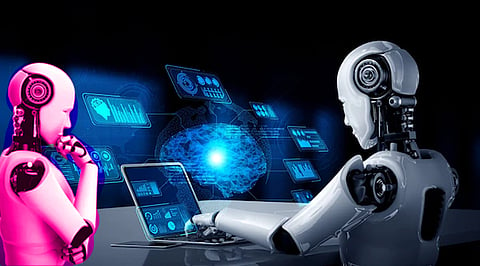
It's impossible to understand what's going on in the enterprise technology space without first understanding data and how data is driving innovation. Synthetic data can be employed in a privacy-safe environment, meaning that users and developers can access it without any concerns over disclosing sensitive information. The main artificial intelligence methods used to create deepfakes are based on deep learning and involve training generative neural network architectures, such as autoencoders or generative adversarial networks (GANs). A 2018 Deloitte survey, for instance, found that "data issues" such as privacy, accessing, and integration was considered the biggest challenges in implementing AI and data analytics initiatives. By generating non-identifiable datasets, however, synthetic data generation can be a vital privacy-enhancing technology that does not carry the regulatory or legal burdens associated with disclosing personal data.
Synthetic data is data that you can create at any scale, whenever and wherever you need it. Crucially, synthetic data mirrors the balance and composition of real data, making it ideal for fueling machine learning models.
What makes synthetic data special is that data scientists, developers, and engineers are in complete control. There's no need to put your faith in unreliable, incomplete data, or struggle to find enough data for machine learning at the scale you need. Just create it for yourself.
Deepfake technology is used in synthetic media to create falsified content, replace or synthesizing faces, and speech, and manipulate emotions. It is used to digitally imitate an action by a person that he or she did not commit.
Bringing Back the Loved Ones!
Deepfakes have a lot of potential users in the movie industry. You can bring back a decedent actor or actress. It can be debated from an ethical perspective, but it is possible and super easy if we do not think about ethics! And also, probably way cheaper than other options.
Just imagine a world where you can get physics classes from Albert Einstein anytime, anywhere! Deepfake makes impossible things possible. Learning topics from its masters is a way motivational tool. You can increase the efficiency, but it still has a very long way to go.
In this technology-driven world, the need for training data is constantly increasing. Synthetic data can help meet these demands. For an AI and data analytics system, there is no 'real' or 'synthetic'; there's only data that we feed it to understand. Synthetic data creation platforms for AI training can generate the thousands of high-quality images needed in a couple of days instead of months. And because the data is computer-generated through this method, there are no privacy concerns.
At the same time, biases that exist in real-world visual data can be easily tackled and eliminated. Furthermore, these computer-generated datasets come automatically labeled and can deliberately include rare but crucial corner cases, even better than real-world data. According to Gartner, 60 percent of the data used for AI and data analytics projects will be synthetic by 2024. By 2030, synthetic data and deepfakes will have completely overtaken real data in AI models.
There are a number of business use cases where one or more of these techniques apply, including:
Join our WhatsApp Channel to get the latest news, exclusives and videos on WhatsApp
_____________
Disclaimer: Analytics Insight does not provide financial advice or guidance. Also note that the cryptocurrencies mentioned/listed on the website could potentially be scams, i.e. designed to induce you to invest financial resources that may be lost forever and not be recoverable once investments are made. You are responsible for conducting your own research (DYOR) before making any investments. Read more here.