Building Artificial Intelligence That Can Build Artificial Intelligence
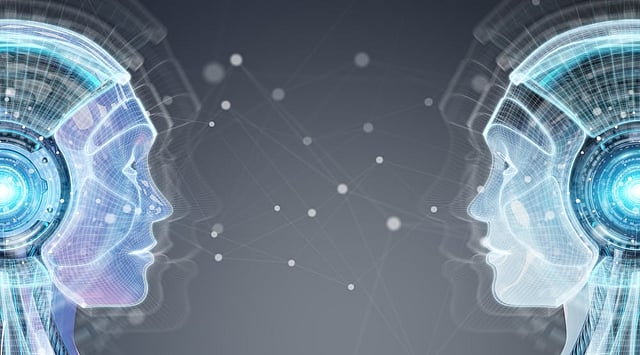
In May 2017, researchers at Google Brain declared the formation of AutoML, an artificial intelligence (AI) that is equipped for producing its own AIs. All the more as of late, they chose to give AutoML its greatest challenge to date, and the AI that can construct AI made a "child" that beat the majority of its human-made partners.
With it, Google may soon figure out how to make AI innovation that can incompletely remove the people from building the AI frameworks that many accept are the future of the innovation business. The venture is a piece of a lot bigger exertion to bring the best in class AI techniques to a more extensive collection of organizations and software developers.
The Google analysts automated the structure of ML models utilizing a methodology called reinforcement learning. AutoML goes about as a controller neural network that builds up a child AI network for a particular activity. For this specific child AI, which the specialists called NASNet, the undertaking was perceiving objects, individuals, vehicles, traffic lights, totes, rucksacks, and so forth, in a video real-time.
AutoML would assess NASNet's performance and utilize that data to improve its child AI, rehashing the procedure a large number of times. At the point when tried on the ImageNet picture classification and COCO object detection data sets, which the Google scientists call "two of the most regarded large-scale scholastic data sets in computer vision," NASNet outflanked all other computer vision frameworks.
As per the analysts, NASNet was 82.7% exact at anticipating pictures on ImageNet's validation set. This is 1.2% superior to any recently published outcomes, and the framework is additionally 4% more productive, with a 43.1% mean Average Precision (mAP). Furthermore, a less computationally demanding adaptation of NASNet beat the best comparatively sized models for mobile platforms by 3.%.
The tech business is promising everything from smartphone applications that can perceive appearances to vehicles that can drive without anyone else. In any case, by certain estimates, just 10,000 individuals worldwide have the education, background and ability expected to fabricate the complex and at times secretive numerical algorithms that will drive this new type of artificial intelligence.
The world's biggest tech organizations, including Google, Facebook and Microsoft, some of the time pay a large number of dollars a year to AI specialists, successfully cornering the market for this elusive ability. The shortage isn't leaving at any point in the near future, since acing these abilities takes long years of work.
The business isn't happy to pause. Organizations are building up a wide range of devices that will make it simpler for any task to construct its very own AI software, including things like image and speech recognition services and online chatbots.
This isn't benevolence. Analysts like Mr. Dean believe that if more individuals and organizations are taking a shot at artificial intelligence, it will drive their very own exploration. In the meantime, organizations like Google, Amazon and Microsoft see genuine money in this field. Every one of them is selling cloud computing services that can support different organizations and developers to build AI. This is no doubt what Google has as a main priority for AutoML, as the organization keeps on hailing the venture's progress. Google's CEO, Sundar Pichai, gloated about AutoML a month ago while revealing another Android cell phone.
Inevitably, the Google project will help organizations build frameworks with artificial intelligence regardless of whether they don't have the broad aptitude, Mr. Dean said. Today, he assessed, close to a couple of thousand organizations have the correct ability for building AI, yet a lot more have the fundamental data.
Google is putting efforts vigorously in cloud computing services, services that help different organizations construct and run software which it hopes to be one of its essential economic motors in the years to come. Furthermore, in the wake of gobbling up such an enormous portion of the world's top AI researchers, it has methods for kicking off this engine. Neural systems are quickly quickening the advancement of AI. As opposed to building an image recognition service or a language interpretation application by hand, one line of code at any given moment, developers can significantly more rapidly assemble an algorithm that learns tasks all alone.
By breaking down the sounds in an immense gathering of old technical support calls, for example, an ML algorithm can figure out how to perceive verbally expressed words. ML is the thing that gives numerous AI frameworks their capacity to perform explicit tasks. Despite the fact that the idea of driving it is genuinely simple. An algorithm learns by being fed a huge amount of data, the procedure requires a gigantic amount of time and efforts. Via automating the way toward making exact, effective AI frameworks, an AI that can build AI takes on the brunt of that work. At last, that implies AutoML could open up the field of ML and AI to non-experts.