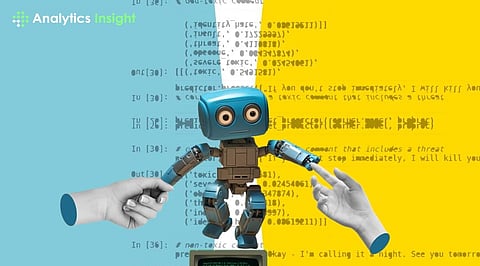
Dive into the cutting-edge realm of artificial intelligence as we explore potent strategies for training AI models with web data. Uncover the keys to harnessing the vast potential of online information, propelling your AI initiatives into the future of innovation and efficiency. In this article, we will discuss some strategies for training AI models from web data.
Prioritizing high-quality data over sheer quantity is crucial for the success of AI models. Curating datasets meticulously ensures the reliability and relevance of the information fed into the models. Web scraping facilitates the extraction of high-quality data from diverse internet sources, offering a rich foundation for training AI models.
Web scraping stands as a formidable process for extracting data from websites, enabling the collection of diverse and real-time information. Automation through web scraping tools streamlines the data collection process, ensuring the constant updating of source material.
Data augmentation involves generating new data from existing datasets, a strategy that enhances dataset size and consequently improves AI model accuracy. Techniques like image rotation, flipping, and cropping contribute to the generation of new data points, enriching the training dataset.
The success of AI models hinges on selecting the appropriate machine learning model architecture tailored to the task at hand. A plethora of model types exist, and the choice depends on the nature of the data and the problem to be solved.
Model optimization is a critical step involving fine-tuning to improve accuracy. Techniques such as hyperparameter tuning and regularization play a key role in this process. Striking the right balance ensures that the model generalizes well to new, unseen data.
Rigorous testing and evaluation are pivotal in the training process. The model must be assessed on a separate dataset to validate its accuracy and reliability. This step guarantees that the model performs robustly in diverse scenarios.
Once the model has been trained, tested, and optimized, it can be deployed to production for real-world applications. Continuous monitoring is essential post-deployment to ensure sustained performance, with adjustments made as necessary.
By meticulously following these strategies, businesses can develop accurate and reliable AI models capable of addressing a myriad of challenges. The combination of web scraping, data augmentation, thoughtful model selection, optimization, and rigorous testing forms a comprehensive approach to harnessing the power of web data for AI model training.
Join our WhatsApp Channel to get the latest news, exclusives and videos on WhatsApp
_____________
Disclaimer: Analytics Insight does not provide financial advice or guidance on cryptocurrencies and stocks. Also note that the cryptocurrencies mentioned/listed on the website could potentially be scams, i.e. designed to induce you to invest financial resources that may be lost forever and not be recoverable once investments are made. This article is provided for informational purposes and does not constitute investment advice. You are responsible for conducting your own research (DYOR) before making any investments. Read more here.