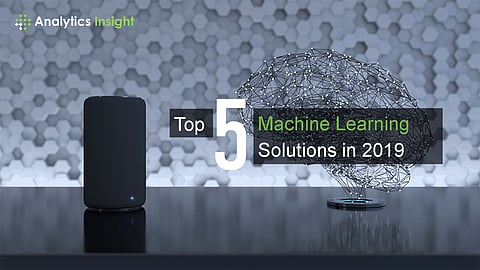
In the present hyper-rapid cloud computing period, AI solutions drive exponential advancement in improving frameworks. ML's capacity to use Big Data analytics and recognize patterns offers a critical upper hand to current organizations. Frequently utilized in integration with artificial intelligence and deep learning, Machine Learning (ML) utilizes complex statistical modeling. These mind-boggling frameworks may live in a private cloud or public cloud. Regardless, the progression of time supports ML: as more information is added to a task and analyzed after some time, Machine Learning delivers increasingly precise the outcomes.
The worldwide ML market totalled $1.4 billion of 2017, as indicated by BCC Research. It is assessed to top $8.8 billion by 2022, a stunning compound annual growth rate (CAGR) of 43.6%. The ML industry is evolving quickly. ML-based startups are always hopping into space. Established sellers are presenting an assortment of offers that use ML in some structure. Dealing with the decisions and choices can be confounding. Let's see some of the best solution providers in the ML space, in light of the features they offer, analyst opinions, client feedback and independent research.
Alteryx Inc. gives advanced analytics tools intended to limit the exertion required to perform data analysis and to improve the way toward accessing and integrating information from numerous data sources. The suite comprises of three items – The Alteryx Designer desktop, Alteryx Server and Alteryx Analytics Gallery.
Alteryx offers incorporation with various significant accomplices, including Tableau, AWS, Teradata, Microsoft, DataRobot, Salesforce, Oracle, Cloudera and Qlik. ML functions highlight parallel model analysis with predictive analytics, alongside the ability to computerize work processes and different procedures.
Amazon SageMaker is a service that empowers an engineer to fabricate and train ML models for predictive or analytical applications in the Amazon Web Services (AWS) public cloud. Machine Learning offers an assortment of advantages for companies, for example, advanced analytics for client information or back-end security threat detection, yet it tends to be hard for IT experts to deploy these models without prior experience and skills. Amazon SageMaker plans to address this challenge, as it gives built-in and basic ML algorithms, alongside different tools, to improve and fasten up the procedure.
Amazon SageMaker supports Jupyter notebook, which are open source web applications that aid engineers share live code. For SageMaker clients, these notebooks incorporate drivers, packages and libraries for normal deep learning platforms and systems. A developer can come up with a pre-constructed notebook, which AWS supplies for an assortment of applications and use cases, at that point alter it as per the data set and schema the engineer needs to train. Developers can likewise utilize custom-built algorithms written in one of the upheld ML structures or any code that has been bundled as a Docker container image. SageMaker can pull information from Amazon Simple Storage Service (S3), and there is no practical farthest point to the size of the data set.
Google Cloud Machine Learning (ML) Engine is a managed service that empowers data scientists and developers to construct and convey better ML models to creation. Cloud ML Engine gives training and prediction services, which can be utilized together or separately. Cloud ML Engine is a demonstrated service utilized by organisations to tackle issues running from identifying mists in satellite pictures, guaranteeing food security, and reacting multiple times quicker to client messages.
ML includes training a PC model to discover patterns in information. The more great information that you train a very much planned model with, the more smart your solution will be. You can come up with your models with different ML systems, including scikit-learn, XGBoost, Keras, and TensorFlow, a best in class deep learning structure that powers many Google products, from Google Photos to Google Cloud Speech. Cloud ML Engine empowers you to naturally plan and assess model architecture to accomplish an intelligent solution quicker and without specialists. Cloud ML Engine scales to use every one of your data. It can prepare any model at a large scale on a managed cluster.
Watson Studio democratizes ML and deep learning on how to quicken infusion of AI in your business to drive development. Watson Studio gives a suite of tools and a cooperative environment for data scientists, developers and area specialists. Watson Studio gives you the environment and tools to take care of your business issues by cooperatively working with information. You can pick the tools you have to investigate and visualize data, to wash down and shape data, to ingest streaming information, or to make, train, and deploy machine learning models.
IBM Watson Studio is intended to oblige an assortment of independent platforms and different kinds of power users. This incorporates data engineers, application developers and data scientists. The outcome is solid cooperation capacities. Among its best highlights: a robust engineering, solid algorithms and a ground-breaking capacity to execute ML.
Azure Machine Learning Studio has risen as a main solution in the managed cloud space. It conveys a visual tool that guides engineers, data scientists and non-data scientists in planning ML pipelines and solutions that address a wide range of tasks. Microsoft Azure offers a program based, visual simplified writing environment that requires no coding. Gartner positions Microsoft a "Visionary" in its MQ. The solution offers a high state of adaptability, extensibility and transparency.
Azure blue ML Studio likewise conveys extensive capacities over the full range of elucidating, diagnostic, predictive and prescriptive analytic sorts. Microsoft is proceeding to grow the features and capacities inside Azure Machine Learning. This incorporates advancing deep learning through the Microsoft Cognitive Toolkit (previously CNTK) as well as the joint ONNX open standard for neural systems. Microsoft likewise plans to automate a developing number of capacities inside the Azure Machine Learning stage.
Join our WhatsApp Channel to get the latest news, exclusives and videos on WhatsApp
_____________
Disclaimer: Analytics Insight does not provide financial advice or guidance. Also note that the cryptocurrencies mentioned/listed on the website could potentially be scams, i.e. designed to induce you to invest financial resources that may be lost forever and not be recoverable once investments are made. You are responsible for conducting your own research (DYOR) before making any investments. Read more here.