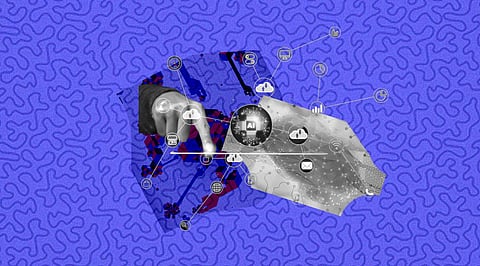
Even as many businesses experiment with AI using rudimentary machine learning (ML) and deep learning (DL) models, a new sort of AI called symbolic AI is emerging from the lab, with the potential to transform both AI's function and its relationship with its human overseers. There are two groups in AI history: symbolic AI and non-symbolic AI, each of which takes a distinct approach to build an intelligent system. The symbolic method tried to create an intelligent system with explainable actions based on rules and knowledge, whereas the non-symbolic method aimed to create a computational system modeled after the human brain. The ultimate objective of computer science is to create an AI system capable of thinking, logic, and learning. Most AI systems today, on the other hand, only have one of the two abilities: learning or reasoning. While symbolic approaches excel in thinking, explaining, and managing large data structures, they struggle to establish their symbols in the perceptual world.
To address problems, Symbolic AI employs a top-down approach (example: chess computer). "You'll find what you're searching for if you look hard enough." Search is the symbolic AI technique. The computer's step-by-step testing of potential solutions and confirmation of the results is referred to as "search" in this scenario. A chess computer that "imagines" millions of different future moves and combinations and then "decides" which moves have the highest probability of winning based on the results is a good illustration of this. The analogy to the human mind is obvious: everyone who has spent a significant amount of time playing a board or strategy game has at least once "played through" motions in their thoughts before reaching a choice. Neural networks can help traditional AI algorithms since they add a "human" gut feeling to them, reducing the number of movements that need to be computed. By integrating these technologies, AlphaGo was able to defeat a human in a game as complex as Go. If the computer had computed all possible movements at each step, this would not have been possible.
The difficulty of modifying ideas once they were stored in a rule's engine was one of the key stumbling blocks of symbolic AI or GOFAI. Expert systems are monotonic, which means that the more rules you add, the more information is encoded in the system, but new rules cannot destroy previous knowledge. Monotonic is a term that refers to only one direction. Machine learning algorithms may be retrained on fresh data, they are better at recording provisional information that may be retracted later if required; for example, when data is non-stationary, they will modify their parameters depending on that new data.
The second issue with symbolic thinking is that the computer does not understand what the symbols signify, implying that they are not necessarily related to any other non-symbolic representations of the world. This contrasts with neural networks, which may connect symbols to vectorized representations of data, which are just translations of raw sensory input.
The obvious question then becomes, "Who are the symbols for?" Are they of any use to machines? Why should robots employ symbols if they allow human sapiens to communicate and manage information based on underlying physiological constraints? Why can't machines communicate using vectors or some noisy language shared by dolphins and fax machines? Let us make a prediction: when machines do learn to communicate with one another intelligibly, it will be in a language that humans will be unable to comprehend. Perhaps the bandwidth of words is insufficient for high-bandwidth devices. Perhaps they require additional dimensions to express themselves clearly. Language is only a keyhole in a door that has been bypassed by machines. The natural language might, at best, be an API that AI provides to humans so that they can ride on its coattails; at worst, it could be a diversion from what actual machine intelligence entails. However, we have conflated it with the pinnacle of success since natural language is how we demonstrate our intelligence.
Knowledge Graph creation: We create a Knowledge Graph for our clients as a starting point for constructing any chatbot or voice assistant. The Knowledge Graph is the data structure of the future, according to us. It will serve as the foundation for all future AI-based applications.
Process implementation: Digitization and preparation of organizational data are unavoidable for businesses. As a result, the creation of a Knowledge Graph is unavoidable sooner or later. Onlim establishes the organizational procedures and workflows that will be necessary for the future for frequent knowledge documentation and update.
Decades of experience are brought to bear: The Onlim team has a combined knowledge of graph construction expertise of many decades. Customers may benefit and learn from this large knowledge base as they move toward their goal of implementing a chatbot/voice assistant.
Maximum convenience: Online takes care of the nitty-gritty details in the background, enabling businesses to concentrate on data preparation and addition. The Online Conversational AI platform allows you to easily edit or alter any information at any time.
A comprehensive approach: Online provides a complete experience by assisting clients through all stages of the process. From storing information in the form of a Knowledge Graph to providing chatbots or voice assistants with the capacity to absorb facts, respond appropriately, and allow users to complete desired transactions such as purchases, the possibilities are endless.