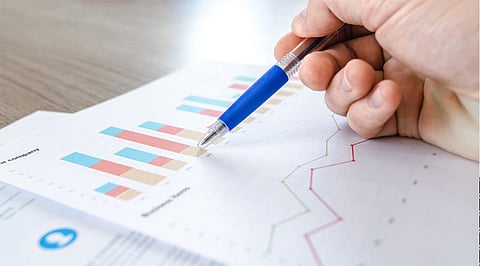
Data Types are a crucial concept in statistics that must be grasped in order to appropriately apply statistical measures to your data and, as a result, make accurate conclusions about it. This blog article will walk you through the various data types you'll need to understand in order to do effective exploratory data analysis (EDA), which is among the most undervalued aspects of a machine learning program.
Did you know that statistics only has four types of data? Have you ever sat down with your data and pondered where to begin?
This article provides an overview of the many types of data required for proper exploratory data analysis.
Data types are crucial notions in statistics because they allow us to appropriately apply statistical measures to data and conclude certain assumptions about it. Because you may apply certain factual metrics only for specific data kinds, having a good understanding of the various data types is critical for Exploratory Data Analysis or EDA.
Likewise, you must know the data analysis and type of data analysis you are dealing with in order to choose the appropriate perceptual approach. You may think of data types as a way to organize different sorts of variables. If you go deeper into statistics, you'll find that there are only two types of data: qualitative and quantitative data. However, there is a segmentation after that, and it is divided into four categories of data. Data types are like a road map for properly conducting a statistical investigation!
Data types are crucial because statistical procedures can only be applied to specific data kinds. Continuous data must be analyzed differently from categorical data, or else the analysis will be incorrect. Knowing what kind of data you're working with allows you to select the best technique of analysis.
1. Qualitative or Categorical Data: Nominal Data and Ordinal Data
2. Quantitative or Numerical Data: Discrete Data and Continuous Data
Data that falls into categories is referred to as qualitative data, also referred to as categorical data. Qualitative data does not have a numerical value. Categorical data includes categorical variables that define characteristics such as a particular gender, origin, and so on. Categorical measurements are specified using plain language requirements rather than numerical values.
Categorical data can sometimes contain numerical values (quant values), but such values are not mathematically meaningful. Date of birth, favorite game, and school code are instances of categorical data. The quantitative value is held by the birthday and school postcode, although it has no numerical meaning.
Subdivided into two parts:
Nominal data is a kind of qualitative data that aids in the labeling of variables without offering a numerical value. The nominal scale is another name for nominal data. It can't be quantified or ordered. However, data may be both primary and secondary data at times. Symbols, characters, words, gender, and other nominal data are instances of nominal data.
The grouping method is used to evaluate the nominal data. The data are sorted into categories in this approach, and the frequency or percent of the data may then be computed. Pie charts are used to visually display this information.
Examples of Nominal Data:
What languages do you speak?
What is your nationality?
Ordinal data/variables are those that have a natural order to them. The disparity between the data values is not recognized in nominal data, which is a crucial characteristic. This variable appears often in surveys, finance, marketing, and questionnaires, among other places.
A bar chart is often used to depict ordinal data. Many visualization technologies are used to analyze and understand this data. Tables may be used to represent the data, with each row representing a different category.
Examples of Ordinal Data:
Opinion
Numerical data also termed quantitative data, symbolizes a numerical value (i.e., how often, how much, or how many). Numerical data is the data about a certain thing's quantities. Length, height, size, mass, and other numerical statistics are instances of numerical data. Regarding the data sets, quantitative data may be divided into two categories. Numerical data is divided into two categories: discrete data and continuous data.
Subdivided into two parts:
Only discrete values can be used with discrete data. There are only a finite number of potential values in discrete information. Those numbers can't be split in any meaningful way. Things may be tallied in full numbers here.
Example of Discrete Data: Number of children in a class
Data that can be computed is known as continuous data. It has an unlimited number of possible values inside a specified range that can be chosen.
Example of Continuous Data: Temperature range
In this article, you learned about the various data types used in statistics. You studied what interval, nominal, ordinal, and ratio measurement categories are, as well as the distinction between discrete and continuous data. Moreover, you now understand which statistical measures and visualization approaches are appropriate for specific data types. You also learned how to convert category variables to numeric variables using several approaches. This allows you to do a large portion of an exploratory study on a dataset.