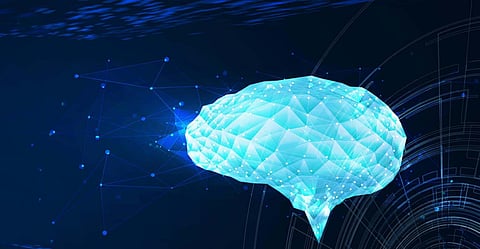
Artificial Intelligence (AI) and machine learning innovation have been growing quickly as of late, with conceivable outcomes developing in tandem with more noteworthy accessibility of data and headways in computing capability and storage solutions. Truth be told, if you look in the background, you can spot numerous instances of machine learning innovation as of now already in a wide range of industries ranging from consumer products and social media to financial services and manufacturing.
Machine learning can turn into a strong analytical tool for huge volumes of data. The blend of machine learning and edge computing can channel a large portion of the commotion gathered by IoT tools as well as gadgets and leave the significant information to be analyzed by the edge and cloud analytic engines.
The advances in Artificial Intelligence have enabled us to see self-driving vehicles, speech recognition, active web search, and facial and image recognition. Machine learning is the establishment of those frameworks. It is so unavoidable today that we most likely use it many times each day without knowing it.
Machine learning algorithms, particularly deep learning neural networks frequently produce models that improve the exactness of forecast. However, the accuracy comes to the detriment of higher computation and memory utilization. A deep learning algorithm, otherwise called a model, comprises of layers of computations where a large number of parameters are processed in each layer and went to the next, iteratively. The higher the dimensionality of the information (e.g., a high-resolution picture), the higher the computational need. GPU farms in the cloud are regularly used to meet these computational necessities.
Edge processing is a distributed computing worldview which brings computation and data stockpiling nearer to the area where it is required, to improve response times and spare bandwidth. In spite of the fact that edge computing addresses connectivity, latency, scalability and security challenges, the computational asset necessities for deep learning models at the edge gadgets are difficult to satisfy in smaller gadgets.
Most organizations today store their information in the cloud. This implies information needs to travel to a central data center, which is regularly found thousands of miles away, for model comparison before the concluding insight can be transferred back to the gadget of birthplace. This is a critical and even perilous issue in cases, for example, fall detection where time is of the essence.
The issue of latency is what is driving numerous organizations to move from the cloud to the edge today. "Insight on the edge," Edge AI" or "Edge machine learning" implies that, rather than being processed in algorithms situated in the cloud, data is processed locally in algorithms put away on a hardware gadget. This empowers real-time activities, however, it likewise serves to fundamentally reduce the power utilization and security vulnerability related with processing data in the cloud.
Before deciding the sort of hardware for edge gadgets, it is essential to build up key performance measurements for the induction. At a significant level, the key performance measurements for machine learning at the edge can be outlined as latency, throughput, energy consumption by the device, and accuracy. The latency alludes to the time it takes to gather one data point, throughput is the quantity of derivation calls every second, and accuracy is the confidence level of the expectation yield required by the utilization case.
Analysts have discovered that lessening the quantity of parameters in deep neural network models help decline the computational assets required for model inference. Some well known models which have utilized such systems with the least (or no) accuracy degradation are YOLO, MobileNets, Solid-State Drive (SSD), and SqueezeNet. Huge numbers of these pre-prepared models are accessible to download and use in open-source platforms, for example, TensorFlow or PyTorch.
Another age of purpose-built accelerators is rising as chip producers and startups work to accelerate and streamline the outstanding tasks at hand engaged with AI and machine learning projects, going from training to inferencing. Faster, less expensive, more power-proficient and scalable. These accelerators guarantee to support edge gadgets to another degree of performance. One of the manners in which they accomplish this is by mitigating edge gadgets' central processing units of the perplexing and overwhelming mathematical work associated with running deep learning models.
As a rule, numerous challenges remain to improve the performance of deep learning models. Yet, it is evident that the inference of the model is moving towards the edge gadgets. It is imperative to comprehend the business use case and key performance necessities of the model to accelerate execution at the asset constrained gadget. SAP Edge Services and Data Intelligence together give a start to finish tool for training machine learning models in the cloud and managing the life cycle and execution at the edge devices.
At the point when we take a look at history and where we are today, apparently, the advancement of edge machine learning is quick and relentless. As future advancements keep on unfurling, get ready for effect and make sure you're ready to take advantage of the opportunities this innovation brings.