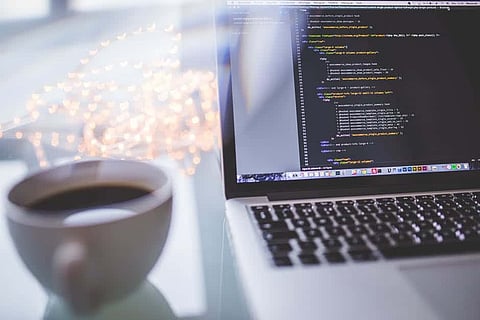
Choosing the best programming language for data science can be a very challenging task as all the languages have their own standouts in terms of parameters such as performance speed, libraries, extensions, interaction with the web and other apps, etc. Since many industries are adopting data analysis, it is better to understand the characteristics of all the tools to see which tool is better for the tasks at hand.
As for data science freshers, language selection primarily depends on their forte and subject inclination. Some tools may be well suited for programming lovers than mathematics lovers and vice versa. Let us do a comparative study of all the programming tools used for various phases of data analysis in terms of their strong and weak points.
As popularity for data science amongst businesses has increased, so has the popularity of the language for data analytics. It is free and open-source and currently has more than 6000 packages contributed by the community of developers, including statisticians and data scientists. The popularity of the language comes from this wide range of packages and modules for statistics and data analysis, and its reporting/data visualization tools.
R makes the code for statistical models concise and relies on step-by-step subroutines for each task. It is a procedural language that sets it apart from other object-oriented languages such as Java and C++. The important features include integration with other languages (Java, C), easy interaction with databases (Excel, PostgreSQL, etc.) and also with many statistical packages (SAS, Stata, etc.).
For beginners in data science or business analytics, one can start with R if you come from statistics or mathematics background and are low on the programming experience. We have listed major pros and cons of the language here.
Pros:
1. Statistical analysis abilities: It can be attributed to the fact that R was developed by statisticians and can make it easy to work on data using several algorithms. The community is instrumental in problem-solving and constant updates of packages and tools.
2. High-quality visualisation: The language allows communication of the data findings through high –quality visual tools such as charts, graphs, and using libraries like ggplot2, ggvis, rCharts, etc. The Shiny package also makes it easy for using visuals in web-based applications.
Cons:
1. Low performance: It is one of the lowest rated languages in terms of performance as it was not made for programmers in mind.
2. No coding standards: As it was built for flexibility, it does not impose strict coding rules. Hence, both good and bad codes can be built in R.
Python can be considered as the second most popular language after R for data analytics. It is widely used by the machine learning community for analyzing unstructured data and mining. Compared to other object-oriented programs, it makes the lines of code shorter.
The major mining or analytics is performed using the libraries NumPy, pandas, SciPy and the library matplotlib is used for plotting of data. Apart from these, Python comes with a rich collection of libraries that can be considered as its USP. It is good to start with Python if you are a fresh graduate and are well-versed with programming.
Python is a combination of statistically strong points of R and scalability of languages like Java. It has one of the biggest online support communities where problem-solving becomes very easy.
1. Great for machine learning: The libraries like TensorFlow, Numpy, keras, pandas greatly make Python the most preferable for machine learning and now is also used for developing deep learning models.
2. Integration with apps: Python makes integration with web apps easy using Flask, Pyramid and can be easily plugged into the production system. It is a single tool that manages the entire workflow.
1. Not good for specialized data tasks as compared to R
2. Falls short in analytics and graphic capabilities compared to R
Matlab was developed by MathWorks which also releases timely toolbox updates and new features . It is a comparatively expensive tool which depends on the number of concurrent users. One of the key features of Matlab is its huge array of toolboxes and libraries that support various tasks like machine learning, image processing, etc.
It is a go-to software for simulations, prototyping and algorithm design. In terms of graphics and visualizations, it may be on a downside compared to R. You don't need to know heavy computer science fundamentals to master Matlab as it is more mathematics centric.
Matlab makes complex matrix operations easy but can be hard to use if the data cannot be represented easily in terms of matrices.
A lot of programmers prefer building prototypes on Matlab, perform analysis and then code applications on Python or Java.
1. Advanced tool boxes: They make building codes, signal processing, machine learning, image processing, etc. easier. Matlab has a powerful simulink tool that can be used by experts in physical sciences too.
2. Easy documentation: The users can easily refer to the documentations for every command and function which helps the programmer whenever he's stuck.
3. Powerful visuals: plotting data, making charts comes easier in Matlab.
1. Lack of source codes compared to python.
2. High cost
3. Poor integration with external apps
Java is a powerful performer and best used for building enterprise-level applications. It is an open-source environment which consists of many libraries, APIs, plugins and Java Virtual machine. This makes it a preference for web-based applications. Also, distributed processing and storage frameworks like Hadoop have been developed in Java.
However, Java contains fewer statistical libraries and is not suitable for data exploration. It also lacks specialized data structures and graphing capabilities. The power of Java can be better leveraged by integration with R. Java has a massive community of developers which means that there are lots of excellent documentation around.
Speed and Scalability: This is the reason why many tech giants are using Java as a backbone for data engineering tasks. Moreover, it is used to build large scale systems.
Poor performance in statistical modeling and data visualization. Least preferable for data analysis.
Julia is an open-source program that has surpassed other languages in terms of execution speed which makes it great in performance. The language is mainly known for its mathematical capabilities. Julia is faster than R and better than Python in terms of scalability.
It integrates one of the best libraries for linear algebra, signal processing, etc. Julia has a growing developing community that are providing external packages at a rapid pace. Julia is capable of filling the gap in functionality provided by other languages mentioned. It has a powerful graphical notebook in collaboration with Jupyter called IJulia.
1. Amazing Speed
2. Combines functionality of R and Python
1. Not as much ready for industry adoption
2. Still growing repository of tools and packages
3. Not a big developer community yet
SCALA is a Java-based language which runs on JVM and is used for building machine learning programs at larger scales. It combines functional paradigms with object-oriented programming. It used the Akka library that supports concurrent models.
SCALA is a relatively difficult language to master as it is based on mathematical principles making it suitable for mathematically oriented programmers. For big data tools like Apache Spark, using SCALA has many advantages.
1. Flexible syntax
2. Shorter programs compared to Java
1. Poor compatibility with earlier versions
2. Functional programming not upto the mark compared to Java
Conclusion:
R and Python may turn out to be the favorites among the analytics professionals and one can begin learning them at any point in their career. Start-ups must focus on R first and then integrate Python programming mainly for developing applications. Employees may have to focus on learning both if the industry demands it as statistics and programming both are integral to analytics. If you love developing mathematical models, then Matlab can be a good start.
I hope this article gave you more clarity on different programming languages.
Join our WhatsApp Channel to get the latest news, exclusives and videos on WhatsApp
_____________
Disclaimer: Analytics Insight does not provide financial advice or guidance. Also note that the cryptocurrencies mentioned/listed on the website could potentially be scams, i.e. designed to induce you to invest financial resources that may be lost forever and not be recoverable once investments are made. You are responsible for conducting your own research (DYOR) before making any investments. Read more here.