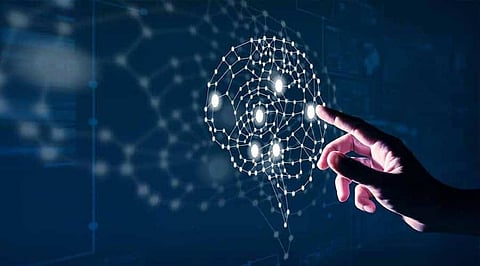
Most of the business leaders claim that the lack of qualified personnel with skills in artificial intelligence (AI) has been a major barrier to its implementation across businesses. With more and more companies choosing to leverage artificial intelligence and its applications like machine learning, deep learning, and more, the knowledge gap can prove a costly affair. While data scientists are helping to build AI applications for data mining, analyzing, filtering the raw data, along with selecting an algorithm to train machine learning models, their roles are diversifying in recent years. Coupled with the acute talent shortage, as the job positions for data scientists are rapidly increasing, companies face the risk of trailing behind in the race of digital transformations. Therefore, business leaders are looking to automate some of the roles and responsibilities of a data scientist. This lead to the emergence of AutoML or automated machine learning. Because of its ability to perform data pre-processing, ETL (extract, transform, load) tasks, and transformation, AutoML rose to popularity last year.
AutoML refers to the process of automating an end-to-end process of leveraging machine learning algorithms to real-world problems. By deploying autoML tools, gathering data and turning it into actionable insights has become much convenient for companies. Doing so increases effectiveness and democratizes machine learning so that non-specialists can apply machine learning to their issues effortlessly. Employing autoML also enables hyperparameter enhancement of AI model settings. Using trial and error and experimentation, it also helps in deciding the best model available for the data introduced.
For instance, Google's AutoML-Zero uses simple mathematical concepts as building blocks to generate complete machine learning algorithms. By employing a sophisticated trial-and-error process, AutoML-Zero identifies the best performing algorithm (from a set of 100 of algorithms, each having unique task capabilities) and retained for future iteration. Furthermore, autoML automates the data storage, identifies leaky spots and misconfigurations to ensure accuracy and precision in the result, while eliminating the risk of pervading biases. Besides it can open up new opportunities for businesses, who are restricted by time constraints and resources to create machine learning models that were until recently inaccessible to them.
Data is multiplying at an unprecedented rate. With reports about data explosion to increase by tenfold, artificial intelligence and machine learning-based data analytics will also see a proliferation of a new wave of data demands. This would mean that autoML will emerge as a promising resource for many companies. AutoML helps small scale and medium scale companies achieve the level of analytical sophistication they need to collect data and extract insights from it without finding and hiring dozens of machine learning experts. It also leaves minimal room for error compared to human counterparts. From handling self-service deployment of models by data scientists, model lifecycle management, feature engineering to imputation and rescaling and model training autoML can free data scientists to focus on other important tasks.
According to a report by McKinsey, autoML will likewise have an impact on hiring patterns too. For instance, AutoML practitioners, like biochemists in pharma research, will be able to perform simpler data-science tasks. It will also empower lesser AI-skilled persons to build and deploy artificial intelligence or machine learning-based model and monitor its performance too. It will further, maximize the business output more efficiently, and increasing its accuracy and turnaround time. In short, autoML will help bridge the talent gap with its enormous wide-ranging capabilities. Though it certainly would not replace data scientists, it puts the power of advanced ML directly in the hands of business users.
Join our WhatsApp Channel to get the latest news, exclusives and videos on WhatsApp
_____________
Disclaimer: Analytics Insight does not provide financial advice or guidance on cryptocurrencies and stocks. Also note that the cryptocurrencies mentioned/listed on the website could potentially be scams, i.e. designed to induce you to invest financial resources that may be lost forever and not be recoverable once investments are made. This article is provided for informational purposes and does not constitute investment advice. You are responsible for conducting your own research (DYOR) before making any investments. Read more here.