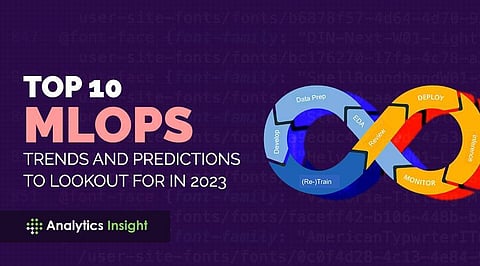
MLOps is the missing bridge between machine learning, data science, and data engineering. It has emerged as the link that unifies these functions more seamlessly than ever before. MLOps helps professionals and advanced systems to consistently deploy machine learning algorithms and solutions for enhanced productivity and effectiveness. The technology is based on the combination of an operating framework for people and technology, as well as, on an abidance for the best set of practices and proven architectural principles. In simpler terms, MLOps is the technology and people systems that empower production-level machine learning. New MLOps trends and predictions are emerging to meet the evolving challenges in scaling machine learning. The emerging advanced MLOps applications can solve a variety of human error and quality issues. Here, we have mentioned some of the top MLOps trends and predictions for 2023 that will definitely gain prominence in the industry.
An ML model can only be as reliable as the data we use to train it. A data-centric approach might help streamline machine learning pipelines easily. Experts have, time and again, demonstrated the effectiveness of this technique to monitor defects and surface inspection use cases. The benefits of good-quality data have become handy in advancing the growth of machine learning deployment.
Identifying drift is basically the concept of a dataset underlying an ML model and how it represents those changes. There are many types of drifts depending upon what specifically changed, but these drifts all represent the ways in which a model's performance can degrade over time. The ability to identify drift can be helpful in deploying successful machine-learning models.
As AI and MlL solutions are expected to increasingly make an impact on global industries, business leaders need to track and quantify the value of models in use. This visibility is quite useful for the health, security, and reputation of organizations using the models. These processes include a methodology to track model quality, visibility, and reusability by measuring the difference in effort for code development through MLops.
MLOps expands beyond the process to transform processes, people, and technologies. People participation is a key ingredient to successfully implementing MLOps. It is crucial to forming a group of business leaders or key stakeholders from different levels and departments to develop best practices.
The MLOps and machine learning industry is advancing quite rapidly. Based on reports, machine learning companies and tech service providers will continue to adopt MLOps and ML solutions. And the rising development of MLOps will trigger increased investments in machine learning.
The streamlining of AI and ML algorithms is quite problematic. Onboarding and deployment of these technologies are extremely challenging to orchestrate workloads, balance servers, to configure the right level of concurrency to scale up the amount of user traffic. There are several ways in which leaders can simplify MLOps integration, however, certain specific problems still remain.
More and more businesses will start adopting Metaflow. It will help leaders to design their workflows, run them at scale, and deploy it to production. It versions and tracks all their experiments and data automatically. Metaflow was recently open-sourced by Netflix and AWS. It can integrate with Amazon SageMaker, Python-based machine learning and deep learning libraries, and big data systems.
There is currently no single consensus on the use of a single MLOps tool or application. MLOps provides the capability to make ML systems adoptable by organizations regardless of their cloud providers or technical stacks, developing a one-stop solution that would be a substantial challenge. This is one of the major reasons why the rising number of libraries and packages in MLOps would make such an impact on businesses.
The year 2022 has witnessed a shift in promoting the best practices of productizing AI. The next steps would encouraging repeatable ML processes that will help small teams to roll out complete AI services on an ongoing basis. The transfer of AutoML to AutoMLOps should definitely leave a major impact in 2023.
Experts believe feature stores will become a mainstream component of ML tech stacks. The year 2023 will also experience an expansion of AI and MLOps across various organizations, decreased time to market for AI projects, and for organizations using online feature stores to enable real-time use cases to be implemented.