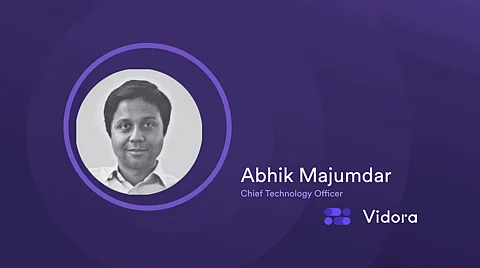
An interview with Abhik Majumdar, CTO, Vidora
In view of new computing advancements, machine learning today isn't like machine learning of the past. The iterative part of machine learning is essential on the grounds that as models are presented to new information, they can autonomously adjust. They gain from past computations to create dependable, repeatable choices and results. It's a technology that is not new – but rather one that has gained a new force. With this new force, Vidora empowers anybody in any business to deploy and utilize complex machine learning models with its self-service platform, Cortex. In a recent interview with Abhik Majumdar, CTO, Vidora, Abhik discussed how Cortex is a breakthrough in Machine Learning space and how C-level executives can seamlessly integrate Cortex into their business.
When myself, Alex Holub (our CEO), and Philip West (our Head of Product) left Ooyala to found Vidora, we saw that while many businesses wanted to embrace ML, most of them were held back by time, cost, and lack of technical expertise to build scalable, company-wide solutions. At Vidora, we sought to address these issues with our self-service ML platform, Cortex. We automated the most difficult parts of the pipeline – data pre-processing, feature cleaning, and feature engineering – and in doing so, launched a product which is intuitive, easy-to-use, and scalable. Cortex is now deployed with some of the world's largest businesses, including News Corp, Walmart, and Discovery, and is being used to tackle a variety of business problems.
My own interest in ML began at IIT in Kharagpur, where I studied Electronics and Electrical
Communications Engineering. After I graduated, I moved to the US to pursue my Ph.D. in Electrical Engineering and Computer Sciences at UC Berkeley, where I worked on probabilistic algorithms and information theory. This work led me to Ooyala where I was one of the first employees along with my Vidora co-founders Alex and Philip.
When we first founded Vidora, our product focused on areas like 1:1 personalization and forecasting user behavior. But we quickly realized that businesses were interested in solving a huge range of problems using ML, especially the large enterprises Vidora works with. We've seen success across a number of verticals including eCommerce, real estate, and telecommunications.
A lot of ML's recent growth in both the US and India is owing to the increase in data availability and the ability to process that data through virtually unlimited processing power in the cloud. Some of the US tech giants like Amazon, Facebook, and Google were quick to see the benefits of using ML to automate and optimize aspects of their business. It's one of the reasons those companies are so successful. They placed ML at the heart of their long-term business strategies. We now have entire industries across the world scrambling to catch up.
I would say that a few years ago, the gap between the tech giants and other companies in India was wider than it was in the US. This was likely due to the early concentration of ML research and talent in the US, and India was a few years behind the US in making a heavy investment into this space. But things are beginning to level out quickly and the growth of Bangalore as a global center for tech innovation shows that. The large tech giants still have an advantage over other companies, but today the field is more level between the non-tech giants in India and the US. Vidora can play a big role in levelling the playing field even more, and we want to help companies provide the same ML platforms to their employees that the tech giants have for theirs.
The ML industry faces a couple of major challenges, and how countries like the US and India deal with them has huge implications on the innovations we'll see in the future.
The first challenge is that ML is still new and relatively opaque to a lot of businesses, which makes it scary to adopt. There is still a skepticism around ML and most businesses, especially larger ones, are naturally risk-averse and therefore innovation-averse. In theory, this should be less of a hurdle as adoption grows. But I believe that this problem is being exacerbated by the second, and more dangerous, challenge that the ML industry faces – the problem of monopolization.
The monopolization of ML in the US could lead to innovation and knowledge in the field slowing significantly. Tech giants like Google, Amazon and Facebook are swallowing up just about any potential ML competition before it even has the chance to develop. If ML continues to be monopolized by a few private entities without regulations that encourage industry competition, ML adoption, and a wider range of research, we could see innovation falter in the US, especially if we fall prey to those companies' claims that they are "democratizing" the technology. I'm sceptical about the extent to which they have ever had people's interests at heart, given how cavalier they have been in their treatment of user privacy – and I think assuming that they want to open ML up would be a mistake.
India has been very forward-thinking in tackling this challenge. The Indian government's 'Digital India' program is investing a lot into a range of ML research, and I think the growth of India's ML industry is a testament to the program's success. In the US, the responsibility for progress has been left mostly up to the private sector. This gives businesses in the US' ML industry a crucial role in maintaining innovation and helping industries transition towards ML adoption. At Vidora, we see this as our most important mission.
Adopting ML is a massive undertaking. The multiple years and millions upon millions of dollars that companies like Facebook and Uber have poured into implementing ML is evidence of that. But when a business is ready for ML, they face some big questions. How can ML be implemented as efficiently and openly as possible? Those are some of the challenges we try to solve with Cortex.
As long as governments and ML companies around the world share the vision of making ML accessible to all, the barriers to entry will erode. Factors such as geographical distance, a concentration of ML-focused companies and so on will become less significant. Not only will more enterprises implement ML, but more will understand it, and the gap between the small number of businesses at the top and everyone else will narrow.
Today, India has the third largest number of AI or ML-focused businesses in the world, and that number is only going to increase. The US risks seeing its ML industry stagnate if government and businesses alike can't resist the hegemonization of the technology that we see today. The onus is on companies like Vidora to keep opening up access to ML, and if we're successful, there should be plenty of exciting developments for machine learning on the horizon.