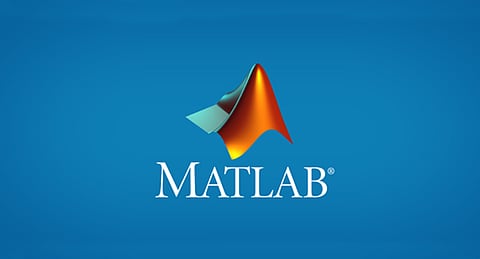
Getting data from test vehicles into the hands of end users is a common barrier for engineers who need data to formulate requirements for new products, troubleshoot field problems, and come up with new technologies. Connectivity technologies such as CAN and high-speed mobile communication removed this barrier in many situations. With more and more streaming data from vehicles, we are faced with a data science challenge. We need to ensure that the speed of data analysis is keeping pace with data intake and, equally important, provide the capability to zoom into and extract insight from stored data throughout the engineering community.
To address this new challenge, one often looks for those who have computer science skills, knowledge of statistics, and domain expertise relevant to their specific engineering problems. Such instinct is not wrong, but these types of candidates are rare. You may find success by focusing on domain expertise. Domain expertise is often overlooked, yet it is essential for making judgement calls during the development of an analytic model. It enables one to distinguish between correlation and causation, and between signal and noise. Domain knowledge is hard to teach. It requires on-the-job experience, mentorship, and time to develop. This type of expertise is often found in engineering and research departments that have built cultures around understanding the products they design and build. These teams are intimately familiar with the systems they work on. They use statistical methods and technical computing tools as part of their design processes, making the jump to the machine learning algorithms and big data tools of the data analytics world manageable. Instead of searching for elusive data scientists, companies can stay competitive by enabling their engineers to do data science with a flexible tool environment like MATLAB that enables engineers to become data scientists.
Engineers with domain knowledge need flexible and scalable environments to do data science. They need traditional analysis techniques such as statistics and optimization, data-specific techniques such as signal processing and image processing, as well as new capabilities such as machine learning algorithms. In particular, machine learning with big data leads to a host of different technologies that support the iterative process of building a data analytics algorithm. It's this beginning stage of the iterative process of building the algorithm that can set a business up for success. This iterative process involves trying several strategies like finding other sources of data and different machine learning approaches and feature transformations. Given the potentially unlimited number of combinations to try, it is crucial to iterate quickly. Domain experts are well suited to iterate quickly, as they can use their knowledge and intuition to avoid approaches that are unlikely to give strong results. The faster an engineer with domain knowledge can apply their knowledge with the tools that enable quick iterations, the faster the business can gain a competitive advantage.
According to Gartner, engineers with the domain expertise "can bridge the gap between mainstream self-service analytics by business users and the advanced analytics techniques of data scientists. They are now able to perform sophisticated analysis that would previously have required more expertise, enabling them to deliver advanced analytics without having the skills that characterize data scientists."
Let's look at a real-world example. Engineers at Baker Hughes used machine learning techniques to predict when pumps on their gas and oil extraction trucks would fail. They collected nearly a terabyte of data from these trucks, then used signal processing techniques to identify relevant frequency content. Domain knowledge was crucial here, as they needed to be aware of other systems on the truck that might show up in sensor readings, but that wasn't helpful at predicting pump failures. They applied machine learning techniques that can distinguish a healthy pump from an unhealthy one. The resulting system is projected to reduce overall costs by US$10 million. Throughout the process, their knowledge of the systems on the pumping trucks enabled them to dig into the data and iterate quickly.
Baker Hughes predictive maintenance workflow
Leveraging tools for processing big data and applying machine learning, engineers such as those at Baker Hughes are well-positioned to tackle problems that improve business outcomes. With their domain knowledge of these complex systems, engineers take these tools far beyond traditional uses for web and marketing applications.
How to start? Engineers with domain knowledge should look for tools that get them up and running quickly. They need to ensure that the selected tools can simultaneously lower the bar for machine learning for the domain experts and provide flexibility and extensibility for others. Finally, engineers need to integrate their data analytics work with their companies' systems, products, and services. This typically means deploying the analytics up to the servers maintained by IT, and down into embedded devices such as an ECU. I have personally worked with many teams using MATLAB to build engineering analytics applications and automatically convert their analytics model to run in embedded devices, which reduces development time by several months and eliminate bugs caused from rewriting the analytics programs.
Technologies that enable domain experts to apply machine learning and other data analytics techniques to their work are here to stay. They provide exciting opportunities for engineering teams to innovate—in both their design workflows and the products they create. Given the shortage of data scientists, engineers as domain experts have an opportunity to play a crucial role in filling this gap. Their knowledge of the business and the products it produces positions them well to find innovative ways to apply data analytics technologies.
Join our WhatsApp Channel to get the latest news, exclusives and videos on WhatsApp
_____________
Disclaimer: Analytics Insight does not provide financial advice or guidance on cryptocurrencies and stocks. Also note that the cryptocurrencies mentioned/listed on the website could potentially be scams, i.e. designed to induce you to invest financial resources that may be lost forever and not be recoverable once investments are made. This article is provided for informational purposes and does not constitute investment advice. You are responsible for conducting your own research (DYOR) before making any investments. Read more here.