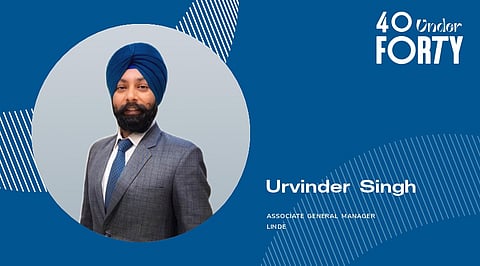
Urvinder Singh has been listed in “40 under 40 Data Scientists” - 2024, as a winner of the "Young Turks in AI and Analytics-2023" and the “AI Changemaker Impact Award” by 3AI, as well as the recipient of the “Emerging Data Analytics Leader of the Year Award.
Urvinder Singh is currently serving as the associate general manager at Linde. In this pivotal role, he is responsible for steering AI and ML projects to success, leveraging his extensive experience and expertise. His versatility and ability to drive data-driven innovation have been honed through his tenure at global giants like Colgate Palmolive and Ericsson.
Urvinder overlooks the data science operations for the Asia Pacific region. This breadth of experience, coupled with his exposure to diverse industries, including Telecom, FMCG/CPG, Oil & Gas, and Manufacturing, has enriched his skills and perspective. As a respected leader in the field of data science, he is passionate about translating cutting-edge technologies into tangible business value. His track record of success in delivering transformative solutions to address critical industry challenges is a testament to his ability to drive data-driven innovation in complex, dynamic environments.
Revolutionizing Retail with Smart Shelf Recommendation Engine
Urvinder had the opportunity to lead some truly transformative projects, and the 'Smart Shelf: Recommendation Engine for Modern Trade Chains' certainly stands out.
At the heart of this initiative was the need to empower Key Account Managers and unlock the true potential of our retail partnerships. The traditional, intuition-based approach to product listing and assortment simply wasn't cutting it anymore. The team needed a data-driven solution that could deliver strategic insights and uncover cross-sell and upsell opportunities.
The innovation achieved by the team with the recommendation engine truly lies in its integrative approach. By seamlessly blending collaborative and content-based strategies within a machine-learning framework, a robust platform was created that could not only suggest the right products for the right stores but also provide invaluable customer segmentation insights.
The key to their success was the way diverse data sources were leveraged, from sales and geographic data to psychographic and behavioral information. By aggregating and harmonizing this data, a comprehensive understanding of the customer base and their evolving preferences was built. This, in turn, allowed for the development of highly personalized and impactful recommendations.
But the innovation doesn't stop there. Advanced clustering algorithms and RFM (Recency, Frequency, Monetary) analysis were also incorporated to optimize shelf space and delist underperforming products. This not only boosted sales of premium items by an impressive margin but also significantly increased the overall share of business in the Modern Trade segment.
What truly sets this project apart, though, is the transformation it has brought to the mindset of the sales team. Empowered with data-driven insights, a shift from intuition-based decision-making to a more strategic, collaborative approach has been enabled. This transition has not only benefited the bottom line but has also strengthened partnerships with retailers, allowing for the delivery of a more tailored and satisfying customer experience.Urvinder says, “as we look to the future, I'm excited to see how we can further refine and scale this innovative recommendation engine across new markets and verticals. The potential is truly limitless, and I'm confident that the lessons we've learned can serve as a blueprint for data-driven success in the ever-evolving world of retail."
Personalizing the Future with Advanced Analytics
One of the industry's key demands is the necessity for personalization and optimization in retail operations. Customers now anticipate tailored experiences and recommendations, and the recommendation engine meets this requirement by harnessing advanced machine learning algorithms to scrutinize various data sources, encompassing sales, geographic, demographic, psychographic, and behavioral data. By comprehending customer behavior and preferences at a detailed level, the solution facilitates Key Account Managers (KAMs) in providing personalized recommendations for cross-selling and upselling opportunities, thus enriching the overall customer experience.
