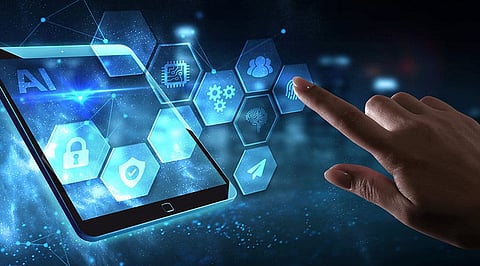
When Artificial Intelligence is changing the way people live, utilizing the multimodal approach can see and perceive outer situations.
Billions of petabytes of data move through AI devices consistently. Nonetheless, at this moment, the vast majority of these AI devices are working independently of one another. However, as the volume of data moving through these gadgets increments in the coming years, technology organizations and implementers should sort out a path for every one of them to learn, think, and work together to genuinely harness the potential that AI can deliver.
An interesting report by ABI Research envisions that while the total installed base of AI devices will increase from 2.69 billion in 2019 to 4.47 billion out of 2024, nearly not many will be interoperable temporarily. Instead of consolidating the gigabytes to petabytes of data coursing through them into a single AI model or framework, they'll work independently and heterogeneously to sort out the data they're fed.
Derived from the Latin words 'multus' which means numerous and 'modalis' which means mode, multimodality, with regards to human perception, is basically that the capacity to use various multiple sensory modalities to encode and decode external surroundings. At the point when joined, they make a united, singular perspective on the world.
Multimodal perception rises above the universe of innovation. When applied to Artificial Intelligence explicitly, joining different AI information sources into one model is known as Multimodal Learning.
In contrast to conventional unimodal learning frameworks, multimodal systems can convey correlative data about one another, which will possibly become evident when they are both included in the learning cycle. In this manner, learning-based techniques that consolidate signals from various modalities are fit for creating more robust inference, or even new insights, which would be inconceivable in a unimodal framework.
Multimodal learning presents two essential advantages:
Firstly, numerous sensors observing the same data can make more strong forecasts, since recognizing changes in it may be possible when the two modalities are available. Secondly, the combination of numerous sensors can encourage the capture of complementary information or trends that may not be caught by individual modalities.
With its visual dialogue framework, Facebook would have all the earmarks to be pursuing a digital assistant that copies human partners by reacting to pictures, messages, and messages about pictures as normally as an individual may. For instance, given the brief "I need to get a few seats — show me earthy colored ones and enlighten me regarding the materials," the assistant may reply with a picture of earthy colored seats and the content "How would you like these? They have a strong earthy colored tone with a foam fitting."
In the automotive space, multimodal learning is being presented with Advanced Driver Assistance Systems (ADAS), In-Vehicle Human Machine Interface (HMI) associates, and Driver Monitoring Systems (DMS) for real-time inferencing and forecast.
Robotics vendors are fusing multimodal systems into robotics HMIs and movement automation to widen customer appeal and give more noteworthy collaboration among laborers and robots in the industrial space.
Similarly, as we have set up that human perception is emotional, the equivalent can be said for machines. In a time when AI is changing the way people live and work-AI, utilizing the multimodal approach, can see and perceive outer situations. Simultaneously, this methodology imitates the human way to deal with perception, including imperfections as well.
All the more explicitly, the advantage is that machines can replicate this human way to deal with the perception of external scenarios. However, certain AI technology can see data up to 150x faster than a human (in corresponding with a human guard).
With this new turn of events, we are drawing nearer to mirroring human insight, and well, the possibilities are endless.
There's only one issue. Multimodal systems get on inclinations in datasets. The variety of questions and ideas engaged with assignments like VQA, as well as the absence of great data, regularly keep models from figuring out how to "reason," driving them to make educated guesses by depending on dataset statistics.
The solution will probably include bigger, more comprehensive training datasets. A paper published by engineers at École Normale Supérieure in Paris, Inria Paris, and the Czech Institute of Informatics, Robotics, and Cybernetics proposes a VQA dataset made from millions of narrated videos. Comprising naturally produced sets of questions and answers from transcribed recordings, the dataset dispenses with the requirement for manual annotation while empowering strong performance on well-known benchmarks, as per the analysts.
Join our WhatsApp Channel to get the latest news, exclusives and videos on WhatsApp
_____________
Disclaimer: Analytics Insight does not provide financial advice or guidance on cryptocurrencies and stocks. Also note that the cryptocurrencies mentioned/listed on the website could potentially be scams, i.e. designed to induce you to invest financial resources that may be lost forever and not be recoverable once investments are made. This article is provided for informational purposes and does not constitute investment advice. You are responsible for conducting your own research (DYOR) before making any investments. Read more here.