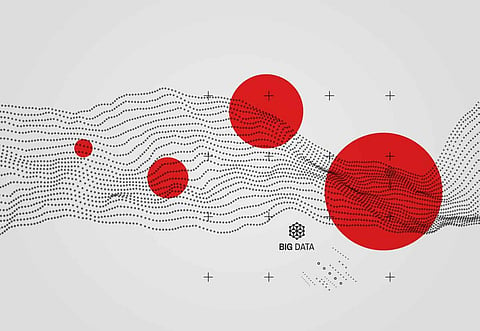
Big data is the base for the next unrest in the field of Information Technology. Organizations today independent of their size are making gigantic interests in the field of big data analytics. This traction comes as a result of the undeniable upper hands that data gives in the present market scene. Think about this, for a typical Fortune 1000 organization, a 10% expansion in accessible data can mean more than $65 million increment in the total income. Sadly, organizations are to a great extent been ineffective in taking out valuable inputs from data. As indicated by a NewVantage Partners research, just 37.1% of the organizations are of the belief that they were fruitful in their big data attempt. Henceforth, it is imperative to comprehend the unmistakable big data challenges and the solutions you should deploy to beat them.
Frequently, organizations neglect to know even the nuts and bolts, what big data really is, what are its advantages, what infrastructure is required, and so on. Without reasonable comprehension, a big data deployment project is a danger to be destined to disappointment. Organizations may squander loads of time and assets on things they don't realize how to utilize. Furthermore, if workers don't see the advantages of big data and/or would prefer not to change the current procedures for its deployment, they can oppose it and hinder the organization's advancement.
Big data, being an enormous change for an organization, ought to be acknowledged by top management first and afterward down the stepping ladder. To guarantee big data comprehension and acknowledgment at all levels, organizations need to compose various training and workshops. To see to big data acknowledgment considerably more, the deployment and utilization of the new big data solution should be checked and controlled. In any case, top management should not exaggerate with control since it might have an unfriendly impact.
Big data must be cleaned, prepared, verified, reviewed for compliance and constantly maintained. The issue with these tasks is that information comes in so quick organizations think that it's hard to play out the majority of the data preparation activities to guarantee ideal data quality. Now and again, companies basically store the majority of their approaching big data without doing a lot to it. This makes data contaminated. Also, inaccurate information can raise the danger of business decisions being founded on wrong data.
Characterize your business rules for data cleaning and preparation and search out automation tools that can perform data prep undertakings for you. Second, figure out which data you completely don't require and set up data cleansing automation at the front of your data accumulation procedures to discard this data before it ever hits your system.
Big data deployment projects involve heaps of costs. If you settle on an on-premises arrangement, you'll need to mind the expenses of new hardware, new employments like heads and developers, power, etc. Additionally, despite the fact that the required systems are open-source, regardless you'll have to pay for the development, setup, design and maintenance of new software. If you settle on a cloud-based big data solution, despite everything you'll have to procure staff as above and pay for cloud services, big data solution development as well as setup and upkeep of required systems.
In addition, in the two cases, you'll have to take into consideration future developments to maintain a strategic distance from big data development escaping out of hand and costing you a fortune.
The specific salvation of your organization's wallet will rely upon your organization's particular technological requirements and business objectives. While organizations with very brutal security necessities go on-premises. There are likewise hybrid solutions when parts of data are put away and handled in cloud and parts, on-premises, which can likewise be financially smart. Also, falling back on data lakes or algorithm improvements, whenever done appropriately, can likewise set aside extra income. Data lakes can give cheap storage chances for the data you don't have to analyze right now. Upgraded algorithms, in their turn, can diminish computing power utilization by five to multiple times. Or then again considerably more.
With everything taken into account, the way to solving this challenge is legitimately dissecting your requirements and picking a relative game-plan.
Big data integration frequently revolves around incorporating data from various business divisions into a "solitary adaptation of reality" that everybody in the business can utilize. Notwithstanding, it is similarly as challenging for IT to oversee big data that comes in all flavors and on a wide range of hardware and software platforms.
There are plenty of backend dispersed data stores. Some of these dispersed data stores are not locally supported by the platform. Depending on the data store, one should utilize an alternate API, for the most part, Python-based, to deal with these circumstances. It's not ideal. Accessing and putting away information in unsupported data stores expects developers to continually change their program for every data store. This slows advancement cycles and makes it any longer for clients to get insights from the data.
Fundamentally, extraordinary big data processing platforms make it hard to rearrange IT infrastructure for simpler information management and big data process streams. This is a huge challenge for IT.
There are software automation tools accessible with several pre-created APIs for a wide range of data, databases, and records. You may even now end up hand-building up an API on a case-by-case premise, yet these tools can complete a dominant part of the work.
Regularly, big data deployment projects put security off till later stages. Furthermore, honestly, this isn't a lot of a smart move. Big data innovations do advance, yet their security highlights are as yet disregarded since it's trusted that security will be allowed on the application level.
The precautionary measure against your conceivable big data security challenges is putting security first. It is especially significant at the phase of structuring your solution's engineering. If you don't coexist with big data security from the very start, it'll nibble you when you wouldn't dare to hope anymore.
Join our WhatsApp Channel to get the latest news, exclusives and videos on WhatsApp
_____________
Disclaimer: Analytics Insight does not provide financial advice or guidance on cryptocurrencies and stocks. Also note that the cryptocurrencies mentioned/listed on the website could potentially be scams, i.e. designed to induce you to invest financial resources that may be lost forever and not be recoverable once investments are made. This article is provided for informational purposes and does not constitute investment advice. You are responsible for conducting your own research (DYOR) before making any investments. Read more here.