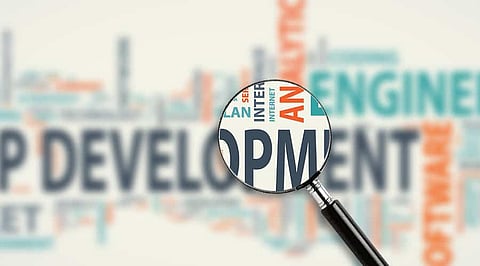
The fast growth of digital texts necessitates the usage of text analytics. It raises the issue of devising a more intelligent method of reading and comprehending materials and eventually obtaining information from them. Read on to learn more about text analytics along with its techniques and applications.
The act of converting unstructured text documents into useful, organized data is known as text analytics. Text analysis works by dissecting words and phrases into their constituent parts and assessing the role and significance of each portion using complicated software rules and machine learning techniques.
Text analytics used to be limited to simple tasks like determining word frequencies. AI technologies such as natural language understanding (NLU) and MI, as well as approaches such as deep learning, have significantly increased the efficacy of text analytics in recent years.
1. Clustering
One of the most important text mining approaches is clustering. Its goal is to find inherent patterns in textual data and arrange them into pertinent subgroups or 'clusters' for further study. The creation of meaningful clusters from unmarked textual material without any prior knowledge is a key problem in the clustering process. Cluster analysis is a common text mining method that aids in data distribution or serves as a pre-processing phase for other text mining techniques that operate on clusters that have been discovered.
2. Summarization
The technique of automatically creating a compressed version of a given text that contains useful information for the end-user is known as text summarization. This text mining approach tries to search numerous text sources for abstracts of texts that provide a significant amount of information in a compact style while maintaining the original documents' general sense and intent. Text summarization combines and incorporates numerous text classification approaches such as decision trees, neural networks, logistic regression, and swarm optimization.
3. Information Extraction
This is the most widely used text mining method. The process of extracting relevant information from large amounts of textual material is referred to as information exchange. The goal of this text mining approach is to extract entities, characteristics, and their connections from semi-structured or unstructured texts. Whatever data is collected is then saved in a database for easy access and retrieval in the long term. Precision and recall methods are used to examine and enhance the effectiveness and relevance of the outputs.
4. Categorization
This is a type of "supervised" learning in which regular language texts are allocated to a pre-set set of subjects based on their content using text mining techniques. As a result, categorization, or Natural Language Processing (NLP), is the act of gathering textual data and processing and analyzing them to find the appropriate themes or indexes for each one.
1. Fraud Detection
Text analytics, along with text mining techniques, presents a huge potential for domains that collect the entirety of their data in text format. This is an opportunity that insurance and finance businesses are seizing. These businesses are now capable of processing claims quickly as well as preventing and detecting fraud by integrating the results of text analysis with pertinent structured data.
2. Social Media Analysis
Many text mining methods have been developed specifically for assessing the performance of social media sites. These aid with the tracking and interpretation of online content created by the news, blogs, emails, and other sources. Text mining technologies can also quickly evaluate the number of posts, likes, and connections your brand has on social media, helping you to better understand how people are reacting to your company and online content. The research will help you figure out "what's popular and what isn't" for your target market.
3. Customer Care Service
In the realm of customer service, text mining applications, notably natural language processing (NLP), are becoming increasingly important. Companies are adopting text analytics tools to improve their entire customer experience by gaining access to textual data from a variety of sources, including surveys, user feedback, and user conversations, among others. Text analysis seeks to minimize the company's reaction time and assist in quickly and efficiently resolving client complaints.
4. Knowledge Management
Managing a large volume of text data has become a difficulty in several areas, such as healthcare. It would certainly fly to the moon if you began designing systems and kept all of the papers relevant to healthcare on a single upwardly scalable rack. The amount of data collected per hour is enormous. All of this information must be kept in such a way that it may be accessed whenever it is needed. It's possible that an epidemic could break out, and hospitals will need to work together to analyze all of their data in order to locate the source or the first affected individual.
5. Risk Management
The absence of appropriate or inadequate risk analysis is one of the leading reasons for company failure. Implementing and adopting risk management tools based on text mining technologies, like SAS Text Miner, may assist organizations in staying current with industry trends and enhancing their ability to mitigate possible hazards. Because text mining tools and technologies can aggregate relevant data from hundreds of text data sources and build linkages between the retrieved insights, they enable companies to access the appropriate information at the right time, therefore improving the risk management framework.
During the projected period, the text analytics market is expected to expand by USD 8.77 billion, representing a CAGR of over 20%. The text analytics market has been unaffected by the worldwide shutdown since most IT activities are conducted through a 'working from home' framework. As a result, there is a continual need for text analytics in its application business all over the world.
During the Coronavirus epidemic, text analytics has shown to be useful in the healthcare industry for examining information on the epidemic. Text analytics is a type of software that aids in the creation of organized text data from unstructured text, as well as the discovery of trends, insights, and trends.