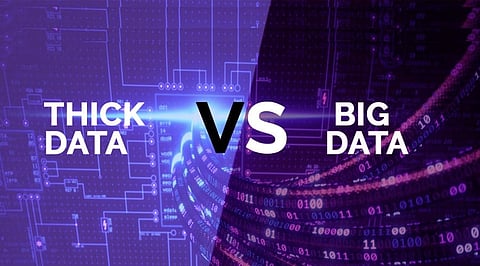
It can be challenging to make decisions, especially when faced with ambiguity. Nonetheless, company decision-makers are confronted with vital decisions on a daily basis, some of which have a significant impact on the consumers. As a result, they naturally desire to make the best decisions possible. However, doing so frequently necessitates their making near-impossible predictions about the future. Decision-makers may not even be able to foresee the future using big data and thick data combined, but they'd be able to get close.
Thick data, at its most basic level, is qualitative information (such as observations, feelings, and reactions) that offers insight into consumers' daily emotional life. When the microphone and camera are switched on during remote user testing, it is most typically collected using ethnographic research methodology.
Thick data is difficult to quantify since it seeks to discover people's emotions, stories, and representations of the environment they live in. It's mushy, and it's only collected in tiny amounts, but that doesn't make it inconsequential or insignificant. Despite this, dense data gives a never-before-seen perspective, the complexity of meaning, and emotionally-charged stories that can impact corporate decisions and increase customer empathy.
As a result, when combined with big data, it can provide a more comprehensive picture of consumer wants, requirements, and preferences. But before we go into it, let's define big data.
For all kinds of reasons, big data is the polar opposite of thick data. The fact that it is quantitative in nature—that is, it works with numbers and figures—is evident. Big data is distinguished by the fact that it is collected on such a huge scale and continues to increase exponentially over time that most computing capacity is incapable of gathering, storing, and analyzing it. Here's how it works: there's a US$138.9 billion industry dedicated to it.
Because big data is collected in such large quantities, it is difficult to analyze—it must be normalized, standardized, defined, aggregated, and so on. However, once this is done, data scientists may isolate trends and make forecasts by focusing on certain features in a large amount of data. This is fantastic and beneficial, but there is one flaw: big data is devoid of context and emotion.
By providing the crucial context and sentiment that is wasted when making big data useful, thick data can fill in the gaps in the movements that big data reveals.
It's like turning off one of your 5 senses by only using big data or thick data. Each of your perceptions is important in and of itself, providing you with knowledge of the world around you, but when they work together, they have a more comprehensive view of any scenario. The same may be said of data. Organizations may show client demands more comprehensively by merging big and dense data.
This is because different types of insights are produced by huge and dense data, and they complement one another. Big data, as previously stated, uses vast volumes of data from millions of customer data points to identify patterns at scale, whereas thick data uses smaller customer selections to reveal human-centered trends in greater depth.
Alternatively, thick data attempts to foster compassion and understanding of humans between data points, whereas big data identifies patterns by separating factors.
Many businesses declare that customers are at the center of their operations, but do they actually look at customer information? If they do, how well does their data reflect consumers' genuine feelings, social and emotional motives, and aspirations?
To sort, organize, and spread information, big data must be normalized, standardized, and defined with specific characteristics and assumptions. The viability of its models is contingent on specific assumptions. If the underlying assumptions are incorrect, the entire outcome may be incorrect. Thick data can help to mitigate this danger. While big data is based on machine learning, thick data is based on the social context of data points' interconnections. It goes above big data to explain how people live their daily lives and why they have particular tastes. This decreases the amount of depreciation that data undergoes before it can be used for analysis.
Expenditures for human-centered research are being slashed while corporations continue investing in big data technologies. It is critical that they pay close attention to ethnographic research in order to stay on top of changing client habits and mutual variances.
Our big data expenditures will be useless if we end up getting the correct answers to the wrong ones." This is where thick data can help restore equilibrium. Looking at non-quantifiable objects can help us formulate the correct questions and look past the numbers. Trust, vulnerability, panic, greediness, desire, security, and other social, emotional, and cultural aspects can all be used to gain a better understanding of consumers.
To conclude, businesses should not be overwhelmed by Big Data and should instead take systematic and cogent steps toward it. They must realize that numbers alone do not capture the emotions and experiences that consumers experience in their daily lives and that ethnographic data is essential for understanding the psychological aspects that influence the numbers.