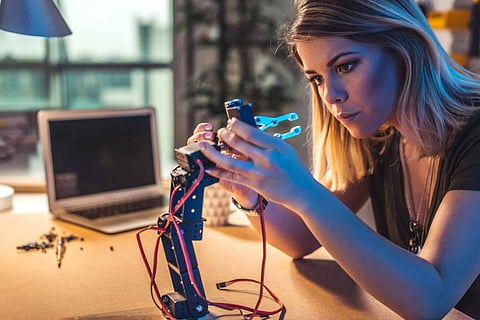
In the course of recent years, users have without doubt seen quantum jumps in the quality of a wide scope of ordinary innovations. Most clearly, the speech recognition functions on our cell phones work much better to anything they used to. When we utilize a voice direction to call our mates, we contact them now. Truth be told, we are progressively connecting with our PCs by simply conversing with them, regardless of whether it's Amazon's Alexa, Apple's Siri, Microsoft's Cortana, or the many voice-responsive features of Google. Chinese pursuit monster Baidu says clients have tripled their utilization of its speech interfaces in the previous year.
Machine interpretation and different types of language processing have additionally turned out to be unquestionably all the more persuading, with Google, Microsoft, Facebook, and Baidu disclosing new traps each month. Google Translate now renders spoken sentences in a single language into spoken sentences in another for 32 sets of languages, while offering text interpretations for 103 tongues, including Cebuano, Igbo, and Zulu. Google's Inbox application offers three instant answers for many incoming mails.
Because of machine learning, and specifically deep learning, we currently have robots and gadgets that have a truly decent visual comprehension of their surroundings. But, let's not overlook, sight is only one of the human senses. For algorithms that better copy human intelligence specialists are presently concentrating on datasets that draw from sensorimotor frameworks and tactile criticism. With this additional sense to draw on, future robots and AI devices will have much more noteworthy attention to their physical environment, opening up new use cases and potential outcomes.
The SenseNet project depends on deep reinforcement learning (RL), a part of machine learning that draws from both supervised and unsupervised learning strategies and depends on an arrangement of remunerations dependent on monitored interactions to discover better approaches to enhance results iteratively. Many trusts that RL offers a pathway to creating self-sufficient robots that could ace certain free practices with insignificant human interference. For instance, introductory assessments of deep RL strategies show that it is conceivable to utilize simulation to create adroit 3D manipulation abilities without having to physically make portrayals.
The SenseNet store on GitHub gives various assets past the 3D object dataset, including training models, classification tests, benchmarks, Python* code tests, and that's just the beginning. The dataset is made significantly progressively helpful through the addition of a simulator that gives scientists a chance to stack and control the items. All this is basically like assembling a layer upon the Bullet physics engine. Bullet is a generally utilized physics engine in recreations, motion pictures, and most as of late, robotics and machine learning research. It is a real-time physics engine that simulates delicate and inflexible bodies, crash detection, and gravity. A robotic hand is incorporated considered the MPL that takes into consideration a full scope of movement in the fingers and a touch sensor is inserted on the tip of the index finger that enables the hand to reproduce contact.
Autonomous vehicles are likely self-evident. Rather than building a pipeline with visual odometry intertwined with GPS/INS, object discovery, tracking, semantic division and so on, one can track sensor inputs directly to controlling wheel/breaking/accelerator utilizing trained information and a deep neural net. As of late, the renowned programmer George Hotz accomplished something like this all alone. Such frameworks so far are additional weak in light of the fact that it would take a colossal dataset to record every one of the large numbers of corner cases that can occur on real world streets. Yet at the same time, they make for some amazing and quite affordable demos.
To quicken the training and testing of numerous reinforcement learning algorithms, Intel's Reinforcement Learning Coach, a machine learning test system is incorporated. Working inside a Python domain, the Reinforcement Learning Coach let's engineers model the connection between an agent and nature, joining different building blocks and giving visualization tools to powerfully show training and test results, the Reinforcement Learning Coach makes the training procedure progressively proficient, and additionally supporting testing of the agent on various situations. The advanced visualization devices, in light of information gathered amid the testing groupings, can be promptly accessed through the Coach dashboard and used to investigate and optimise the agent being tested.
Deep learning, in that vision, could change any industry. According to Jeff Dean, who drives the Google Brain venture emphasizes that there are essential changes that will happen since computer vision truly works. Does that mean it's a great opportunity to support for "the singularity", the hypothesized minute when hyper-savvy machines begin enhancing themselves without human contribution, setting off a runaway cycle that leaves humble people ever further in the dust, with unnerving consequences? Not at this time. Neural nets are great at perceiving designs, now and then in the same class as or superior to anything we are at it. However, they can't reason.
Join our WhatsApp Channel to get the latest news, exclusives and videos on WhatsApp
_____________
Disclaimer: Analytics Insight does not provide financial advice or guidance. Also note that the cryptocurrencies mentioned/listed on the website could potentially be scams, i.e. designed to induce you to invest financial resources that may be lost forever and not be recoverable once investments are made. You are responsible for conducting your own research (DYOR) before making any investments. Read more here.