detail.
Most of the computer processing applied to human language is only a rearranging of strings, skating delicately over symbols that are only the petrified artifact of a live intelligence. The computer doesn't have the foggiest idea what they connote. It has no visceral intuition of the items to which they refer. Feeding a computer a string about a "little house in the enormous woods close to the bright creek where the trout used to bounce" will bring out no picture or nostalgia, at least not on its own. For the greater part of the historical backdrop of computers, we have put away text in machines so as to relay the words later to different people, who were called upon to supply the importance.
Inside BabyAI++, each level is divided into two setups: training and testing. In the training setup, the operator is exposed to all tile and color types in the level, yet a few blends of color-type pairs have waited. In the testing design, all color-type pairs are empowered, driving the operator to utilize language grounding to relate the sort of the tile to the color.
BabyAI++'s levels comprise of articles that can be picked up and dropped; doors that can be unlocked and opened; and different tasks that the specialists must embrace. Like the conditions themselves, the tasks are arbitrarily created and they're imparted to the operator through "Baby Language," a compositional language that utilizes a subset of English jargon. The co-authors affirm that this shows descriptive texts are helpful for operators to sum up situations with variable dynamics by learning language-grounding.
Join our WhatsApp Channel to get the latest news, exclusives and videos on WhatsApp
_____________
Disclaimer: Analytics Insight does not provide financial advice or guidance. Also note that the cryptocurrencies mentioned/listed on the website could potentially be scams, i.e. designed to induce you to invest financial resources that may be lost forever and not be recoverable once investments are made. You are responsible for conducting your own research (DYOR) before making any investments. Read more here.