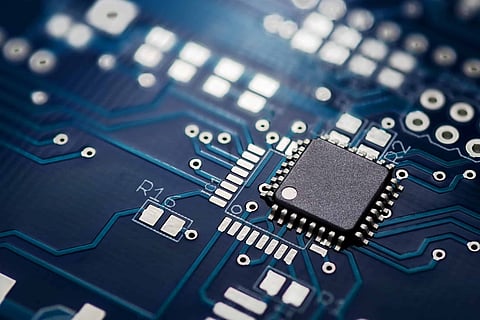
In today's digital age, artificial intelligence (AI) has earned a name for itself as a futuristic agent that can lead us to a world powered by machines mimicking human intelligence. As days pass by, the use of AI technology is becoming a significant factor in business success, healthcare services, customer engagement, and more. Further, this technology is pushing the envelope of human capability in a manner that humans-machine coexistence will be viewed as a symbolic relationship in the coming days. And this means combining the cognitive, emotional strengths of humans with that of machines (computational capabilities, data analysis, pattern recognition, and next action determination based on statistics).
Meanwhile, AI's big data, machine learning, and automation potential have forced policymakers and business leaders worldwide to plan for a future where AI is a core competency. Also, the proliferation of AI functions has significantly disrupted production and labor processes. The end-user industries of AI comprise manufacturing, agriculture, healthcare, media and advertising, oil & gas, BFSI (Banking Financial Services and Insurance), automotive, transportation, and retail. However, it is also important to remember that all these capabilities of AI are powered by high-performing AI chips (GPUs, FPGAs and ASICs), thus it also has an impact on the semiconductor industry too.
In reality, AI impacts the growth of the semiconductor sector in two ways, i.e., building demand for innovative technologies and enhancing the product fabrication process. The former creates new marketing opportunities and facilitates manufacturing process improvements. For instance, the Tensor Processing Units (TPUs) enables Google applications that utilize AI to execute things like identifying words people are uttering in audio recordings, spot objects in images and videos, and pick up essential emotions in written text.
In the case of the latter, machine learning, neural network, support vector machines are used in processes like wafers defects inspection and classification, optical metrology, chip manufacturing, and modeling, photoresist contour prediction, semiconductor production result prediction, wafer process control and monitoring and so forth.
According to Accenture Semiconductor Technology Vision 2019, an annual report by Accenture, almost three-quarters of semiconductor executive respondents (77%) informed to have adopted AI within their business or are piloting the technology. In addition, nearly two-thirds of semiconductor executives (63%) expect that AI will have the greatest impact on their business over the next three years, compared with just 41% of executives across 20 industries. Another survey by the Redline Group revealed that AI could allow semiconductor companies to capture 40 to 50% of the total value from the technology stack, representing the best opportunity they've had in decades. Additionally, many AI applications will require specialized end-to-end solutions, necessitating changes to the semiconductor supply chain.
One of the main challenges faced in the semiconductor supply chain is chip production processing time. According to IEEE, chip production processing time refers to the period between initial processing and the final product. And during this time, up to 30 percent of production costs are lost to testing and yield losses. Hence by embedding AI applications into the production cycle allows companies to systematically analyze losses at every stage of production so manufacturers can optimize operating processes. This ability will become even more valuable when working with next-generation semiconductor materials, which tend to be more expensive (and volatile) than traditional silicon.
It is well known that every nation and enterprise is striving to dominate the AI market. Companies are implementing AI to improve customer satisfaction, expedite decision-making, and reduce inefficiencies. Even in Asia, governments and corporates are becoming much more proactive in developing their AI capabilities. From China to Japan, Singapore to India, policymakers across Asia are developing national-level plans for how AI can be used to enhance domestic and regional competitiveness. And here, too, semiconductors can play a huge role in determining the trajectory of the nations in an AI-powered future. And this also implies geopolitical tension to weigh in, creating an immense bottleneck in the regional AI industry.
Last year in July, Japan placed restrictions on exporting to Korea three elements crucial for semiconductors' production: hydrogen fluoride, fluorinated polyimide, and photoresists. The photoresists and hydrogen fluoride are made of Chinese raw materials, more precisely rare earth metals. Owing to China's status quo as the leading supplier of raw materials and one of the largest manufacturing hubs of the world, the Mandarin region plays a key role in the AI supplier market in Asia. According to government sources, Japan produces about 90% of fluorinated polyimide, about 70% of hydrogen fluoride, and 90% of photoresists. This makes Japan almost a full monopolist in this production chain, making it very difficult for its consumers to find substitutes or build up enough capabilities to avoid Japan entirely. So, while South Korea has the tag of being the world's largest semiconductor and chipmakers, such instances of regional restriction could change every institute's outlook, which is part of the semiconductor and AI market.