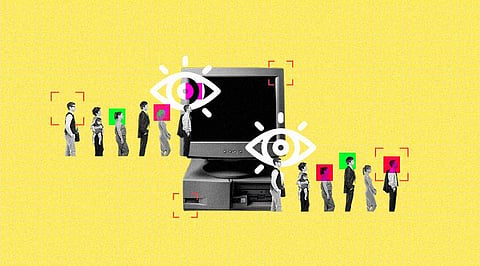
Computer vision, a field of artificial intelligence, has witnessed significant advancements in recent years. One of the key factors contributing to these advancements is the availability of high-quality open-source datasets. These datasets play a crucial role in training and evaluating computer vision datasets, enabling researchers and developers to tackle complex visual recognition tasks.
1.ImageNet:
ImageNet is a widely used dataset that contains millions of labeled images across various categories. It has been instrumental in advancing object recognition and has served as the benchmark for numerous computer vision competitions and research projects.
2.COCO (Common Objects in Context):
The COCO dataset focuses on object detection, segmentation, and captioning. It includes a diverse range of images with detailed annotations, making it a valuable resource for tasks like instance segmentation and image captioning.
3.Open Images:
Open Image is a large-scale dataset that provides a diverse collection of images with annotations for object detection, visual relationship detection, and more. It offers a vast amount of data across various domains and is continually expanding.
4.Pascal VOC (Visual Object Classes):
The Pascal VOC dataset has been widely used for object detection, classification, and segmentation tasks. It contains annotated images from various object classes and has been a popular choice for evaluating computer vision algorithms.
5.Cityscapes:
Cityscapes is specifically designed for semantic understanding and scene understanding in urban environments. It consists of high-resolution images with pixel-level annotations, making it a valuable resource for tasks like semantic segmentation and object detection in urban scenes.
6.SUN (Scene Understanding):
SUN dataset focuses on scene understanding and provides a large collection of images with detailed annotations for scene classification, object detection, and other related tasks. It covers a wide variety of indoor and outdoor scenes.
7.LFW (Labeled Faces in the Wild):
The LFW dataset is dedicated to face recognition and contains thousands of labeled face images. It has been extensively used to evaluate face recognition algorithms and has contributed to significant advancements in this field.
8.CelebA:
CelebA dataset focuses on face attribute recognition and contains a large number of celebrity images with annotations for various attributes like age, gender, and facial expressions. It serves as a valuable resource for training models related to face analysis.
9.CIFAR-10 and CIFAR-100:
CIFAR-10 and CIFAR-100 are image classification datasets that consist of small, low-resolution images across multiple classes. They have been widely used as benchmark datasets for evaluating image classification models, particularly in the early stages of deep learning research.
10.MNIST:
MNIST is a classic dataset in computer vision and is primarily used for handwritten digit recognition. It consists of a large number of grayscale images of handwritten digits, making it an essential resource for benchmarking and developing algorithms for digit recognition tasks.