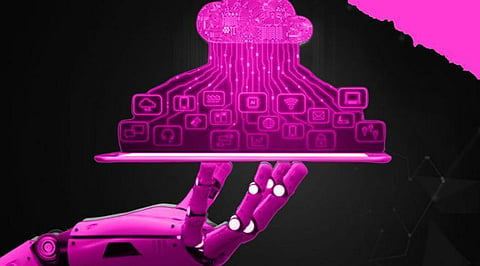
The word Cloud technologies evoke technologically progressive vibes for, the convenience and cluster of applications it has to offer with efficiency. The cherry on the cake is the money businesses can save with Cloud-hosted servers and data storage facilities. Taking this game further, in recent years artificial intelligence applications have migrated to the cloud to bring down exorbitant prices. The low CAPEX costs are the reason why businesses went for the cloud to reduce overall expenditure. Having sturdy processors and data storage facilities was costlier with frequent maintenance and operation costs taken into consideration. Also, one cannot have unlimited access to storage facilities, as it depends on the allocated budget. Bring in AI, a complicated and process-intensive technology, it has too many needs to cater to for it to serve its purpose. Cloud technologies are not comparable and are gradually evolving to scale up to the need but it is possible only if the needs are specific.
Ever since Cloud technologies came into prominence, AI/ML stakeholders have largely been under the influence of the notion that on-premise infrastructure cannot stand the computation-heavy AI/ML operations. But with evolving AI/ML technologies and the improved hardware options in the field, running them on-premise is proving to be more conducive to achieving positive outcomes. "We still have a ton of customers who want to go on a cloud migration, but we're definitely now seeing — at least in the past year or so — a lot more customers who want to repatriate workloads back onto on-premise because of cost," said Thomas Robinson, vice president of strategic partnerships and corporate development at MLOps platform company Domino Data Lab. It is not only data storage that influences cost efficiency but the way it is stored and the number of iterations a model has to go through at every stage of its development cycle escalates the cost. Given the fact that the models process terabytes and petabytes of data at every stage, the to and fro transfer of which from the cloud to the model development center or between the clouds makes no sense, even if it doesn't involve high transaction costs. For this very reason, AI developers have started developing on-premise data centers, and in certain cases started depending on managed service providers and co-location providers (colos). Eventually, AI/ML cloud symphony is emerging as an area demanding for the adoption of a strategy requiring switching between cloud for specific tasks and on-premise for more liberal experimentation and streamlined workload management.