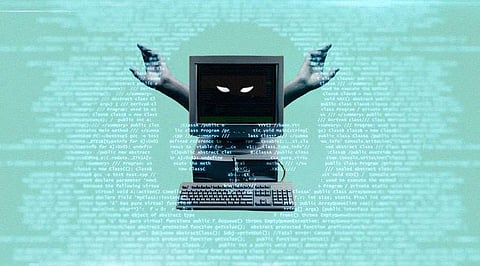
In today's digital landscape, cybersecurity is of paramount importance. With the constant evolution of cyber threats, more than traditional security measures are required to protect sensitive information and systems. This is where deep learning, a subset of artificial intelligence, comes into play. Deep learning algorithms can analyze vast amounts of data, learn patterns, and make intelligent decisions. In cybersecurity, deep learning is proving to be a game-changer.
Deep learning models excel at detecting known and unknown threats by analyzing network traffic, identifying negative patterns, and detecting anomalies in real-time. These models can swiftly identify potential cyber-attacks, providing early warning signs to prevent data breaches.
Deep learning algorithms can analyze file behavior and characteristics to identify malware. By training on large datasets of known malware samples, these models can quickly and accurately identify new strains of malicious software, helping security teams stay one step ahead of attackers.
Deep learning can enhance intrusion detection systems (IDS) by analyzing network traffic and identifying suspicious activities. These models can detect network intrusions, unauthorized access attempts, and unusual behaviors that may indicate an ongoing cyber-attack.
Phishing attacks remain a significant concern in cybersecurity. Deep learning algorithms can analyze email content, URLs, and other indicators to identify phishing attempts. By learning from past phishing campaigns, these models can detect and block suspicious emails, protecting users from phishing scams.
Deep learning can analyze user behavior patterns and detect deviations indicating insider threats or compromised accounts. By monitoring user activities and analyzing their behavior, these models can identify unusual or suspicious actions, helping organizations mitigate insider risks.
Deep learning algorithms can identify sensitive data patterns and monitor data access and transfer to prevent unauthorized data leakage. These models can analyze data flow across networks, identify potential vulnerabilities, and enforce security policies to protect sensitive information.
Deep learning models can analyze network traffic to detect patterns associated with Distributed Denial of Service (DDoS) attacks. By monitoring network flows and identifying anomalous traffic patterns, these algorithms can help organizations defend against and mitigate the impact of DDoS attacks.
Deep learning can automate the process of vulnerability assessment by analyzing code, configurations, and system logs. These models can identify vulnerabilities in software and systems, allowing organizations to address them before they can be exploited proactively.
Deep learning algorithms can analyze large volumes of threat intelligence data from various sources to identify emerging threats and trends. By continuously monitoring and analyzing threat feeds, these models can provide timely and accurate threat intelligence, enabling organizations to take proactive measures against evolving cyber threats.
Deep learning can be applied to detect fraudulent activities in financial transactions. By analyzing transactional data, customer behavior, and historical patterns, these models can identify potentially fraudulent transactions in real-time, helping organizations prevent financial losses
Join our WhatsApp Channel to get the latest news, exclusives and videos on WhatsApp
_____________
Disclaimer: Analytics Insight does not provide financial advice or guidance. Also note that the cryptocurrencies mentioned/listed on the website could potentially be scams, i.e. designed to induce you to invest financial resources that may be lost forever and not be recoverable once investments are made. You are responsible for conducting your own research (DYOR) before making any investments. Read more here.