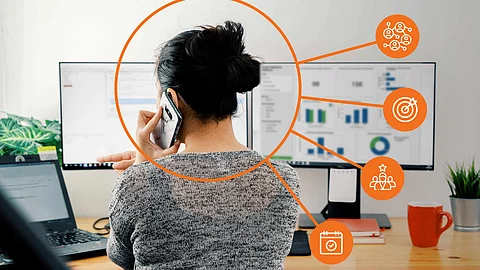
Data science isn't really one single thing, skillset or system. This is the reason data science is constantly said to be an 'interdisciplinary part' of science that consolidates arithmetic, human behavior and workflow studies, flexible utilization of logical frameworks and a core employment of algorithms. This makes being a data scientist truly difficult work, as though algorithmic rationale wasn't at that point really extreme.
Something other than data analytics, something other than big data insight, something other than the capacity to deal with new surges of raw unstructured data and something beyond realizing how to drive a database while blindfolded, data scientists need to understand business and be flexible super-performers.
What are the habits that make a data scientist successful?
Information-hungry people make great data scientists. Truly. Studies uncover more than a fourth of the data scientists surveyed documented their first bit of code before they even crossed 16 years of age. However, starting young can't be named as an achievement of accomplishment of those, who set off late in the realm of coding like after 26, 36% of them are presently holding the charming posts of senior or higher-level developers, which is a serious thing.
Self-teaching is something that each engineer of any age wants to rehearse. A recent review found, albeit 67% of data scientists bag computer science degrees, over 74% of them are self-taught some way or the other.
Some studies propose that since writing computer programs is focused on independent research planned for comprehending new difficulties, self-education is a significant piece of being a successful developer. In picking what to learn next, the best core value is to plant yourself in one discipline and learn tools as a way to develop. Tools will consistently change. Eventually, it's interest and enthusiasm for programs that should fuel the drive to learn new devices and adapt to tech's evolving landscape."
A Data Scientist is constantly an individual with a win-win approach. If he isn't having a win-win approach then he isn't a Data Scientist. This is the most significant habit that a fledgling in Data Science should create or any individual who has as of late got a major disappointment in his interview. We frequently get rejected in our interview at enormous organizations as Data Scientist, often our forecasts turn out wrong and regularly we get neglected to finish our objective. However, a successful data scientist is one who has already won over his mind and has an inspirational standpoint towards life. He realizes failures are a piece of work. They don't cause him to lose however "empower him to learn new everyday" and a data scientist wants to learn new things and new advances everyday.
The work of data scientists is, by definition, experimental. They should be permitted to try and the results could possibly be fruitful, yet do enough experiments in the correct areas and you will discover the value," said Asplen-Taylor. Considering problem-solving experimentation further, data scientists need to follow not to lead, for example, they should be given an issue to fix, which implies they need business analysts to characterize the issue and after their experimentation stage, they need somebody to test the result of their projects, approve the outcomes (so they are not marking their own schoolwork) and they need IT individuals who will place their models into a production environment and to then record them (which is key from a data privacy perspective guaranteeing that what they are doing is transparent) and bolster the models.
If you would prefer not to build attribution models until Google automates you out of a job, you have to discover new business issues to illuminate. Have an ear for why organizations missed income targets. Those are the business issues they haven't solved and are happy to pay for answers for. By what method can data science or AI tackle these difficulties? There are a ton of prescient issues; something happened that the organization didn't envision. That is regularly a supply chain disruption or a change in customer preference.
Likewise, data science or machine learning capabilities issues; an organization doesn't have the capabilities to analyze their data. These are issues shared by a ton of organizations. Figure out how to take care of these issues and advertise your capacities. You're significantly progressively important to an organization if you can tune in for their business issues and blend solutions.
Once in a while the experience that counts, and not simply education. Managers look for problem-solving skills in any employee, it has gotten one of the most sought after abilities to be found in any desirable employee considerably more than the programming language capability. Demonstrating computational thinking or the capability to separate huge, complex issues is similarly as important (if not more so) than the benchmark technical skills required for a job.
Likewise, it states, organizations value a scientist's experience and portfolio unquestionably more than their educational qualifications and training. Qualifications that by and large support the resume like the prestige of degree, education level, skill endorsements or certificates rank the least among what organizations care about the most.