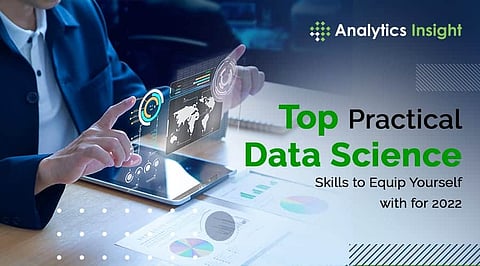
Data science is the field that is emerging to be the best program attracting a lot of young minds. It is not only about theoretical knowledge, but it is more about the practical part too. The practical data science skills help in gaining a grip over real-life problems that exist in the market. There remains a core set of data science from scratch skills that are foundational for all general practitioners and specialists, who want to be employable with full-stack capabilities. Let's see the top practical data science skills to equip yourself with for 2022. These are the top data scientist skills that can add great value to their resumes.
Learning how to write robust SQL queries and scheduling them on a workflow management platform like Airflow is vital as a data scientist. It will help you in building core data pipelines and improving the insights that are gathered making things easier. Being able to write robust pipelines for your projects instead of depending on data analysts will also save a lot of time of yours. This is one of the practical data science skills to learn to stay in demand in the market.
If you are building models, or exploring new features, or even performing deep dives, data wrangling is vital. Data wrangling means transforming your data from one format to another format. This is one of the practical data science skills to learn to stay in demand in the market. And while coming to feature engineering, it is a type of data wrangling that refers to extracting features from the raw data.
GitHub is essentially a cloud-based repository for files and folders. While Git is not the skill to acquire first, it is important for almost every coding-related role. Git allows you to collaborate and work with projects in parallel with others. It keeps track of all versions of your code. This is one of the practical data science skills to learn to stay in demand in the market.
To build a visually stunning dashboard with over 95% accuracy, one needs to have great communication skills to communicate ideas and plans to others. So storytelling is referring to how you communicate your insights and models. Storytelling and communication are severely undervalued skills in the tech world. So storytelling is the skill that can separate juniors from seniors and managers. This is one of the practical data science skills to learn to stay in demand in the market.
Building regression and classification models, predictive models are not something that you will always be working on, but it's something that employers will expect you to know if you're a data scientist. To give some perspective, mission-critical models had a significant impact on the business. This is one of the practical data science skills to learn to stay in demand in the market.
Many machine learning algorithms were considered 'block boxes' for a long time because it wasn't clear how these models derived their predictions based on their respective inputs. SHAP and LIME are two techniques that tell you not only the feature importance for each feature but also the impact on the model output, similar to the coefficients in a linear regression equation. With SHAP and LIME, you can create explanatory models and also better communicate the logic behind your predictive models. This is one of the practical data science skills to learn to stay in demand in the market.
A/B testing is a form of experimentation where you compare two different groups to see which performs better based on a given metric. A/B testing is arguably the most practical and widely-used statistical concept in the corporate world. Why? A/B testing allows you to compound 100s or 1000s of small improvements, resulting in significant changes and improvements over time. If you're interested in the statistical aspect of data science, A/B testing is essential to understand and learn. This is one of the practical data science skills to learn to stay in demand in the market.
Join our WhatsApp Channel to get the latest news, exclusives and videos on WhatsApp
_____________
Disclaimer: Analytics Insight does not provide financial advice or guidance. Also note that the cryptocurrencies mentioned/listed on the website could potentially be scams, i.e. designed to induce you to invest financial resources that may be lost forever and not be recoverable once investments are made. You are responsible for conducting your own research (DYOR) before making any investments. Read more here.