Furthermore, the retail sector is observing a transition towards optimization and efficiency in inventory management and sales strategies. The recommendation engine addresses this demand by integrating advanced clustering algorithms for customer segmentation, enabling businesses to profile clusters of stores exhibiting similar behavior. This not only optimizes product recommendations but also streamlines inventory management by identifying and removing underperforming products from store shelves.
Moreover, in an era marked by rapid technological advancements, there is an increasing requirement for scalability and real-time integration in analytics solutions. The recommendation engine meets this demand by being deployed on the Google Cloud Platform, providing scalability and real-time integration with the KAMs' purchase order system. This ensures that recommended products are dynamically updated based on real-time data, enhancing agility and responsiveness to market changes.
Data-Driven Decision-Making
Their solution stood as a transformative innovation within the retail sector, profoundly enhancing operational efficiency and customer satisfaction. By harnessing advanced machine learning algorithms and a variety of data sources encompassing sales, geography, demographics, psychographics, and behavioral patterns, the engine empowered Key Account Managers (KAMs) to make informed, data-driven decisions regarding product assortment. This optimization of shelf space utilization and inventory management resulted in notable enhancements in sales performance and revenue generation.
Furthermore, the engine effectively tackled the cold start problem by leveraging data at every geocode level, eliminating arbitrary listing, and facilitating more strategic recommendations. This, in turn, contributed to a significant increase in the market share of their products by over 6%, a 56% upsurge in premiumization, and an impressive 80% adherence to recommended products. The integration of real-time updates and seamless alignment with the purchase order system ensured agility and responsiveness to market fluctuations.
Overall, their recommendation engine redefined retail analytics, establishing new benchmarks for data-driven decision-making and empowering businesses to flourish in the dynamic retail landscape. Not only did this solution demonstrate a notable increase in ROI, but it also underscored the transformative potential of data science within the retail sector, sparking a wave of digital transformation and a shift in mindset. This, in turn, paved the way for the adoption of analytics use cases incorporating technologies like GenAI for e-commerce, media spending optimization, and beyond.
Navigating Challenges in Retail Analytics
While the recommendation engine offers significant advantages, it also presents its own set of potential challenges. firstly, the integration of data from diverse sources with varying granularities requires meticulous preprocessing and data-cleaning efforts. secondly, ensuring the sustained accuracy and relevance of recommendations demands continuous monitoring and updates, particularly in light of evolving customer behaviors post-COVID. Additionally, concerns regarding data privacy and security, especially concerning sensitive customer information, require careful consideration. moreover, maintaining the freshness and accuracy of psychographic and behavioral data post-pandemic is crucial, given the significant shifts in demographics and purchasing patterns. addressing these challenges is essential to maintaining the continued effectiveness and integrity of the recommendation engine in providing actionable insights and driving business success.
Building the Future of Retail with Urvinder Singh's Vision
From a development standpoint, the entire solution was internally constructed, with a significant emphasis placed on change management. This initiative marked a paradigm shift in product placement and decision-making within the modern trade sector. Close collaboration with key stakeholders such as operations and sales teams was essential to ensure alignment of objectives and smooth integration of the solution into existing processes. Cultivating a culture of data-driven decision-making across the organization was deemed crucial, prompting extensive training and awareness programs to empower teams in effectively utilizing insights from the Recommendation Engine.
Furthermore, partnerships were forged with external data providers and technology vendors to access a wide array of data sources, enhancing the solution's robustness and scalability. These collaborative efforts and the focus on change management played a pivotal role in driving innovation, surmounting challenges, and achieving success in optimizing retail operations and enhancing customer experiences.
Join our WhatsApp Channel to get the latest news, exclusives and videos on WhatsApp
_____________
Disclaimer: Analytics Insight does not provide financial advice or guidance on cryptocurrencies and stocks. Also note that the cryptocurrencies mentioned/listed on the website could potentially be scams, i.e. designed to induce you to invest financial resources that may be lost forever and not be recoverable once investments are made. This article is provided for informational purposes and does not constitute investment advice. You are responsible for conducting your own research (DYOR) before making any investments. Read more here.