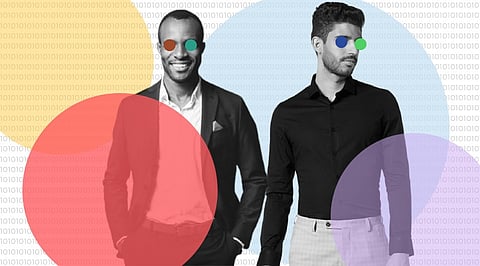
Data science is a booming field. Also, we have come across so many guides and tutorials on how to become a successful data scientist. A career in data science is a promising one because as a data scientist you will be around data and trying to make sense out of it, which can help in making data-driven decisions. However, did you know there are different types of data scientists? Most of the people embarking on a career in data science often face this dilemma of understanding which type of data scientist they are.
If you are interested in statistics, you will like building new statistical theories for big data. On the other hand, if you are a math lover, your love for maths can help with analytic business optimization. This is just the tip of the iceberg, there are many types of data science jobs to choose from and make a successful career in. Here, we present to you some of the most popular types of data scientists to help you understand the difference and choose the preferred one.
A remarkable data analyst is the core of an organizations' data endeavors. A strong foundation in statistics is the thing that carries rigor to data-driven decision-making. A data analyst takes a look at industry information to tackle business-important inquiries and provide insights to respective teams. They make sense of enormous datasets, create hypotheses, and impart these to business leaders. If you wish to become a data analyst then you need to have a solid sense of the processes that are more than just data. As a data analyst, you will focus on what matters. This implies managing the substantial responsibility of addressing business questions and gathering bits of knowledge from existing data.
These data science categories are chalked out by Harvard Business Review. These data scientists are basically product managers, designers, etc. that make decisions based on data, for example, which content to license, which sales lead to follow, which medication is less inclined to cause a hypersensitive response, which site page design will prompt greater engagement or more buys, which email will yield higher income, or which explicit aspect of a product user experience is suboptimal and needs attention. These data scientists design, define, and implement metrics, run and interpret experiments, create dashboards, draw causal inferences, and generate recommendations from modeling and measurement.
As a data scientist for machines, you will be taking steps on the basis of information a machine gives you. For example, recommendation systems, which suggest what shirt a client may like or what medication a doctor should give depending on a designed optimization function. Contingent upon your engineering foundation, such work items are either provided legitimately to the production system or if they are prototypes they are given off to software engineers to help implement, optimize and scale them.
This is one of the most popular data science types. The machine learning engineers are less solid than the statisticians at details and less solid than the software engineers at software development.
However, what they do bring is a comprehension of model development, programming design, and model deployment that can be fundamental for particular kinds of organizations and datasets. ML engineer takes the prototyped model proposed by the data analyst and makes it usable in the production. They make programs that control gadgets and foster algorithms that assist machines with identifying designs in their data, fathom commands, and even figure out how to settle on their own decisions.
This is data analysis in the conventional sense. The field of statistics has consistently been about number crunching. A solid statistical base qualifies you to extrapolate your enthusiasm for various data scientist areas. Hypothesis testing, confidence intervals, Analysis of Variance (ANOVA), data visualization, and quantitative research are some of the important skills possessed by statisticians, which can be extrapolated to pick up expertise in explicit data scientist fields.
Statistics knowledge, when clubbed with domain knowledge, (for example, marketing, risk, actuarial science) is the ideal blend to land a statistician's work profile. They can create statistical models from big data analysis, complete experimental design, and apply theories of sampling, clustering, and predictive modeling to information to decide future corporate activities.
We have research scientists and scholastics to thank for the lively advances in fields like deep learning, computer vision, and natural language processing. They will, in general, examine issues that are more crucial with more time in researching. Tech giants and research labs usually hire research data scientists to introduce breakthroughs in existing technologies. They likewise dominate in writing research, reproducible experiments, and publishing papers at conferences.
Who to hire completely depends upon your needs and the current phase of your data operation. For example, the needs of a small-scale organization are different from a tech giant. Hence, small enterprises tend to hire data scientists who have extensive knowledge and can juggle multiple roles, managing different types of data in data science. Further, if your product is such that it depends solely on machine learning, you will definitely need a machine learning engineer that can bring the model to production.
On the other hand, if you wish to make data-driven decisions on a whole, then you might need statisticians and data analysts that can churn out insights from data and translate it across the decision-makers of the organization.