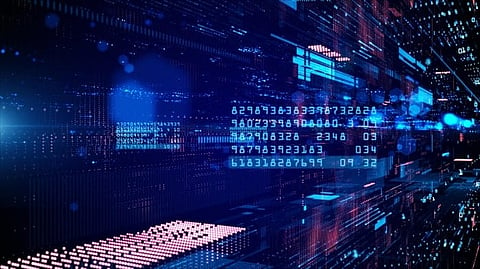
While the entire purpose of business intelligence (BI) is to find behavioral patterns in the data and infer future trends or actions that can benefit the business, many enterprises have been missing a key component: mainframe data. Without this precious core data, much of which is hidden in mainframe environments, BI and modern analytics won't live up to their potential.
It has often been stated that data is "the new oil" that can power economic growth. If that's true, then it is also true that mainframe data has been largely untapped, confined to use in traditional systems of record and given only the most limited exposure to modern analytics.
Enterprises must clearly find better ways of accessing, analyzing, and using the data they already possess. The mainframe must yield its secrets.
The mainframe environment has evolved with consistency for more than half a century. It's been the rock on which many businesses built their IT infrastructure. Mainframes reliably sustained business processes, research, and even helped businesses adapt to the World Wide Web.
However, while the rest of IT has galloped toward shared industry standards and even open architectures in on-premises systems and in the cloud, mainframe has stood aloof and unmoved. It operates largely within a framework of proprietary hardware and software that did not readily share data – and perhaps didn't need to. But with the revolutionary pace of change, especially in the cloud, old notions of scale and cost have been cast aside. As big and as powerful as mainframe systems are, there are things the cloud can now do better.
Analytics is one of those things. For example, in the cloud no problem is too big. Effectively unlimited scale is available if needed. Just as significant, a whole host of analytic tools like Kibana, Splunk and Snowflake, have emerged to better examine not only structured data but also unstructured data which abounds in mainframes.
These tools have largely been deployed on "new" data from newer on-premises and cloud systems, yielding extremely important insights. But those insights could be enhanced, often dramatically, if mainframe data, historic and current, were made available in the same way or, better yet combined – for instance in modern cloud-based data lakes.
Given an opportunity to use mainframe data more strategically and finally monetize its long-hidden value, most organizations would do so. But until now this has been difficult to accomplish. A combination of technology limits and pricing policies of incumbent vendors have made data analytics on the mainframe frustrating and moving data off the mainframe cost prohibitive.
Most methods of mainframe data movement, typically described as "extract, transform, and load" (ETL), require intensive use of mainframe computing power. This can interfere with other mission critical activities such as transaction processing, backup, and even regularly scheduled batch jobs. Moreover, mainframe software vendors typically charge in "MSUs" which roughly correlate with CPU processing loads. And all traditional data movement tasks depend on CPU processing.
New technology is now available that turns this process on its head. Data can be extracted from the mainframe and loaded to a cloud target where it can be economically transformed into any standard format, combined with other data and analyzed as much and as often as needed. The secret to this new method is the extract process almost entirely bypasses the CPU (avoiding MSU charges) and instead uses the mainframe's built-in zIIP engines (a subsidiary processing system within the mainframe) which is not part of the MSU billing system.
Regardless of the industry, an organization with the ability to liberate its mainframe data will have the capacity to monetize it, empowering new business applications, better services, and more refined operations.
For example, a bank with the capability to access mainframe data might be able to better analyze customer behavior to develop new, tailor-made solutions or anticipate needs (such as a particular kind of loan at a given stage of a customer's life). This also allows the bank to gain better insights for core activities like making the best lending decisions and meeting security and regulatory requirements.
In healthcare, mining historic data has long been recognized as a potential way to develop predictive medicine as well as a tool for assessing the effectiveness of treatment or even potentially identifying undesirable side-effects of treatment.
Aside from global banks and the healthcare industry, governments, airlines, insurers and other financial institutions can all reap the benefits of accessing and monetizing mainframe data. According to ITPro, 70 percent of Fortune 500 businesses have their core business operations powered by mainframe systems. Since mainframe technology isn't going away, businesses need a better way to tap into their wealth of data.
The cloud was designed for handling vast amounts of data. The costs of storing, managing, analyzing and using data in the cloud are generally more favorable than any other option available on-premises. CEOs and key decision makers must find the right partner to extract mainframe data so they can move and transform it to a modern, standard format in the cloud where it is accessible to cloud apps. Once in the cloud in a manageable, malleable format, the sky is the limit in terms of what enterprises can do and how they can benefit from this now accessible, usable data.
Grasping the potential of legacy data hidden away in mainframes can be a challenge in itself. However, when business leaders get a taste of what is possible and see the ease of full, transparent integration of their mainframe data, they become quick converts to the process.
This evolution in mainframe data management will change how BI evolves over the next decade. User experience and product offerings will become more personalized. Companies will customize their offerings according to historical data liberated from the mainframe to enhance their business analytics. Enterprises that follow this path will have an opportunity to excel while those that don't will likely be left behind.
Gil Peleg, Founder and CEO, Model9 has over two decades of hands-on experience in mainframe system programming and data management, as well as a deep understanding of methods of operation, components, and diagnostic tools.
Starting his career in the IDF computer center as a member of the mainframe cyber security team, he became deeply familiar with mainframe system internals as he studied vulnerabilities and examined data protection techniques.
Prior to founding Model9, Gil worked at IBM ITSO center in Poughkeepsie, NY, and later at the IBM Haifa Research Lab, working on mainframe storage development, and acquiring data management practices. He then continued in the storage and data management domains, working at companies such as XIV and Infinidat.
He is a co-author of eight IBM Redbooks on z/OS Implementation. He holds a B.S.c in Computer Science and Mathematics.
Join our WhatsApp Channel to get the latest news, exclusives and videos on WhatsApp
_____________
Disclaimer: Analytics Insight does not provide financial advice or guidance. Also note that the cryptocurrencies mentioned/listed on the website could potentially be scams, i.e. designed to induce you to invest financial resources that may be lost forever and not be recoverable once investments are made. You are responsible for conducting your own research (DYOR) before making any investments. Read more here.